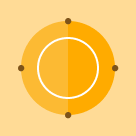
This post is part of a series. Check out Part 1: The Data + AI Trifecta: People, Process, and Platform
In the current rush to adopt artificial intelligence (AI) or large language models (LLMs), it's easy for many business and technical leaders to focus only on the underlying IT overhaul and underestimate the profound shift this technology will have on employees and how they work in the future.
While the technical work to align processes and deploy the right platform is vital, successfully creating a culture of data and AI within an enterprise also requires buy-in from the workforce, from the executive ranks down to entry-level workers.
By helping all employees understand the value that a data and AI-first strategy can bring to their roles, the business results it can help generate, and, ultimately, the career possibilities it can unlock, business leaders can create internal champions that help lower resistance to change and ensure the modernization journey starts strong.
Regarding your plan for people, here are a few winning strategies to keep in mind.
Plan for resistance
No matter how cool the tech is or how significantly it can impact business results, employees will be skeptical and may resist any efforts that require them to break away from their established processes. Even in-house technologists, who may be devoted to the tools they currently use, are likely to put up a fight.
Yet, many executives still underestimate the "immune response" to tech overhauls. Just like your body fighting off an infection, the organization rejects the "new organ" because it's unfamiliar. Demanding every employee immediately adopt a new application or process is a quick way to fail.
Workers must discover how new, AI-powered tools fit into their regular course of work and, more importantly, the impact they provide. That's why flexibility is so critical. For example, many companies are still figuring out their long-term strategy for generative AI. But that hasn't stopped organizations from allowing employees to begin experimenting with some of the popular LLM-backed chatbots in the market.
It's a delicate balancing act. But the sooner you can demonstrate specific value (including what's in it for them) to your employees, the faster you'll be able to drive adoption, experimentation, and business results.
Democratize your data
The best way to find value in data + AI is to make it available to the entire organization.
Large businesses can have tens of thousands of employees with varying roles, using different tools/systems, and requiring disparate data outputs. Without considering what end users will need from the tech, your projects will continue to get stuck in the pilot stage.
Leaders should spend time aligning who should get access to what information (controls), the differences between your users (interface and data format), and where the return on investment is clear.
Many organizations struggle to implement precise security protocols to enable more employees access to corporate information while protecting restricted data. Without a clear strategy for governance, CISOs and other security leaders may immediately halt those exciting new AI investments. And without understanding what outcomes employees should aim to achieve, companies risk wasting money on the wrong AI projects.
Take a typical example: The operations team in a large retailer wants a dashboard that provides real-time inventory updates.
- While that sounds exciting, the team only orders new inventory once a week, so there is no immediate need for real-time updates and related infrastructure investments. It's simply a waste of valuable real-time computing dollars.
- Instead, the team may see a more immediate impact from an AI system that can help predict future demand so the operations team can better plan their inventory needs and be more prepared in the event of a spike in demand.
- Meanwhile, the marketing team may want to start building hyper-personalized customer profiles. But without a plan in place to protect sensitive user information – and how end use will comply with local privacy regulations – the project won't get the green light.
As you are planning your implementation, think about how you will introduce these new capabilities. Some may choose to start with a single business unit, while others will try an enterprise-wide roll-out. Where an organization begins can depend on many factors.
For example, if the underlying platform provides robust data and compliance features, like Unity Catalog's data lineage tool, businesses may feel more comfortable with a broader launch.
However, for less tech-savvy organizations, a gradual approach can help ease concerns around IT security, for example. And success in smaller projects can help those businesses build the confidence they need to invest deeper in data and AI across the enterprise.
We recommend most companies start with "boring AI," or applications focused on automating mundane tasks that may not appear exciting but can result in huge productivity gains. For example, many Databricks customers cite their ability to analyze thousands of documents in seconds instead of hours as one of the most impactful outcomes of using the platform.
By taking a more quiet, behind-the-scenes approach to introducing AI into the organization, employees can start to see the benefits the tech provides with little effort required on their part. It's a tried-and-true tactic in the tech industry and the approach recently helped Robotic Process Automation (RPA) gain enterprise adoption.
Then, as businesses look to take on more sweeping projects, workers will be more willing to break away from their established processes because they've already seen the game-changing impact (and internal recognition) that AI can provide.
Organize and Track for Consistency, Capability, and Accountability
There are also several different approaches companies can take to introduce data and AI into their business.
