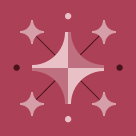
Generative AI (GenAI) is moving incredibly fast. So much so, that in less than two years, GenAI has emerged as one of the most exciting and transformative technologies, empowering enterprises across diverse industries to drive innovation, enhance productivity, and deliver exceptional customer experiences. At Databricks, we've seen exponential growth in the demand and development of GenAI applications across our platform from every sector of industry, be that communications, energy, financial services, healthcare and life sciences, manufacturing, public sector, media and entertainment, or retail and consumer goods.
As we approach Data + AI Summit, we'll be bringing together a global community to deliver on the promise of data intelligence for all. GenAI will be a core theme of the event, and we'll be joined by more than 130 partners supporting the development and deployment of GenAI applications.
In this blog, we explore how leading enterprises in various sectors are leveraging generative AI to revolutionize their operations and stay ahead of the curve:
Communications
The communications industry is undergoing a transformative shift – from utility to value added service provider – and data and AI is at the core of providing better consumer, network and partner experiences. In this era where data and AI are being used to scale efficient growth, communications service providers are turning to GenAI applications to help drive stronger customer engagement while lowering the cost to serve and operate. According to the latest GenAI report from TMForum, communications service providers (CSPs) are facing a number of challenges when it comes to the development and deployment of GenAI applications, including ensuring data accuracy and traceability, as well as addressing biases and "hallucinations" in large language model outputs. This is an area Databricks is hyper focused on addressing. Today, we are empowering teams like AT&T, T-Mobile, Rogers, Comcast, and more to address these challenges and realize the full potential of GenAI. Here are some of the most critical use cases being implemented:
Enhanced Customer Support: Using RAG to augment agents (make them more productive, reduce wait time). Generative AI-powered chatbots and virtual assistants are transforming the customer service landscape in the communications industry. These intelligent agents can handle a wide range of inquiries, provide instant responses, and offer personalized solutions, all while freeing up human agents to focus on more complex tasks.
Personalized Content Creation: Generative AI enables communications companies to create highly personalized content, from targeted marketing campaigns to tailored customer support interactions. By analyzing user data and preferences, these AI models can generate unique content that resonates with individual customers, fostering deeper engagement and loyalty.
Field Technician Support: Bot-like experience for employees and contractors to ask bot questions to figure out quickest path to resolution - network dependencies. Generative AI is being leveraged to optimize network performance and resource allocation in the communications sector. By analyzing vast amounts of network data, these AI models can identify patterns, predict potential issues, and recommend optimal configurations, leading to improved service quality and operational efficiency.
Here are a few talks you won't want to miss at Data and AI summit related to the communications industry and building a strong foundation for data and AI, including GenAI applications:
Organization | Abstract |
---|---|
T-Mobile | T-Mobile's Data and AI Evolution with Connected Data Architecture The T-Mobile Network Engineering team has been working with Databricks Lakehouse for years to help solve the massive scale (up to 600TB/day of ingest) challenges. We can now link previously siloed subscriber data assets to network data across a unified multi-tenant data platform facilitated by Unity Catalog. This modern Lakehouse approach streamlines collaboration, reducing data duplication, complexity, and costs. Enhanced data sharing accelerates innovation by breaking down data silos. |
AT&T | AT&T's Journey Toward a Serverless Data Intelligence Platform Data teams today are faced with the need to rapidly onboard an increasing number of AI and data engineering projects while simultaneously accelerating time to value. Join us for an insightful session on AT&T's evolution on the Databricks Platform as they scale beyond their initial use cases to enable several lines of businesses. From automated pipelining with Delta Live Tables to serverless Databricks SQL warehouses and AI/ML use cases, Databricks has empowered the streamlining and acceleration of new data products at AT&T. However, it can be challenging to meet complex security and connectivity requirements when workloads are not deployed on your own network. This session describes how AT&T meets stringent security and regulatory requirements while adopting the Databricks serverless platform, starting with serverless SQL warehouses. We also describe the latest enhancements that Databricks Platform team has built in partnership with AT&T. |
Energy
Data and Artificial Intelligence (AI) are becoming as fundamental to the energy sector as electricity itself. In the current evolution of energy systems, analytics and AI are revolutionizing the production, distribution, and consumption of energy. With renewable sources now contributing to 30% of global power generation, an increase of 10% since 2010, the application of data and AI has become critical. These technologies are leading the charge in managing an increasingly diverse energy mix and in advancing the electrification of consumption behaviors.
