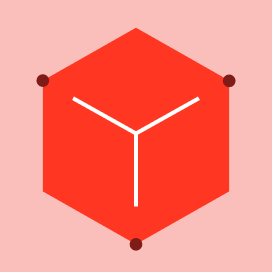
A Guide to Apache Spark Use Cases, Streaming, and Research Talks at Spark + AI Summit Europe
For much of Apache Spark’s history, its capacity to process data at scale and capability to unify disparate workloads has led Spark developers to tackle new use cases. Through innovation and extension of its ecosystem, developers combine data and AI to develop new applications.
So it befits developers to come to this summit not just to hear about innovations from contributors—but to share their use cases, experiences, research, absorb knowledge, and explore new frontiers.
.@databricks @halfabrane & Frank explain how to build scalable genomics pipelines in the cloud @SparkAISummit #ApacheSpark #UnifiedAnalytics pic.twitter.com/Qc6wencS4k
— { Jules Damji } ? (@2twitme) June 6, 2018
In this final blog, we shift our focus to these developers who make a difference, not only in their contributions to the Apache Spark ecosystem but also in use of Spark at scale in respective industries.
Let’s start with CERN’s Next Generation Data Analysis Platform with Apache Spark. Enric Tejedor from CERN will share how Spark is used at scale to process exabyte of data from the Large Hadron Collider (LHC) in innovative ways. Similarly, Daniel Lanza of CERN will also discuss Stateful Structure Streaming and Markov Chains Join Forces to Monitor the Biggest Storage of Physics Data. Two fascinating talks that will demonstrate the Spark’s scope and scalability.
“Traditional data architectures are not enough to handle the huge amounts of data generated from millions of users,” writes Ricardo Fanjul of Letgo. Learn from his talk on why and how Spark is used in Designing a Horizontally Scalable Event-Driven Big Data Architecture with Apache Spark.
From atomic particles’ collision data in physical sciences to genomic data in life sciences, Spark is there to process data at scale. Thanks to advancement in unified analytics at scale, in particular, Spark’s ability to process distributed data and because of cheap cloud storage, Spark enters new frontiers in Health and Life Sciences. Databricks’ Henry Davidge will share: Scaling Genomics on Apache Spark by 100x.
Hearing from engineers who undertake migrating workloads from one architecture to another, in favor of Spark, is always insightful. Three speakers will chart their Spark migratory journeys: first, learn from Manuele Bardelli (OLX) as he will chart his technical migratory journey in his talk, “All-at-Once, Once-a-Day” to “A-Little-Each-Time, All-the-Time”; second, Matteo Pelati (DBS bank) will share his Spark journey: Migrating from RDBMS Data Warehouses to Apache Spark; and finally, Yucai Yu (eBay) will discuss Experience of Optimizing Spark SQL When Migrating from Teradata.
Research heralds technology shifts and innovation: at CERN it led to WWW; at Google, it led to TensorFlow and more; at UC Berkeley AMPLab, it led to Apache Spark. Two research sessions may interest you: Accelerating Apache Spark with FPGAs: A Case Study for 10TB TPCx-HS Spark Benchmark Acceleration with FPGA (Intel) and Spark-MPI: Approaching the Fifth Paradigm (NSLS-II). Continuously processing time-series data using Spark is one of many use cases. To address how to use it with Spark, Liang Zhang (Worcester Polytechnic Institute) will share his research work, Spark-ITS: Indexing for Large-Scale Time Series Data on Spark.
We take flying for granted just as we do driving. But what of the machinery that propels us to our desired destinations? Over time they tire. How do you monitor or detect or predict preventive maintenance? Messrs Peter Knight and Honor Powrie (both from GE) will show how to monitor engines in their talk, GE Aviation Spark Application – Experience Porting Analytics into PySpark ML Pipelines.
Uber’s ride-sharing service is as ubiquitous in a global city as its skyscraper skyline. Learn how Uber uses Apache Spark for running hundreds of thousands of analytical queries every day with their Hudi Platform, built with Spark. Messrs Nishith Agarwal and Vinoth Chandar (both from Uber) will discuss this use case in their talk: Hudi: Near Real-Time Spark Pipelines at Petabyte Scale
Finally, two structured streaming and machine learning related use cases of notable interest: First, from Vedant Jain (Databricks), A Microservices Framework for Real-Time Model Scoring Using Structured Streaming; and second, from Heitor Murilo Gomes (LIAAD) and Albert Bifet (LTCI, Telecom ParisTech), Streaming Random Forest Learning in Spark and StreamDM.
What’s Next?
Take advantage of this promo code JulesPicks for a 20% discount and register now!
Come and find out what’s new with Apache Spark, Data, and AI. We hope to see you in London