You may want a team churning out quick AI projects that can start to pinpoint common roadblocks and best practices to make future AI efforts more successful. In parallel, you may also have a team dedicated to driving a particular priority project – a bespoke use case that requires longer-term focus, skills, and deep technology development.
But even those approaches can vary dramatically by company. For some, a central team of data engineers and scientists may be the right approach to extend expertise and value across the organization.
A core data science team that is aligned with the different business units can use the operational knowledge in those teams to build tailored solutions. Meanwhile, a centralized data engineering team can focus on broader data hygiene, governance, and curation. This provides a good balance between control and autonomy.
Having a centralized team can also make it easier to track all the ongoing projects so internal advocates can easily elevate the most promising pilot initiatives and have evidence to support continued investment in those projects. And as new technologies emerge, the business could be in a much better place to more quickly deploy them in key operations.
However, for others, a decentralized approach, where different units are empowered to pursue data and AI initiatives independently, may be better. In that instance, a central team might still help the organization establish controls, policies, frameworks, and best practices. And once that foundation is created, employees are free to start building on their own.
It's a way for companies to let the creativity and subject matter expertise of the workforce take charge, while still ensuring there's some standardization across the different data and AI projects.
Communicate What to Expect
Driving a successful data and AI strategy requires a change in behaviors. And changing how people work is foundationally built on communication.
Leaders need to help their organization understand why the change is happening, the impact on operations, and how employees are able to provide feedback along the way. The latter is critical, as any efforts to simply push technology changes through the organization can be met with stiff internal resistance.
Communications need to be shared at all levels of leadership. Two fundamental components of every communication should be a reminder of the strategic relevance of data + AI for the organization and best practices or lessons learned. A solid communication plan can help reinforce good behaviors and course correct where things are not going well.
The communication plan should be shared on multiple channels, frequently over time. Too often we have seen organizations send out a single email and then presume that everyone is on board. But it takes more than that. The data and AI strategy must be reflected in emails, newsletters, all hands, knowledge management systems, and data readiness events – anywhere possible to ensure that change leaders are meeting their people where they prefer to learn.
And the communications should be tailored around how different employees will get value data and AI. For example, sales leaders might want to frame the strategy around how the technology will help them pinpoint new business leads much easier. Meanwhile, the recruiting team is much more interested in how data and AI can help them quickly find the best candidates from thousands of job applications.
Upskill and Encourage the Team
The rapid rate of technological change means that companies and employees are never done learning. An enterprise's ability to create a continuous learning culture for new data and AI technologies will generate short-term results, while also preparing the business for long-term adoption.
These education efforts should take many different forms.
- AI to train on AI: Businesses can start on their AI journey by using the technology to actually help educate employees on AI. By tapping models that can turn one learning concept into many different outputs (e.g., infographic, white paper, or a TikTok video) that will resonate with your various audiences.
- Communication is key: Leaders need to get the business talking about data and AI regularly. This could mean creating internal communities or groups focused on the technology, forums where employees can share best practices or get answers to common questions, and regular highlights of success stories to sustain the excitement around data and AI.
- Offer many different education pathways: Some employees may want to click-through self-service modules, while others prefer instructor-led courses. There will be workers who simply want a quick workshop that arms them with the basic skills needed to get started, while others will want to obtain an advanced certification. Businesses should support all of these different pathways to ensure success.
- Cultural reinforcement and safety: Not every data and AI project is going to be a success. Businesses need to allow employees to experiment with the technology in a safe and financially responsible manner. For example, hackathons are a great way to encourage more "blue sky" research. Recognize and reward employees who are leveraging data and AI, and showcase their results and innovations to inspire others. Adjusting job descriptions to focus more on data and AI helps to further solidify the technology's role in the enterprise.
- Go big: It can't just be C-Suite level executives or the most technical members of the workforce who are trained to use data and AI in their jobs. Success depends on usage from the top brass down to front-line staff. And training and education efforts should reflect that.
Scaling data and AI across the business is one of the toughest modern technology challenges that organizations face. It can easily exhaust leaders and take IT teams to the brink of sanity.
By taking time in the beginning to think about not just the AI infrastructure, but also about organizational needs, businesses can more seamlessly weave data and AI into their whole operation - and realize new levels of success.
Curious of other ways CIOs are leveraging data and AI within their organizations? Read our joint report with MIT Tech Review on how executives are laying the foundation for data and AI growth.