AutoML Time Series Forecasting for Infrastructure Resources
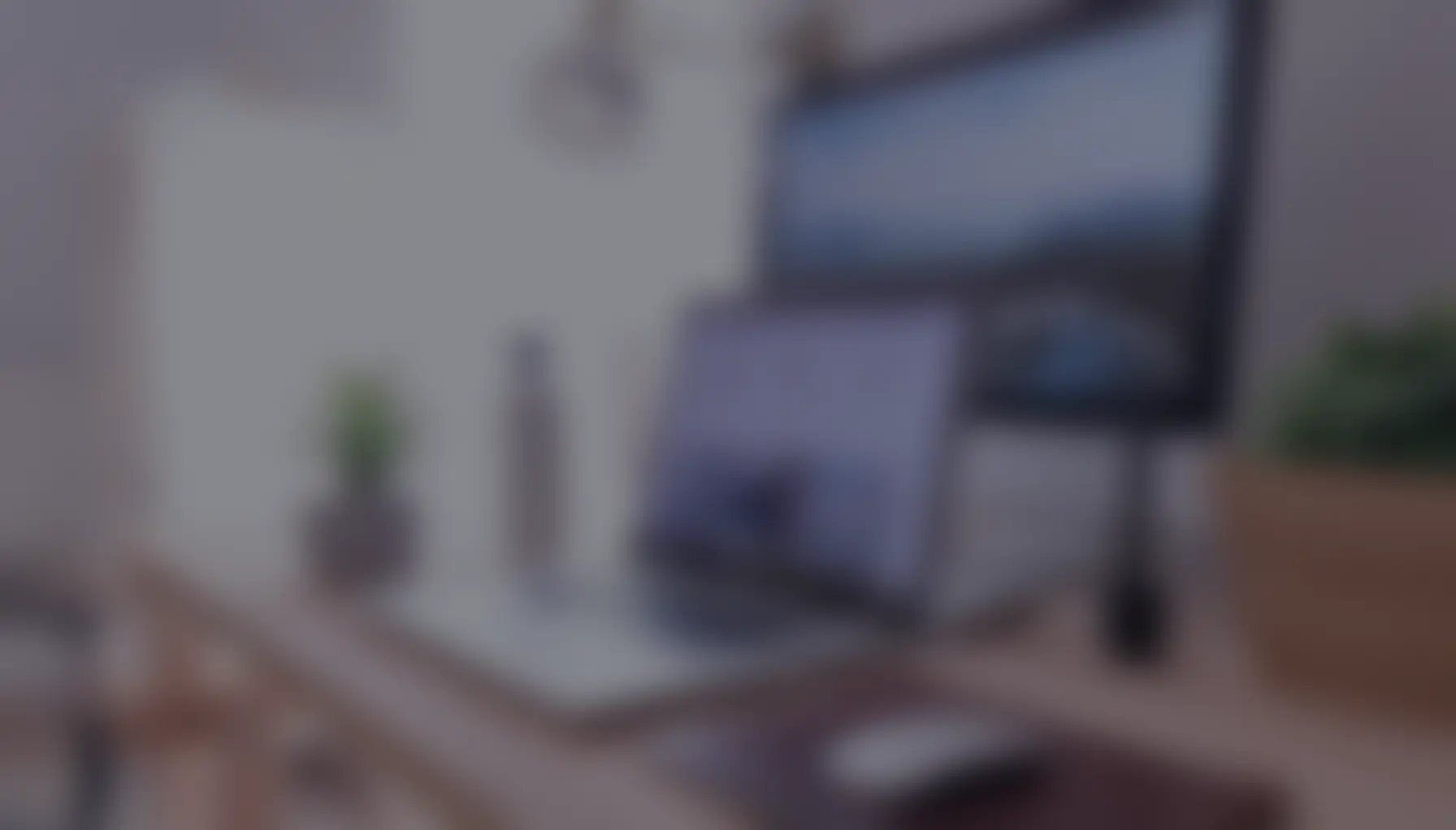
OVERVIEW
EXPERIENCE | In Person |
---|---|
TYPE | Breakout |
TRACK | Data Science and Machine Learning |
INDUSTRY | Enterprise Technology |
TECHNOLOGIES | AI/Machine Learning, Apache Spark, MLFlow |
SKILL LEVEL | Intermediate |
DURATION | 40 min |
DOWNLOAD SESSION SLIDES |
This session presents a framework for applying AutoML time series forecasting powered by Spark to plan the IT infrastructure resources, which include computation and storage resources such as CPU, memory, disk sizes, etc. The forecasting focuses on time series metrics of infrastructure resources. One significant innovation is that the Spark-based AutoML and big data distributed computation is used to automate and linearly scale up the fitting and forecasting operations of millions of time series models in parallel. The forecasting framework can address long-term planning to achieve and maintain an adequate supply equilibrium and to guide the daily dynamic provisioning of computing and storage resources. Two empirical applications are presented. In the applications, thousands of time series models are automatically built and used to forecast and plan the short- and long-term resource demand.
SESSION SPEAKERS
Heping Liu
/Senior Principal Engineer
Workday Inc.