As global momentum shifts towards renewable energy and decarbonization, propelled by changing consumer preferences and market forces, data and AI are proving to be essential in managing the integration of legacy and emerging energy infrastructures. This transition is characterized by price volatility with significant economic ramifications. McKinsey predicts that strategic deployment of data analytics and AI could generate up to $5 trillion in value over the next decade and is crucial for achieving the net-zero emissions goal by 2050.
Looking ahead, industry leaders in the energy domain are set to leverage data and AI to excel through this period of transformation and to buffer against the risks posed by market instability. Generative AI, in particular, is expected to have a significant, transformative impact. Below are some key applications currently observed among our clients in the energy sector:
Asset Performance Management: The energy industry is all about physical assets (rigs, grids, wind turbines, and pipelines) that are operated for long periods of time, often running into decades. This complex machinery generates high-frequency sensor data (millions of data points per equipment) that can be used to reduce unplanned downtime, maximize output and drive a high degree of process automation, ultimately driving safer operations and higher performance.
Renewable Energy Forecasting: The inherently variable nature of renewable energy sources like wind, solar, and hydro necessitates building advanced capabilities in prediction and planning. The capabilities to forecast renewable energy generation factoring in dynamics such as wind speed, solar irradiance, and hydro inflow, etc. in order to match better with expected load and price signals - will help companies reduce uncertainty, efficiency, and profits in the renewable energy market.
Grid Optimization: With the deployment of Advanced Metering Infrastructure (AMI), utilities can gain real-time visibility into grid conditions. With AI capabilities, companies can better forecast load, predict outages, and uncover usage patterns to help utilities drive better servicing of demand, at lower cost and lower disruptions, opening the door to a more proactive and predictive approach to grid management.
Energy Trading: Electricity markets are highly volatile and require very short timeframes for decisions: lack of data accuracy and timeliness in analysis can result in significant financial downside. By bringing together asset performance predictions, market pricing, and risk management practices, companies can optimize value capture through better day-ahead offers and optimize trading strategies.
Organization | Abstract |
---|---|
Shell | AI and The Lakehouse: Shell's Journey Towards Effective Data Governance Join our speakers from Shell Energy as they discuss the challenges and solutions in implementing a data strategy and lakehouse in a large, diverse organization. They'll share their experiences, including initial hurdles in data strategy and governance, and how they used Unity Catalog and a business-owned data product approach to overcome them. They'll delve into the concept of Data Mesh, discuss the roles of the product team and the customer, and provide real-life examples. They'll also share insights on using analytics, PowerBI, ML models, and AI for Data Governance. This session is for professionals grappling with data strategy and governance, offering insights on aligning goals, identifying use cases, team organization and scaling operations. Attendees will gain a deeper understanding of achieving success with data, analytics, and AI. The session, aimed at an intermediate level, will include code snippets and working code demonstrations. |
Ordnance Survey | The AI of Where: Unleashing the Power of Gen AI on Geospatial Data In this session, we will explore cutting-edge GenAI applications in location intelligence, offering a unique perspective on the evolution of spatial data analysis to enhance Ordnance Survey's ability to deliver new insights for Great Britain. Discover innovative uses of Large Language Models and the Segment Anything Model in unlocking geospatial dataset potential. Learn how advanced AI techniques automate real-world feature extraction from diverse earth observation data. Real-world examples and case studies illustrate generative AI's effectiveness in solving complex challenges, showcasing Ordnance Survey and Databricks' groundbreaking contributions to geospatial intelligence. Delve into the pivotal role of grid index systems in scaling earth observation and geospatial datasets, optimizing AI model applications. Understand how these systems efficiently manage large-scale geospatial data, seamlessly integrating with generative AI for enhanced analytical use cases at OS. |
Financial Services
