How To (Maybe) Make Money Betting on NBA Using Rocket Science
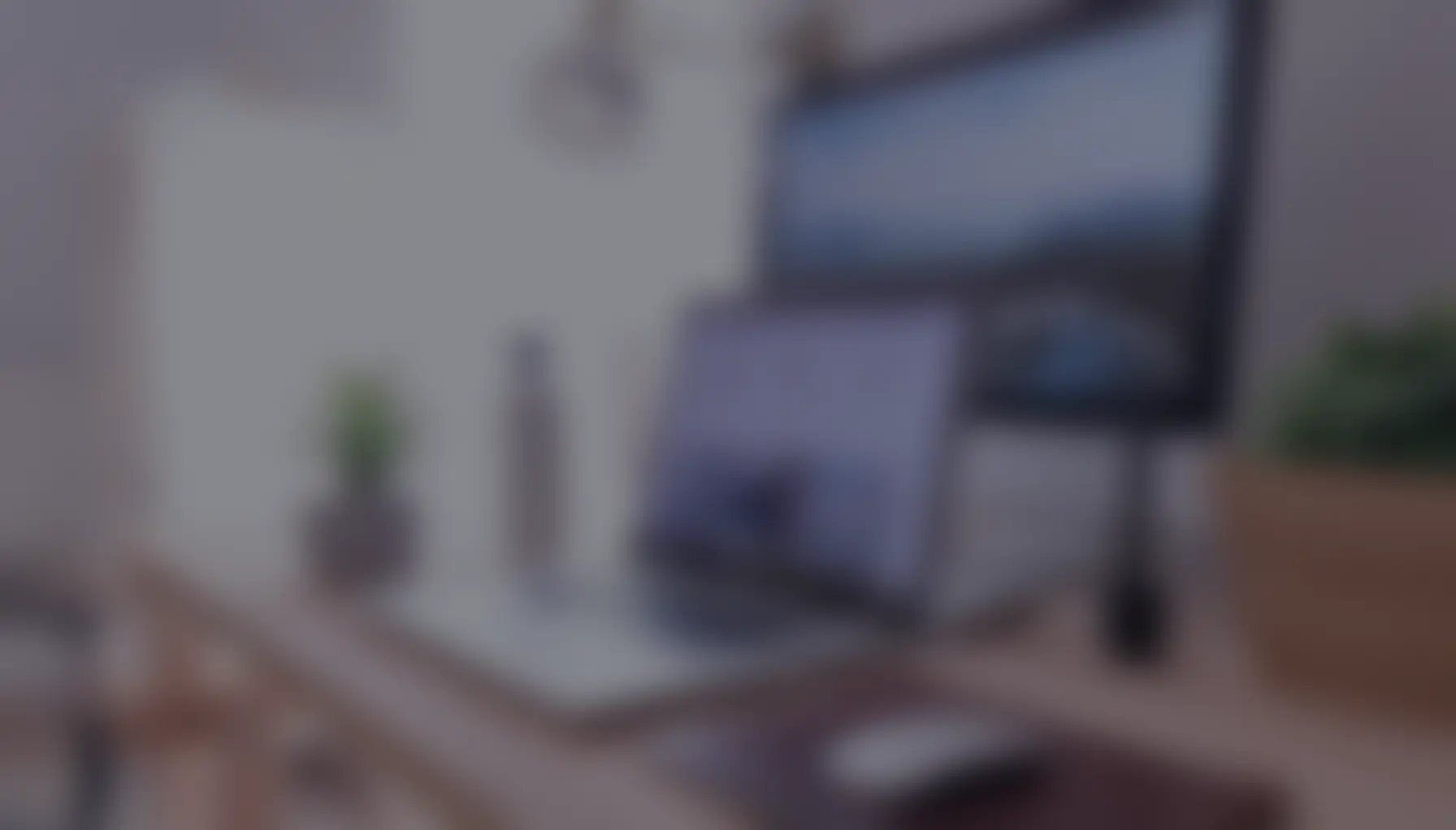
OVERVIEW
EXPERIENCE | In Person |
---|---|
TYPE | Breakout |
TRACK | Data Science and Machine Learning |
INDUSTRY | Media and Entertainment |
TECHNOLOGIES | AI/Machine Learning, Delta Lake, MLFlow |
SKILL LEVEL | Advanced |
DURATION | 40 min |
DOWNLOAD SESSION SLIDES |
When trying to predict what will happen in a sporting event, it’s crucial to understand the strengths and weaknesses of the players and teams involved. At DraftKings, we use Kalman filters, a rating technique most known for its usage in rocket science, to generate ratings that provide estimates of player and team skill in various sporting scenarios. Ensuring these ratings are up to date is essential to ensure markets are profitable, as just a few percent difference in probability can tip the scale between making and losing money. This session will discuss how Kalman filters work, how we use them to predict NBA games, and the Databricks pipelines used to generate these ratings. We also discuss how these ratings can be used end-to-end to generate accurate probabilities that can be used for bookmaking. We will describe our event-driven pipelines to propagate these ratings, using Databricks Workflows and Delta Lake CDF to push data to our models.
SESSION SPEAKERS
Robert Barnes
/Senior Manager, Data Science Engineering
DraftKings
Siu Fai Hsu
/Lead Data Science Engineer
DraftKings