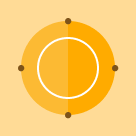
The Executive’s Guide to Data, Analytics and AI Transformation, Part 3: Build Successful Operating Models for Data Teams
This is part three of a multi-part series to share key insights and tactics with Senior Executives leading data and AI transformation initiatives. You can read part two of the series here.
In order to succeed with data, analytics and AI, companies must find and organize the right talent into high performing teams — ones that can execute against a well-defined strategy with the proper tools, processes, training and leadership. Digital transformations require executive-level support and are likely to fail without it — especially in large organizations.
However, it’s not enough to simply hire the best data and AI talent — the organization must want to succeed, at an enterprise level. In other words, they must also evolve their company culture into one that embraces data, data literacy, collaboration, experimentation and agile principles. We define these companies as “data native.”
Chief Information Officers and Chief Data Officers — two sides of the data coin
Data native companies generally have a single, accountable executive who is responsible for areas such as data science, business analytics, data strategy, data governance and data management. The data management aspects include registering data sets in a data catalog, tracing data lineage as data sets flow through the environment, performing data quality checks and scanning for sensitive data in the clear.
Many organizations are rapidly adding the Chief Data Officer (CDO) role to their executive ranks in order to oversee and manage these responsibilities. The CDO works closely with CIOs and other business stakeholders to establish the overall project plan, design and implementation — and to align project management, product management, business analysis, data engineering, data scientist and machine learning talent.
The CDO and CIO will need to build a broad coalition of support from stakeholders who are incentivized to make the transformation a success and help drive organization-wide adoption. To do this, the stakeholders must understand the benefits of — and their role and responsibilities in — supporting the initiative.
There are two organizational constructs that are found in most successful data native companies. The first is the creation of an AI/ML center of excellence (COE) that is designed to establish in-house expertise around ML and AI, and which is then used to educate the rest of the organization on best practices. The second is the formation of a data and AI transformation steering committee that will oversee and guide decisions and priorities for the transformative data, analytics and AI initiatives, plus help remove obstacles.
Creating an AI/ML COE
Data science is a fast-evolving discipline with an ever-growing set of frameworks and algorithms to enable everything from statistical analysis to supervised learning to deep learning using neural networks. While it is difficult to establish specific and exact boundaries between the various disciplines, for the purposes of this document, we use “data science” as an umbrella term to cover machine learning and artificial intelligence.
Organizations wanting to build a data science competency should consider hiring talent into a centralized organization, or COE, for the purposes of establishing the tools, techniques and processes for performing data science. The COE works with the rest of the organization to educate and promote the appropriate use of data science for various use cases.
A common approach is to have the COE report into the CDO, but still have data scientists dotted line into the business units or department. Using this approach, you achieve two goals:
- The data scientists are closer to the business stakeholders, have a better understanding of the data within a business unit and can help identify use cases that drive value
- Having the data scientists reporting into the CDO provides a structure that encourages collaboration and consistency in how work is performed among the cohort and brings that to the entire organization
Data and AI transformation steering committee
The purpose of the steering committee is to provide governance and guidance to the data transformation initiative. The CDO and CIO should co-chair the committee along with one business executive who can be a vocal advocate and help drive adoption. The level of executive engagement is critical to the success of the initiative.
The steering committee should meet regularly with leaders from across the organization to hear status reports and resolve any conflicts and remove obstacles, if possible. The leaders should represent a broad group of stakeholders, including but not limited to:
- Business partners: To provide insight and feedback on how easy or difficult it is to drive adoption of the platform
- Data science: To report on the progress made by the COE on educating the organization about use cases for ML and to report the status of various implementations
- InfoSec: To review the overall security, including network, storage, application and data encryption and tokenization
- Architecture: To oversee that the implementation adheres to architectural standards and guardrails
- Risk, compliance and legal: To oversee the approach to data governance and ethics in ML
- Communication: To provide up-to-date communications to the organization about next steps and how to drive adoption
Partnering with architecture and InfoSec
Early on, the CDO and CIO should engage the engineering and architecture community within the organization to ensure that everyone understands the technical implications of the overall strategy. This minimizes the chances that the engineering teams will build separate and competing data platforms. In many cases, a named enterprise architect (EA) or similar will be required and responsible for validating that the overall technology design and data management features support the performance and regulatory compliance requirements — specifically, whether the proposed design can meet the anticipated SLAs of the most demanding use cases and support the volume, velocity, variety and veracity (four Vs) of the data environment.
From an InfoSec perspective, the CDO must work to ensure that the proper controls and security are applied to the new data ecosystem and that the authentication, authorization and access control methods meet all the data governance requirements. An industry best practice is to enable self-service registration of data sets, by the data owner, and support the assignment of security groups or roles to help automate the access control process. This allows data sets to be accessible only to the personnel that belong to a given group. The group membership could be based primarily on job function or role within the organization. This approach provides fast onboarding of new employees, but caution should be taken not to proliferate too many access control groups — in other words, do not get too fine grained with group permissions, as they will become increasingly difficult to manage. A better strategy is to be more coarse-grained and use row- and column-level security sparingly.
Centralized vs. federated labor strategy
In most organizations today, managers work in silos, making decisions with the best intentions but focused on their own functional areas. The primary risk to the status quo is that there will be multiple competing and conflicting approaches to creating enterprise data and AI platforms. This duplication of effort will waste time and money and potentially erode the confidence and motivation of the various teams. While it certainly is beneficial to compare and contrast different approaches to implementing an architecture, the approaches should be strictly managed, with everyone designing for the same goals and requirements — as described in this post and adhering to the architectural principles and best practices.
Even still, the roles of the CDO and CIO together should deliver a data analytics and AI platform with the least amount of complexity as possible, and one that can easily scale across the organization. Having the data engineering teams centralized, reporting into a CIO, makes it easier to design a modern data stack — while ensuring that there is no duplication of effort when implementing the platform components. Below shows one possible structure.
To learn how you can establish a centralized and cohesive data management, data science and data governance platform for your enterprise, please contact us today.
This blog post, part of a multi-part series for senior executives, has been adapted from the Databricks’ eBook Transform and Scale Your Organization With Data and AI. Access the full content here.

The C-suite dialogue on generative AI
Industry experts reveal winning strategies that minimize risk.