In the fast-paced world of finance, firms are constantly seeking ways to remain competitive through increased automation, accelerated product innovation, and improved operational efficiency. Executives believe that Generative AI will play a significant role in helping Financial Services Institutions (FSI) automate, streamline and become more efficient. FSIs have started to invest in AI capabilities to analyze vast amounts of data and provide insights that augment human intelligence. For example, Bloomberg announced large-scale language model (LLM) 'Bloomberg-GPT,' a purpose-built 50-billion parameter LLM specifically for the financial industry, while Morgan Stanley launched AI @ Morgan Stanley Assistant–the tool gives financial advisors speedy access to a database of about 100,000 research reports and documents, and JP Morgan reportedly used a Chat-GPT-based language AI model to analyze 25 years of Fed speeches to uncover potential trading signals. Other use cases include:
Personalized Investment Strategies: Generative AI is revolutionizing the way financial institutions develop investment strategies. By analyzing customer data, market trends, and economic indicators, these AI models can generate personalized investment recommendations that align with an individual's risk profile and financial goals.
Automated Compliance and Regulatory Monitoring: Generative AI is proving invaluable in the financial services industry by automating the monitoring of regulatory changes and compliance requirements. These AI models can quickly parse through vast amounts of legal and regulatory documents, identify relevant updates, and generate tailored compliance reports, ensuring that organizations stay ahead of evolving regulations.
Fraud Detection and Prevention: Generative AI is playing a crucial role in enhancing fraud detection and prevention in the financial sector. By analyzing transaction patterns, customer behavior, and other relevant data, these AI models can identify anomalies and potential fraudulent activities, enabling financial institutions to proactively mitigate risks and protect their customers.
Organization | Abstract |
---|---|
Northwestern Mutual | Accelerating Operational Excellence with GenAI This session will showcase how our Financial/Insurance company implemented a Retrieval Augmented Generation (RAG) system to enhance customer service efficiency. We'll provide an overview of our RAG architecture and how we used Databricks to build a robust data pipeline for indexing content and collecting user feedback. We'll explore innovations like multi-stage content chunking, advanced search retrieval techniques, and an evaluation framework for optimization. Our feedback loop using Databricks workflow improves the RAG implementation. We'll also highlight our unique orchestration layer that accelerates Generative AI use cases. By the end, you'll understand how Generative AI can transform customer service operations with strategies for efficiency and automation. These learnings apply to any customer service organization striving for operational greatness. |
JP Morgan Chase | Accelerating J.P. Morgan Data Product Development in the Age of AI Artificial intelligence is fast becoming a generation-defining technological breakthrough. Hear how technology leaders from JP Morgan Payments Data team are putting AI to use, from encouraging agile technical experimentation to driving real business outcomes. You'll also learn how to plan your own AI investments by evaluating emerging use cases and shifting organizational culture. See how JP Morgan Payments Engineering have built a self-service innovation factory with Databricks and MLflow for Data Scientists, Data Analysts and Data Engineering. |
Banco Bradesco | GenAI Platform to Democratize Use and Maintain Governance Despite Bradesco being one of the largest banks in Latin America, the bank deals with time-consuming processes. Seeking a solution, Bradesco turned to GenAI, which has the potential to revolutionize products and create game-changing experiences. To democratize the use of GenAI within the bank while ensuring security, we developed a GenAI platform using a lakehouse architecture to guarantee performance and scalability. Also, we used APIs to access established LLM models that are structured in functionalities such as summarization into microservices. |
Avanade | Integrating Mosaic AI and Data Security in Advanced Machine Learning With LLMs, data compromise and security are always huge challenges. We will explore the innovative strategies and best practices for embedding security within this LLM framework, ensuring that the acceleration and optimization of ML models do not compromise data integrity and confidentiality. The talk will highlight case studies from various industries, illustrating the successful implementation of secure and efficient ML solutions. |
Healthcare and Life Sciences
While healthcare and life sciences have been exploring the use of data and AI to improve patient care, medical research, and clinical decision-making for years, large language models (LLMs) have elevated AI to a strategic initiative that healthcare leaders can no longer ignore.
