eBook
Standardizing the ML Lifecycle
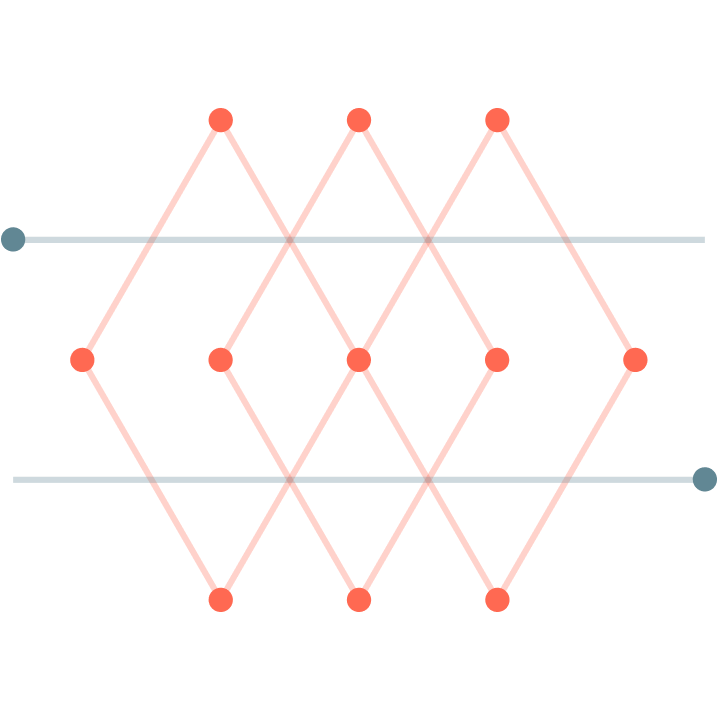
Find out how to successfully build, deploy and reproduce ML models at scale. And learn engineering best practices, discover why MLflow has emerged as a leader in automating the end-to-end ML lifecycle with over 2 million monthly downloads and get an introduction to MLflow’s newest component — Model Registry. The eBook covers:
- Key challenges organizations face when managing ML models throughout their lifecycle
- How MLflow addresses these challenges, including experiment tracking, project reproducibility, and model deployment and management
- How Managed MLflow provides a fully managed, integrated experience with enterprise reliability, security and scale on Databricks