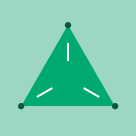
At Databricks, we have had the opportunity to help thousands of organizations modernize their data architectures to be cloud-first and extract value from their data at scale with analytics and AI. Over the past few years, we’ve been fortunate to engage directly with customers across industries and regions about their data-driven aspirations – and the roadblocks that slow down their ability to get there. While challenges vary greatly among industries and even individual organizations, we have developed a rich understanding of the top four habits of data and AI-driven organizations.
Before diving into the habits, let's take a quick look at how organizations have approached enabling data strategies. First, data teams have made technology decisions over time that propel a way of thinking that is based around technology stacks: data warehousing, data engineering, streaming real-time data science, and machine learning. The problem is that's not how business units think. They think about use cases, the decision-making process, and business problems (e.g., customer 360, personalization, fraud detection, etc). As a result, enabling use cases becomes a complex stitching exercise across technology stacks. These pain points aren’t just anecdotal. In a recent survey conducted by Databricks and MIT Technology Review, 87% of surveyed organizations struggle to succeed with their data strategy; it often comes back to their approach of focusing on a ’technology stack.’ Second, there continues to be ample support within IT teams to custom-build solutions rather than buying off-the-shelf offerings. This is not to say there aren’t valid scenarios where custom-built solutions are the right choice, but in many cases technology vendors have managed to solve the majority of the common and low changing use cases enabling teams to focus on more value-added initiatives indexed on creating value for the business faster. Lastly, from a people perspective, organizations have been well-intentioned in their strategies tying technology to business outcomes but have failed because the corporate culture around data hasn't been addressed - in fact in the 2022 Data and AI Leadership Executive Survey, 91.9% of respondents identify culture as the greatest challenge to becoming data-driven organizations.
Luckily, these challenges are solvable – but require a different approach. We’re currently in a “data renaissance” where enterprises realize that to execute on novel data and AI use cases, the legacy model of siloed technology stacks needs to give way to a unified approach. In other words, it’s not about just data analytics or just ML - it’s about building a full enterprise-wide data, analytics, and AI platform. They also recognize that they need to empower their data teams with more turnkey solutions in order to focus on creating business value and not building tech stacks. Organizations also realize that the strategy can’t be some top-down authoritarian initiative but needs to be supported with trainings to improve data literacy and capabilities that make data ubiquitous and part of everyday life. Ultimately, every organization trying to figure out how to achieve all this while making things simple. So how can you get there? These are the top habits we've identified among successful data and AI-driven organizations.
1. Embrace an AI future
When we first started on the Databricks journey, we often discussed how high-quality data is critical for analytics, but even more so for AI, and that the latter, especially for data-driven decision making, will power the future. Over time, as use cases like personalization, forecasting, vaccine discovery, and churn analysis have accelerated and advanced with AI, people are more comfortable with the fact that the future is in AI. The habits are shifting from just asking what happened? to now focusing on why, generating high confidence predictions, and ultimately influencing future outcomes and business decisions. And we see around the world organizations like Rolls-Royce, ABN AMRO, Shell, Regeneron, Comcast, and HSBC are using data for advanced analytics and AI to deliver new capabilities or drastically enhance existing ones. And we see this across every vertical. In fact, Duan Peng, SVP of Data and AI at WarnerMedia, believes “The impact of AI has only started. In the coming years, we’ll see a massive increase in how AI is used to reimagine customer experiences.”
2. Understand that the future is open
There’s an interesting statistic from MIT that states 50% of data and technology leaders say that if given the redo button, they would embrace more open standards and open formats in their data architectures - in other words, optionality. The challenge to this approach is that many data practitioners and leaders associate “open” strictly with open source – and primarily within the context of the on-prem world (i.e., Apache Hadoop). But oftentimes, you've got an open source engine, and it was just about how do you get services and support around it.
In our conversations with CIOs and CDOs about what open means to them, it comes down to three core tenents. First, for their existing solution, what is the cost of portability? It’s great you threw some code on GitHub repos somewhere. That's not what they care about. What they really care about is, if it comes down to it, the viability of moving off the platform from both a capability and cost standpoint. Next, how well do these capabilities allow for plugging into a rich ecosystem, whether it's homegrown or leveraging other vendors products? Third, what is the learning curve for internal practitioners when onboarding? How quickly can they get up to speed?
Every organization is under increasing pressure to fly the plane while it’s being upgraded, but as we get to the point where there are multiple options on how to fly and upgrade, that open nature allows optionality for the future. The optionality enabled by an embrace of open standards and formats is becoming a critical component organizations are increasingly prioritizing in their strategies.
3. Be multi-cloud ready
There are three types of data and AI-driven organizations: those who are already multi-cloud, those who are becoming multi-cloud, and those that are on the fence about multi-cloud. In fact, Accenture went on to predict multi-cloud as their number 4 cloud trend for 2021 and beyond. There are many drivers for a multi-cloud approach, such as the ability to deliver new capabilities with cloud-specific best-of-breed tools, mergers and acquisitions, and requirements of doing business like regulations, customer cloud-specific demands, etc. But one of the biggest drivers is economic leverage. As cloud adoption grows and data grows, for many, spending on cloud infrastructure will be one of the largest line items. As organizations think about a multi-cloud architecture, two things roll up to the top as requirements. First, the end-user experience needs to be the same. Data leaders don't want end-users to think about how to manage data, run analytics or build models separately across cloud providers. Second, in this pursuit of consistency, they don't want some watered-down capability either. There’s a lot of investments that cloud providers are making in their infrastructure. And successful organizations recognize the need to ensure that, as they operate on each cloud, they deeply integrate with them across performance, capabilities, security, and billing. This is pretty hard to get right.