Accelerated Drug Discovery: Generative AI is revolutionizing the drug discovery process in the healthcare and life sciences industries. These AI models can analyze vast amounts of molecular data, identify promising drug candidates, and simulate the effects of potential treatments, significantly reducing the time and cost associated with traditional drug development.
Personalized Treatment Recommendations: Generative AI is enabling healthcare providers to deliver more personalized and effective treatments. By analyzing patient data, medical histories, and research findings, these AI models can generate tailored treatment plans and medication recommendations, improving patient outcomes and reducing the risk of adverse reactions.
Intelligent Medical Imaging Analysis: Generative AI is transforming the way medical professionals analyze and interpret medical images, such as X-rays, CT scans, and MRI scans. These AI models can detect subtle patterns and anomalies, assist in diagnosis, and provide recommendations for further investigation, enhancing the accuracy and efficiency of medical decision-making.
Organization | Abstract |
---|---|
CVS Health | Building the World's Largest RAG for Knowledge Management at CVS Health CVS Health has 300,000+ employees and a diverse portfolio of businesses. Managing knowledge across a complex organization is challenging when knowledge is spread across various sources used by thousands of teams. Finding info you need to perform work can be a challenge in a big company. How do we ensure our employees can access the most relevant/reliable information at the right time/place? We set out to solve the knowledge management problem and improve the experience at CVS Health. We'll highlight our approach to building the world's largest RAG system for knowledge management. Many RAG systems have been POCs that fail to scale. We will describe how we use RAG to create a unified and scalable knowledge platform that serves many use cases and BUs, sharing tech and org challenges we faced, how we overcame them, and strategies to tackle this massive problem. This talk aims to inspire and inform other practitioners and researchers interested in applying RAG at scale to their domains and problems. |
Providence Health | Healthcare Data Intelligence with Unity Catalog: Providence's Journey Join us for a transformative session as Providence shares its journey in implementing Unity Catalog. Discover how Providence analyzed its current state to define governance, right-size clusters, consolidate workspaces, and centralize data, all while collaborating closely with Databricks and leveraging best practices to optimize performance and manage costs effectively. Gain insights into the challenges and strategies employed, including cleanup, optimization, and mindset shifts. Learn how implementing Unity Catalog and Lakehouse architecture has unlocked improved data intelligence, sharing, collaboration, and support for Providence's most critical clinical and operational GenAI and LLM use cases. Take advantage of this opportunity to learn from Providence's experience and apply these lessons to your data management journey. |
Mankind Pharma | Leveraging GenAI to Accelerate Innovation Mankind Pharma is a leading Indian pharmaceutical manufacturer. Its mission is to build a healthier India by focusing on drug affordability, quality and accessibility. It is ranked number one by prescriptions and number three by volume in India in FY23. More than 20 brand families generate over $1b+ annual business of which more than 97% comes from India. This business is supported by 15k reps, 13k+ stockists and 25 manufacturing facilities spread all over India. Mankind Pharma's digital transformation journey is leveraging GenAI to accelerate business processes in multiple areas. In this session three use cases will be covered:
|
Manufacturing
Artificial Intelligence (AI) is revolutionizing the manufacturing sector by streamlining production processes, increasing efficiency, and reducing operational costs. AI-powered systems enable predictive maintenance, minimizing downtime by forecasting machine failures before they occur. They also enhance quality control through precise defect detection and facilitate optimized supply chain management via advanced analytics. By integrating AI into manufacturing, companies can achieve greater agility, improve product quality, and drive innovation, paving the way for smarter, more sustainable manufacturing practices.
