AutoFeedback: Scaling Human Feedback with Custom Evaluation Models
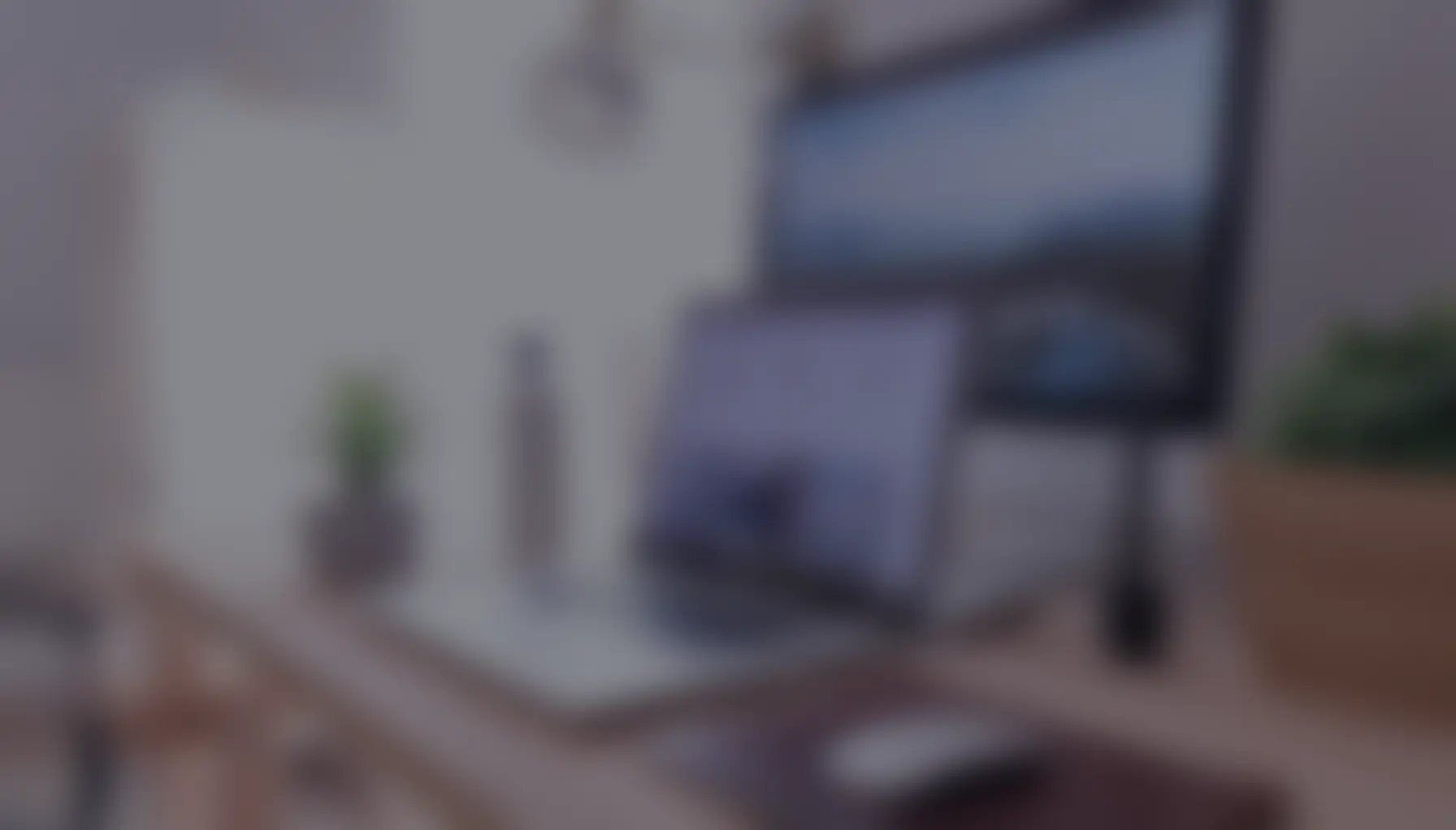
OVERVIEW
EXPERIENCE | In Person |
---|---|
TYPE | Breakout |
TRACK | Data Science and Machine Learning |
INDUSTRY | Enterprise Technology |
TECHNOLOGIES | AI/Machine Learning, GenAI/LLMs |
SKILL LEVEL | Intermediate |
DURATION | 40 min |
DOWNLOAD SESSION SLIDES |
Human feedback plays a crucial role in evaluating the output of LLM applications. However, relying solely on human review can be time-consuming and costly. To address this, we have developed an AutoFeedback system combining human and model-based evaluation strengths. We will discuss how our custom evaluation models, built using in-context learning and fine-tuning techniques, can significantly improve the efficiency and accuracy of LLM evaluation. By training these models with human feedback data, we have achieved a 44% reduction in absolute error on a 7-point grading task. Additionally, our evaluation models are capable of generating explanations for their grades, enhancing transparency and interpretability. Our synthetic bootstrapping procedure allows us to fine-tune models with as few as 25-50 human-labeled examples. The model-generated feedback approaches the accuracy of models trained on larger datasets while reducing costs by 10x+ compared to human annotations.
SESSION SPEAKERS
Arjun Bansal
/CEO & Co-founder
Log10