4. Simplify the data architecture
Productivity and efficiency are critical. Ultimately any modernization efforts are aimed at simplifying architectures as a means to increase productivity, which has a domino effect on organizations’ ability to get to new insights, build data products and deliver innovations faster. Organizations want their data teams to focus on solving business problems and creating new opportunities, not just managing infrastructure or reporting the news. To give you an example, Google published “Hidden Technical Debt in Machine Learning Systems,” outlining the tax associated with building ML products. Ultimately the findings concluded that data teams spend more time on everything else from data curation, management, and pipelines than the actual ML code, which is what ultimately will move the business forward.
This begs the question: how can data teams automate as much as possible and spend more time on the things that will move the needle? Many organizations have engineers who love to build everything. But the questions you want to ask are: is building everything yourself the right approach? How do you focus on the core strength and competitive advantage? The fundamental needs of any organization aren't that unique; in fact, many are on the same journey, and third-party solutions are becoming incredibly effective at automating turnkey tasks. Ask yourself how much is it worth to basically lower your overall TCO and be able to move faster? Or as, Habsah Nordin, Head of Enterprise Data, at PETRONAS puts it, “It’s not about how sophisticated your technology stack is. The focus should be: Will it help create the most value from the data you have?”
Why are so many struggling if it is this simple?
The answer: 30+ years of fragmented, polarizing legacy tech stacks that just keep getting bigger and more complex. The figure below is a simplified picture of what is the reality for many. In fact, only 13% of organizations are actually succeeding with their data strategies and it’s owed to their focus on getting the foundations of sound data management and architecture right.
Most organizations land all of the data first and foremost in a data lake, but to make it usable, they have to build four separate siloed stacks. The red dots represent customer and product data that must be copied and moved around these different systems. The root of this complexity comes from the fact that there are two separate approaches that are at odds with each other.
On one hand, you have data lakes, which are open, and on the other end you have data warehouses that are proprietary. They're not really compatible. One is primarily based on Python and Java. The other one is primarily based on SQL, and these two worlds don't mix and match very well. You also have incompatible security and governance models. So you have to do security governance and control for files in the data lake and for tables and columns in the data warehouse. They are like two magnets that instead of coming together, repel each other making it nearly impossible for the broader population of organizations to build on the four habits outlined above. Even the original architect of the data warehouse, Bill Inmon, is recognizing that the current state of affairs outlined in the image above is not what’s going to unlock the next decade-plus of innovation.
The great convergence of lakes and warehouses
As organizations think about their approach, there are only two paths, a lake-first or warehouse-first. Let’s first explore data warehouses, which have been available for decades. They're fantastic for business analytics and rearview mirror data analysis that focuses on reporting the news. But they're not good for advanced analytics capabilities, and working with them gets quite complex when data teams are forced to move all of that data into a data lake just to drive new use cases. Furthermore, data warehouses tend to be costly as you try to scale. Data lakes, where the majority of the world's data resides today, helped solve many of these challenges. Over the years, a bunch of great water analogies emerged around data lakes like data streams, data rivers, data reservoirs that support ML and AI natively. But they don’t do a good job supporting some of those core business intelligence (BI) use cases, and they are missing the data quality and data governance pieces that data warehouses encompass. Data lakes too often become data swamps. As a result, we are now seeing the convergence between lakes and warehouses with the rise of the data lakehouse architecture.
Lakehouse combines the best of both data warehouses and data lakes with a lake-first approach (see FAQs). If your data is already in the lake, why migrate it out and confine it to a data warehouse...and then be miserable when trying to execute on both AI and analytics use cases? Instead, with lakehouse architecture, organizations can begin building on the four habits seen in successful data and AI-driven organizations. By having a lake-first approach that unlocks all the organizational data, and is supported by easy-to-use, automated, and auditable tooling, an AI future becomes increasingly possible. Organizations also gain optionality that was once thought to be a fairy tale. True lakehouse architecture is built on open standards and formats (and the Databricks platform is built on Delta Lake, MLflow, and Apache Spark™), which empowers organizations with the ability to take advantage of the widest range of existing and future technology, as well as to access to a vast pool of talent. This optionality also extends to being multi-cloud ready by not only gaining leverage but also ensuring a consistent experience for your users with one data platform regardless of which data resides with which cloud provider. Lastly, simplicity, this goes without saying, if you can reduce the complexity of two uniquely distinct tech stacks that are fundamentally built for separate outcomes, a simplified tech landscape becomes absolutely possible.
What you should hopefully takeaway is that you're not alone on this journey, and there are great examples of organizations across every industry and geo that are making progress on simplifying their data, analytics, and AI platforms in order to become data-driven innovation hubs. Check out the Enabling Data and AI at Scale strategy guide to learn more about the best practices building data-driven organizations as well as the latest on the 2021 Gartner Magic Quadrants (MQs) where Databricks is the only cloud-native vendor to be named a leader in both the Cloud Database Management Systems and the Data Science and Machine Learning Platforms MQs.