At Databricks, we advocate for widespread access to AI technologies. We hold the conviction that all businesses should have the opportunity to develop their own language models and maintain ownership of their data and the resulting models. In the manufacturing and energy sectors, unique processes are vital for staying ahead in a competitive landscape and for boosting operational efficiency. These proprietary methods are often kept confidential as trade secrets, a strategy that protects competitive advantages rather than sharing them through patents or academic work. Unlike many existing language models that require relinquishing proprietary insights, we support a model that safeguards a company's exclusive knowledge and practices.
Automated Production and Quality Control: Generative AI is revolutionizing manufacturing operations by automating production processes and enhancing quality control. These AI models can analyze sensor data, identify potential issues, and recommend adjustments to optimize production, leading to increased efficiency, reduced waste, and improved product quality.
Predictive Maintenance: Generative AI is enabling manufacturers to anticipate and prevent equipment failures through predictive maintenance. By analyzing sensor data and historical maintenance records, these AI models can identify patterns and predict when equipment is likely to require maintenance, allowing organizations to proactively address issues before they disrupt production.
Supply Chain Optimization: Generative AI is transforming supply chain management in the manufacturing industry. These AI models can analyze vast amounts of data, including supplier performance, logistics, and market trends, to generate optimized supply chain strategies, improve inventory management, and enhance overall supply chain resilience.
Organization | Abstract |
---|---|
Boeing | How Boeing Uses Streaming Data to Enhance the Flight Deck and OCC Jeppesen Aero Insights monitors up to 200,000 general, business, and commercial aviation flights per day at more than 4000 airports worldwide by combining industry-leading Jeppesen aeronautical maps with live aircraft position data. The system uses Apache Spark™ structured streaming with arbitrary stateful aggregation to detect each pushback, taxi, take-off, hold, diversion, landing, and parking event. The data informs internal marketing, strategy, and engineering decisions through dashboards and ad-hoc analysis. It also makes its way to airline customers on the flight deck and in the operations control center (OCC) through Jeppesen products like FliteDeck Pro and Fleet Insight and directly with APIs available through Boeing Developer Tools. Learn how we use Unity Catalog to produce discoverable internal data products, Delta Live Tables pipelines with custom libraries to parse complex data types, and MLflow to train models and make online predictions for pilots. |
Hapag-Lloyd AG | Enhancing Audit Efficiency at Hapag-Lloyd with Generative AI Our focus is on a sophisticated solution deployed on the Databricks Data Intelligence Platform, featuring advanced Retrieval-Augmented Generation and scalable model serving. We delve into the challenges faced by Hapag-Lloyd's Corporate Audit team, especially the labor-intensive auditing and report generation processes that overshadow their substantial business value. We demonstrate how this solution significantly streamlined the workflow, reducing the time spent writing, proofreading, and approving reports. Furthermore, the implementation greatly enhanced the readability and impact of executive summaries, increasing their likelihood of board review and action. We will share insights into how Databricks' cutting-edge LLM architecture can revolutionize business processes and amplify value, while addressing challenges and underscoring tangible business benefits. |
Rolls-Royce | Cloud-Based Generative AI Supporting Preliminary Engineering Design Many engineering solutions require specialized know-how and a deep understanding of the physics mechanisms that underpin their design and operation. Concurrently, there is a growing need for enhanced design space exploration capabilities overcoming the limitations of parametric models, enabling the assessment of innovative design concepts through free-form geometry modelling approaches. This session outlines the collaborative work between Rolls-Royce and Databricks, with a primary focus on optimizing Conditional Generative Adversarial Network (cGAN) training processes. Leveraging Databricks and acquired know-how resulted in a significant reduction in runtime, approximately by a factor of 30, achieved through distributed computing for parallel hyper-parameter tuning. The integration of MLflow ensures transparency and reproducibility. Furthermore, the implementation of Unity Catalog establishes a crucial governance framework for compliance-centric industries, including aerospace. |
Media and Entertainment
Like many industries, media and entertainment organizations are in the midst of a digital revolution - from shifting consumer behavior and content overload, to evolving monetization and revenue models, to the challenges facing copyright and intellectual property protection. The growth of GenAI has been largely viewed with cautious optimism, with the industry embracing the technology, recognizing its potential to transform content creation, distribution, and monetization. Meanwhile, also addressing the significant challenges posed to content quality, customer trust, and balancing the critical role humans play.
