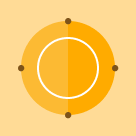
The secret to good AI is great data. As AI adoption soars, the data platform is the most important component of any enterprise's technology stack.
It’s increasingly clear that Generative AI systems won’t be one monolithic, but rather a combination of many different components that must work together. And while data is one of the most important pieces, there are many other functions required for enterprises to actually deploy the models into the real-world.
That’s why, when businesses are looking to build the foundational platform that will support the breadth of their data and AI needs, they should keep three core pillars in mind: gathering the data, governing it and creating value from it.
Data intelligence platforms
Increasingly, companies are realizing that significant positive outcomes are possible when each of these pillars is managed through one platform. We call this a Data Intelligence Platform, and soon it will become the most important market in enterprise software.
The DI Platform should enable companies to:
- Operationalize their data, whether that’s building a custom LLM or enabling anyone in the organization to generate the code to run a SQL query.
- Tap into any commercial or open source AI model they want, then customize or fine-tune it with their own proprietary data,
- Query the information like they are using a search engine, with a natural language prompt, and;
- Easily bring in data from partners, and then quickly visualize the resulting insights.
And as information flows to all these new use cases, companies should be able to pin-point with precision detail where data is moving and for what purpose – as well as put guardrails around who or what can access the information.
Below we dive into the key considerations companies should keep in mind when choosing a DI Platform.
Consolidation
In most enterprises today, the critical tasks of storing, overseeing and using data are split across many different tools. In fact, according to a recent survey of technology executives by MIT Technology Review and Databricks, 81% of large organizations, or those with over $10 billion in annual revenue, currently operate 10 or more data and AI systems.
Relying on so many different technologies is not only expensive, it’s a data unification and governance nightmare. It’s why, alongside future-proofing their IT foundation, companies are also trying to consolidate the number of tools they are using.
That unification of data - with the right controls in place - helps significantly reduce IT complexity. With the whole company increasingly operating on a single platform, managing the underlying data becomes easier. It eliminates common questions like: “Where is the most recent supply chain data?” and “What are the most recent supply chain business rules?”
But it’s not just about the underlying data. Pivoting to a modern data platform can help the business save money on AI experiments. Building models on top of data warehouses will almost always be more expensive than running them on a DI Platform that’s built around the lakehouse architecture. It’s why 74% of organizations have already made the move to the lakehouse, per research from MIT and Databricks, and are relying on it as their foundation for the AI era.
And because many of the data-related tools that enterprises are running are built in-house, shifting to an end-to-end platform that’s usable by anyone in the organization reduces the reliance on highly-skilled engineers, while also democratizing the use of data within the organization.
There’s no AI without data governance
Data IP leakage, security concerns and worries over the improper use of corporate information. These are all fears we hear regularly from enterprise executives. And as governments continue to ramp up pressure on companies to protect customer data, businesses are rightly concerned that any misstep could earn them the attention of regulators.
As more governments require consumer information to be stored locally, for example, businesses have to be able to track how data is moving through the organization with precision detail. But it’s not only data compliance. Increasingly, businesses have to worry about AI compliance.
Companies will soon have to be able to explain how they are training their models, what data they are using to do that, and how the model ultimately came up with the results. In fact, some industries – like insurers or financial services providers – are already required to prove to regulators that the technology they use to generate claims decisions or manage credit risk isn’t harmful to the consumer.
Managing and using data has become too complex an operation for enterprises to still rely on bespoke tools for every step in the process. It adds unnecessary complexity and makes building the workflows to support predictive analytics that much more complicated.
Consolidating that work onto one platform makes it much easier for organizations to track their AI efforts and explain to regulators how the models work. Lineage tools will enable the businesses to track where the data is coming from, where it’s going, and who is using it.
Build to Scale
There are three key steps to launching any new AI solution: preparing the data, fine-tuning the model, and deploying the end application.
First, companies must pinpoint relevant and timely data, and get it into the hands of the proper experts. This remains a significant challenge for businesses. Not only is information spread across so many different places, but deciding which employees can access what information can’t be handled by a one-size-fits-all policy.
Most AI models also can’t be instantly launched into operations. Companies need to be able to continually evaluate and change the models to make sure they are producing the most accurate and helpful results while protecting their data. That’s where a capability like Lakehouse Monitoring, Databricks’ tool to oversee data pipelines, becomes so vital.
And ultimately, AI isn’t useful unless it actually gets used. That means companies need to be able to hide all the complexity that goes into developing and running the model with a consumer-friendly application that enables developers and other end users to instantly start building.
Tracking each of these steps separately adds enormous complexity to the process. Instead, a DI Platform that can handle the whole model development cycle, from data discovery to the end application, as well as provide the monitoring tools needed to continually improve the model.
But while the underlying platform is important, it’s just one step in the process. Check out our previous blog for insights on how to get your employees and culture ready for the AI future.