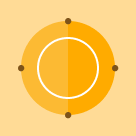
With the pace of modern business and the competitive need for more and more data, organizations now correctly ask whether their data management practices still support their business strategies—especially those now trying to wrangle data across multiple platforms to achieve sophisticated real-time analytics and generative AI outcomes.
- Does this most valuable asset enable better agility to take advantage of new trends and opportunities?
- Are they getting the insights they need to facilitate data-driven decisions?
- Can they collaborate on the growing amount of data or is it segmented into departmental silos with varying degrees of governance, quality and cost control?
If the answers to these questions are unknown, it’s time to create or revisit a data strategy.
What is a Data Strategy?
A data strategy is a comprehensive plan that outlines how an organization intends to collect, manage, govern, utilize and derive value from its data. It becomes the roadmap for aligning data activities with broader business goals and sets the objectives for data democratization and the priorities for the data management strategy.
What is the purpose of a data strategy?
The data strategy requires flexibility to adapt as the business strategy changes. If the business goal around your data activities is to increase efficiency/performance and grow revenue, your strategy will be different with a more transformative goal to become data-driven and monetize data as a product. But both call for a unified approach for gathering, maintaining, securing and analyzing data.
The difference between data management and a data strategy
Don’t confuse data strategy with data management. Data management includes the processes and systems used to collect, store, orchestrate and share data across the organization. It focuses on the operational aspects such as the data infrastructure and data lifecycle management.
Why is Having a Data Strategy Important?
The data strategy impacts all aspects of a business. Business value is unlocked only when data silos are removed and employees, stakeholders and customers are trained to extract insights.
Data democratization happens when everyone in an organization has the training and tools necessary to access and understand the data. To gain confidence and broad adoption, every component of the data pipeline requires a great amount of research and buy-in up and down the organization to determine the systems and practices the teams will use when collecting, storing or interacting with data.
When data and AI is available for all, it reduces dependence on the technical staff and creates frameworks for data privacy and organizational control. But making data-based business decisions also involves a cultural shift with greater levels of transparency, collaboration and quality control.
Data governance and AI go hand in hand. Building effective large language models (LLMs) would not be possible without high-quality, secure data. Not only do compliance-bound companies have to precisely track how data is moving through the organization, if they are training AI models, they will have to explain to regulators how those models work and where the data came from ensuring data quality. Separate data and AI platforms create governance silos that result in limited visibility and explainability of AI models.
A solid data strategy facilitates adoption and helps plan for changes to workflows and new ways people can interact with data. When data is democratized across an organization, it carries risk. The strategy should set governance priorities around ownership and access and help identify gaps to take corrective actions to improve operations, enhance customer experience and drive growth.
The data strategy defines the metrics to track for successful outcomes but also helps plan for experimentation and new AI investments.
The Benefits of a Data Strategy
Without a strategy for effective governance and the ability to share data, businesses could not embrace exciting technologies like machine learning and generative AI to innovate and find new value in their data. In addition to removing bottlenecks and improving data democratization, a data strategy frees the data teams from constant requests and tickets to focus on more advanced work like machine learning (ML) and AI models. Potential benefits include:
Informed decision making
Real-time data analytics reporting allows organizations to quickly address new challenges or opportunities with accurate data-driven business decisions.
Increased efficiency and cost savings
Eliminate inefficient processes that hinder decision-making and lead to poor outcomes.
Helps create a data-focused culture
Having a data strategy provides a roadmap for the entire business with buy-in from the top, training and improved data literacy throughout the organization.
Data governance/reduced risk:
Knowing data can be collected, organized and shared in compliance with any relevant regulations such as the EU's General Data Protection Regulation (GDPR) and the Health Insurance Portability and Accountability Act (HIPAA) will help ensure strong reliability and security and mitigate business risks.
Improves customer experience
The ability to collect and analyze real-time customer behavior data allows businesses to personalize their online experiences and offerings. Get closer to customers with engagement strategies using AI, ML and automation to build a foundation for personalization.
How a Data Strategy Can Help Achieve Analytical and AI Maturity
In determining a data strategy for tomorrow, it can be helpful to examine the current analytical maturity of the business. Organizations just storing unstructured data have virtually no analytics other than what they can surmise from spreadsheets. Their data is probably siloed, and they lack the skills to analyze the raw data. At this stage, they should be determining what kind of questions they want to ask to get more value from their data.
As organizations build their analytics muscles, they gain advanced SQL and predictive modeling skills to begin forecasting trends. They use data modeling tools to automate the orchestration of information. At the highest level of analytics maturity, organizations get actionable insights on why something changed and how to respond. This level of prescriptive analytics involves working with large data sets using machine learning algorithms and AI models.