Automated Content Generation: Generative AI is enabling media and entertainment companies to streamline content creation processes. These AI models can generate original scripts, articles, and even music compositions, freeing up human creators to focus on more complex and creative tasks.
Personalized Content Recommendations: Generative AI is revolutionizing the way media and entertainment companies engage with their audiences. By analyzing user preferences, viewing habits, and content metadata, these AI models can generate personalized content recommendations, enhancing user experience and increasing customer loyalty.
Customer Experience: The promise of an LLM is that you no longer need a 'preference center'. We are moving from rules-based to behavior-oriented, a next-generation preference center. There is no need to ask a user what they like when you look at what they're engaged in. We are able to understand intent per user at scale and translate that into a customer experience.
Organization | Abstract |
---|---|
SEGA | Crafting Worlds: Enhancing Creative Storyboarding with AI at SEGA The rapid integration of artificial intelligence has made an important impact on video game development and publishing. SEGA Europe is a publisher that collaborates with several studios worldwide including Sports Interactive, Creative Assembly, Amplitude Studios, Relic Entertainment, and Two Point Studios, to provide entertainment and memorable experiences through video games. SEGA has been prominent in embracing this technological revolution, and AI now plays a crucial role in all aspects of the business. This session aims to shed light on the significant strides made by SEGA Europe in the data science space, primarily focusing on the role of generative AI within creative processes. It will cover the business need and challenge to be solved, the collection and annotation of image data for video game characters, techniques to fine-tune diffusion models to the art direction and game concepts, and how to serve this application on Databricks. |
Fox | GenAI-Powered Media Dynamics: Leveraging LLMs for Personalized Content Engagement Fox is a media powerhouse with a vast library of articles, videos, and images. Through the Databricks fine-tuning API, they have trained a number of custom LLMs with distinctive style and tone, unlocking a multitude of Gen AI applications. Moreover, Fox innovates in the extraction of insights from video transcription data, identifying entities and topics from segments. This advancement facilitates marker integration for seamless VOD playback and semantic search, enhancing user experiences with curated video libraries and personalized recommendations. Fox is at the forefront of revolutionizing media interaction, ushering in a new era of immersive digital engagement. |
Vivvix | Scaling Video Ad Classification Across Millions of Classes with GenAI Vivvix, an ad-intel company, leverages real-time insights from diverse creatives (videos, audio) leveraging ML and GenAI. Our initial goal aimed to categorize video ads into 30,000 product classes, with a planned expansion to six million. While an initial transformer-based machine learning model achieved high accuracy, we anticipated challenges from exponential growth in training time and limited data per class. To address these issues, we leveraged OSS LLMs. We used optimized LLama2(Vllm) to categorize creatives by identifying product categories and conducting similarity searches across the labels. Our baseline machine learning model achieved 69% accuracy with ~ 25,000 labels and a training dataset of ~200k creatives. By integrating LLMs, we achieved a remarkable 15% uplift in accuracy. Combining both approaches, we devised a solution where the LLM model acts as a pre-processing step, generating summaries for subsequent machine learning analysis. |
Public Sector
Between defense and intelligence, transportation and infrastructure support, financial and civilian services, and healthcare and education, the public sector has its hands full in determining how best to implement GenAI applications in a safe and effective manner. As we've discussed, few technologies have had the power to disrupt like GenAI, from how interactions with citizens, to the delivery of services, through to how internal processes are managed, the public sector is taking a cautious - but necessary - look at the implementation of this technology. Databricks is supporting government organizations to bring GenAI to life through foundational use cases, such as the automation of repetitive tasks, enhancing data analysis, and supporting citizen engagement. Here are a few notable examples:
Intelligent Policy Development: Generative AI is empowering public sector organizations to develop more informed and effective policies. By analyzing vast amounts of data, including demographic information, economic indicators, and social trends, these AI models can generate policy recommendations that address the unique needs and challenges of different communities. In the field of sustainable investing, The International Finance Corporation (IFC) offers MALENA, the World Bank Group's first AI service, to external users, to support Environmental, Social, and Governance (ESG) integration in emerging markets.
