Delivering Domain Specific LLMs with GPU Serving: Case of IFC MALENA
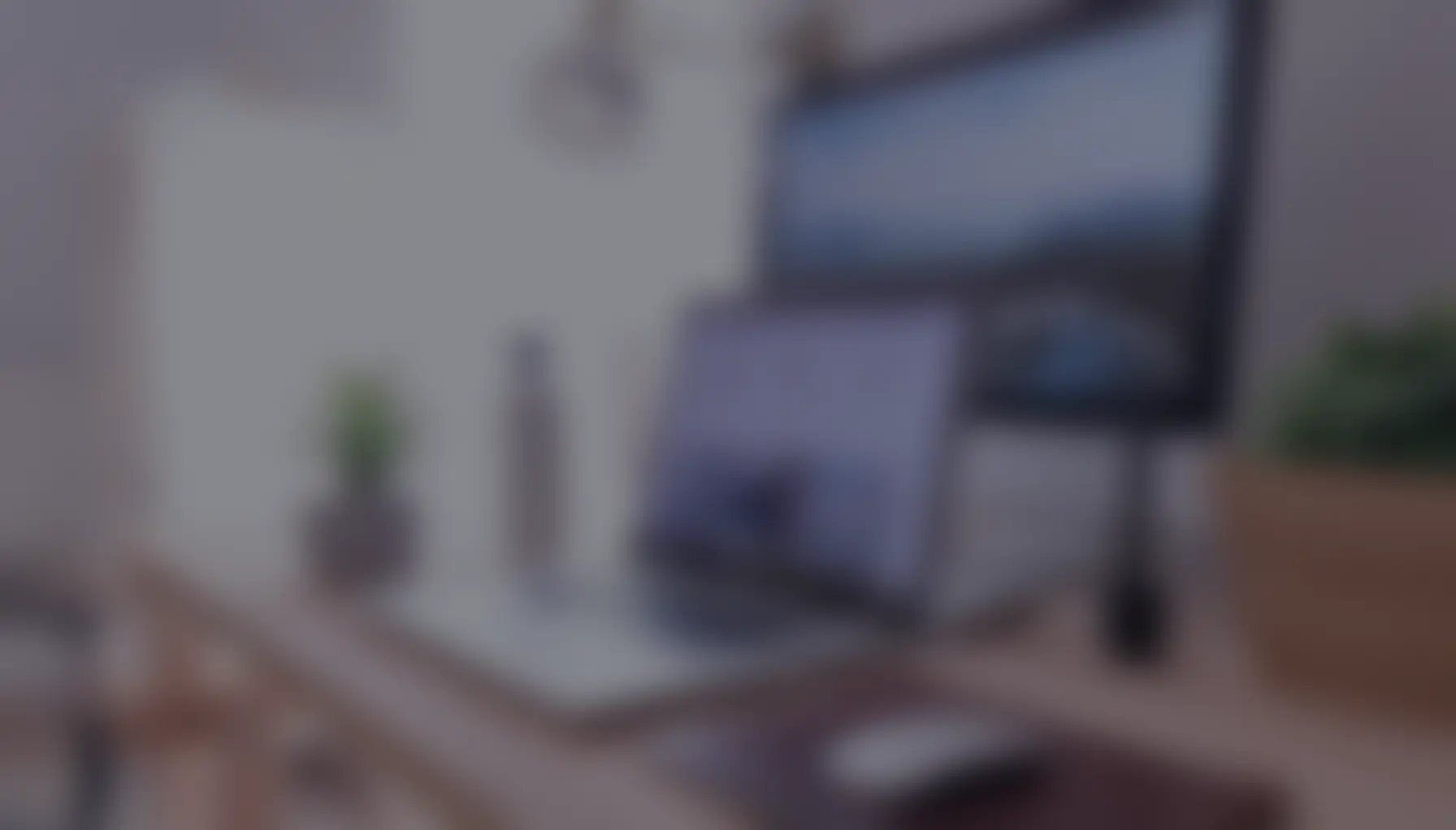
OVERVIEW
EXPERIENCE | In Person |
---|---|
TYPE | Breakout |
TRACK | Generative AI |
INDUSTRY | Public Sector, Financial Services |
TECHNOLOGIES | AI/Machine Learning, GenAI/LLMs, MLFlow |
SKILL LEVEL | Intermediate |
DURATION | 40 min |
DOWNLOAD SESSION SLIDES |
The International Finance Corporation (IFC), a member of the Word Bank Group, is harnessing the power of data and AI to address the development challenges of poverty and climate change. IFC successfully scaled its AI-powered MALENA platform using Lakehouse to accelerate the development of custom large language models. As AI model sizes grow and users expect inference results in real-time, secured and low-latency model serving becomes critical. In this session, the IFC team will share how use of Databricks' model serving enhanced real-time inferencing when serving internal IFC users and external B2B REST API users. The team will share their LLM Ops journey and performance metrics for Azure Functions versus CPU model serving, particularly for serving fine-tuned models built on Google BERT. The team will show how and why optimized GPU model serving may offer an optimal solution for fine-tuned models trained on foundation models such as Llama 2 or Mistral.
SESSION SPEAKERS
Blaise Sandwidi
/Lead Data Scientist, ESG Officer, PhD
International Finance Corporation (IFC)
Jonathan Lorentz
/Data Scientist
International Finance Corporation