To achieve that, data storage is typically centralized as a “single source of truth” as different functions and business units then transform it with their own labels (metadata) and reports according to their requirements in a more blended data strategy.
Understanding the level of analytical maturity can point out where an organization needs to be and what it needs to focus on to remove the roadblocks. That’s what a data strategy is for.
How to Build a Data Strategy
A good data strategy starts with an honest assessment of “Where am I and why am I there?” when it comes to the current business value of a data estate. This evaluation should help identify gaps in resources, processes, tools and people. Getting the right people in the room is the first of many steps:
Put together a team
- Who is an essential stakeholder on the data strategy team? This cross-functional team should represent stakeholders that have access to relevant data, with a shared goal of breaking down silos to democratize data for everyone in the organization.
Choose your objectives
- Define the business goals and outcomes for the data strategy, such as increasing operational efficiency, improving customer experience, revenue growth or reducing costs and interpreting the data to make it more meaningful and actionable.
Evaluate your current situation
- What are the current data sources, where they are located, who owns them and who has access to them? What systems, tools and processes are implemented and what is the level of analytical maturity currently in place? Determine what other data sources are available and identify any gaps or challenges that need to be addressed.
Create a roadmap
- What is the action plan—the initiatives and projects needed to achieve the desired outcomes? This roadmap should include timelines, resource requirements and milestones to track progress.
Establish clear, overarching policies
- What are the principles and standards that ensure high-quality data is used? Outline the key roles and responsibilities, timelines and resources required.
Invest in any new technology required
- What new technologies or approaches will unlock new opportunities such as data integration platforms and data management solutions? Do you have the analytics capabilities essential for extracting insights from the collected data and visualizing the information in an understandable manner? Which existing resources, systems and tools can be leveraged? A robust infrastructure will need to handle vast amounts of data.
Educate and build a data-first culture
- What new skills are needed to stay on top of emerging data types, changing business needs and technology advancements? Is there a dedicated space to experiment and test new machine learning models and AI features?
Monitor and regularly reassess
- What are the measures of success? Use meaningful metrics and define KPIs that align with business objectives. Establish benchmarks to measure progress over time and use data-driven insights to adjust as needed to remain aligned with evolving business objectives and market conditions.
Challenges of Implementing a Data Strategy
When it comes to data strategy and the team, limited data literacy and lack of tools will thwart any true democratization of data. Personnel should be trained to work with complex datasets and understand how they can be used to drive business value.
Getting people on board
Strategy execution is also hampered without buy-in up and down the organization. Successful data-driven companies create a culture of data and AI with shared values, attitudes and behaviors that foster data-driven decisions and improve business performance.
Ensuring it is achievable and sustainable
Re-engineering a data strategy and changing a culture is a process. Make sure the goals and objectives are achievable and sustainable. Focus on what’s viable and valuable in the short term and build organizational confidence and capabilities with each successful result.
Choosing the right type for your business
Choosing the right type of data strategy can also be a challenge. Over-indexing on reliability, security and compliance could prevent experimentation and innovation.
Data Strategy Case Study: Databricks and Thomas
A key precept of a modern data strategy is “data for the many, not the few.” Data is core to everything for global talent assessment provider Thomas, which transformed its consultancy operation to a SaaS-based self-serve business model. The shift required a shift from batch processing to working with real-time events. That increased the types and amount of data captured by 400% to power new machine learning and AI capabilities, analytics and BI reporting.
In addition, Thomas’s data estate was not well integrated, causing the data team to spend more time and resources moving data between systems. Moving to a data lake as the single source of truth allowed the data team to identify and resolve problems more effectively and efficiently and drive self-serve analytics.
Luke Treglown, Senior Manager of Data Science at Thomas, writes that data insights are “being delivered ~40% faster than our previous data stack. We are able to implement fixes and new solutions far quicker, freeing up ~20% of our data science team’s time to focus on innovation and experimentation.”
Start Unlocking The Power of Your Data Today
Looking for more tips and best practices to define your data strategy? We’ve got you covered.
- Data Strategy Blog - Subscribe to our data strategy blog channel at https://www.databricks.com/blog/category/data-strategy
- Additional Resources - Get even more data strategy tips and hear case studies from other leaders at https://www.databricks.com/why-databricks/executives
- Partners - We have hundreds of partners to help you on your journey, learn more at https://www.databricks.com/company/partners/consulting-and-si