Automated Citizen and Constituent Services: Generative AI-powered chatbots and virtual assistants are revolutionizing the way public sector organizations deliver services in various areas, from delivering a personalized student experience to providing a more efficient pathway to citizenship. These intelligent agents can handle a wide range of inquiries, provide instant responses, and direct users to the appropriate resources, improving the overall constituent experience and increasing efficiencies.
Enhanced Mission Delivery: Generative AI is being leveraged in the public sector to improve mission delivery in various government agencies, with missions ranging from defense and intelligence to healthcare and citizen service. Common use cases include document retrieval and summarization, automated entity resolution, streamlined logistics, and intelligence gathering.
By analyzing historical data, real-time information, and other relevant data sources, AI models in the public sector can predict potential threats, identify high-risk areas, and recommend proactive measures to mitigate risks.
Organization | Abstract |
---|---|
National Institutes of Health (NIH) | Layered Intelligence: Generative AI Meets Classical Decision Sciences In this talk, we'll explore how Generative AI, especially Large Language Models (LLMs), integrate into classical decision science methodologies. We'll see how LLMs extend beyond chatbots to enhance optimization algorithms, statistical models, and graph analytics. This layered approach brings a new edge to traditional methods, allowing for complex problem-solving, nuanced data interaction, and improved interpretability. The session will cover the methods and architectures, along with real-world applications and demo implementations. We'll discuss how AI LLMs can breathe new life into decision sciences, advancing strategic analytics and decision-making. |
Ordnace Survey | The AI of Where: Unleashing the Power of GenerativeAI on Geospatial Data In this session, we will explore cutting-edge GenAI applications in location intelligence, offering a unique perspective on the evolution of spatial data analysis to enhance Ordnance Survey's ability to deliver new insights for Great Britain. Discover innovative uses of Large Language Models and the Segment Anything Model in unlocking geospatial dataset potential. Learn how advanced AI techniques automate real-world feature extraction from diverse earth observation data. Real-world examples and case studies illustrate generative AI's effectiveness in solving complex challenges, showcasing Ordnance Survey and Databricks' groundbreaking contributions to geospatial intelligence. Delve into the pivotal role of grid index systems in scaling earth observation and geospatial datasets, optimizing AI model applications. Understand how these systems efficiently manage large-scale geospatial data, seamlessly integrating with generative AI for enhanced analytical use cases at OS. |
North Dakota University System | Unleashing the Potential of Unstructured Data with LLMs and Databricks Unstructured data can provide valuable insights for decision-making and problem-solving in various domains. However, extracting and analyzing such data can be challenging due to its complexity and diversity. This presentation will highlight the Retrieval augmented generation (RAG) architecture, an LLM framework the North Dakota University System (NDUS) has used for leveraging unstructured data, including policies and procedures, using the native AI features within Databricks. We will demonstrate how Databricks has been critical in creating an AI portal for all NDUS staff, faculty, and students to access their approved AI apps. We will also discuss the benefits and challenges of using the RAG architecture and Azure Databricks for unstructured data analytics and generation. |
Retail and Consumer Goods
Retail and consumer goods companies (RCG) can no longer afford to ignore the transformative potential of generative AI, which is poised to revolutionize every aspect of operations, from product development to customer experience. Failing to adopt this technology risks falling behind competitors and missing out on the substantial economic benefits it offers - estimated to range from $2.6 trillion to $4.4 trillion annually across various industries. Here are just a few of the core use cases driving generative AI applications across RCG:
Personalized Product Recommendations: Generative AI is transforming the retail and consumer goods industries by enabling personalized product recommendations. These AI models can analyze customer data, purchase history, and browsing behavior to suggest products that are tailored to individual preferences, — enhancing user engagement, increasing sales and fostering long-term customer loyalty.
Automated Inventory Management and Demand Forecasting: Generative AI is revolutionizing inventory management and demand forecasting in the retail and consumer goods sectors. By analyzing a wide range of data — sales data, supply chain insights, and market trends — enterprises can leverage AI models to predict demand, optimize inventory levels, and automate replenishment. With these insights, alignment between supply and demand dynamics are solidified to improve operational efficiency, avoid stock-outs, inform marketing strategies and lower costs.
Boosting Front-Line Worker Productivity and Effectiveness: The advent of generative AI is ushering in a new era of productivity and strategic decision-making on the front lines of retail, customer service, and consumer goods. Retailers, Consumer Goods companies, and Restaurants are embracing AI to streamline various facets of their operations, ranging from store associates managing customer inquiries to enhancing product quality through actionable insights for managers. Through AI, companies can optimize a spectrum of activities, from extracting image metadata to create cohesive product descriptions for e-commerce listings to elevating customer service through AI-powered chatbots. AI also empowers store managers to help their staff efficiently handle time-sensitive situations, and for shop floor managers to know what decisions to make and when to make them. These advancements underscore the essential role of generative AI in refining front-line operations, deepening customer engagement, and driving revenue growth.
Organization | Abstract |
---|---|
CVS Health | Building the World's Largest RAG for Knowledge Management at CVS Health CVS Health has 300,000+ employees and a diverse portfolio of businesses. Managing knowledge across a complex organization is challenging when knowledge is spread across various sources used by thousands of teams. Finding info you need to perform work can be a challenge in a big company. How do we ensure our employees can access the most relevant/reliable information at the right time/place? We set out to solve the knowledge management problem and improve the experience at CVS Health. We'll highlight our approach to building the world's largest RAG system for knowledge management. Many RAG systems have been POCs that fail to scale. We will describe how we use RAG to create a unified and scalable knowledge platform that serves many use cases and BUs, sharing tech and org challenges we faced, how we overcame them, and strategies to tackle this massive problem. This talk aims to inspire and inform other practitioners and researchers interested in applying RAG at scale to their domains and problems. |
Sephora | Prompt Engineering is Dead; Build LLM Applications with DSPy Framework Stop prompt engineering in LangChain. You wouldn't hand-select weights of your neural network, so don't hand-select your prompts. DSPy is an open-source framework that provides a paradigm shift towards building pipelines to optimize language model prompts, model tuning, and LLM applications with code. In this session executives will learn how adopting DSPy can save time and resources while enhancing application performance, and developers will leave equipped with knowledge on how they can incorporate DSPy into their LLM application development process. We will demonstrate how to move away from traditional prompt engineering to a more systematic approach - stitching together DSPy's "signatures, modules, and optimizers" functionality to create a system that leverages language models for specific tasks within your application – with the goal of empirically optimizing your LLM application's performance. |
To find even more customers using our AI tools to build solutions across their organization, visit our Databricks customer page or tune into the Data + AI Summit.