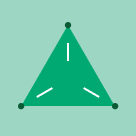
Introducing Python User-Defined Table Functions (UDTFs)
Apache Spark™ 3.5 and Databricks Runtime 14.0 have brought an exciting feature to the table: Python user-defined table functions (UDTFs). In this blog post, we’ll dive into what UDTFs are, why they are powerful, and how you can use them.
What are Python user-defined table functions (UDTFs)
A Python user-defined table function (UDTF) is a new kind of function that returns a table as output instead of a single scalar result value. Once registered, they can appear in the FROM
clause of a SQL query.
Each Python UDTF accepts zero or more arguments, where each argument can be a constant scalar value such as an integer or string. The body of the function can inspect the values of these arguments in order to make decisions about what data to return.
Why should you use Python UDTFs
In short, if you want a function that generates multiple rows and columns, and want to leverage the rich Python ecosystem, Python UDTFs are for you.
Python UDTFs vs Python UDFs
While Python UDFs in Spark are designed to each accept zero or more scalar values as input, and return a single value as output, UDTFs offer more flexibility. They can return multiple rows and columns, extending the capabilities of UDFs.
Python UDTFs vs SQL UDTFs
SQL UDTFs are efficient and versatile, but Python offers a richer set of libraries and tools. For transformations or computations needing advanced techniques (like statistical functions or machine learning inferences), Python stands out.
How to create a Python UDTF
Let’s look at a basic Python UDTF:
from pyspark.sql.functions import udtf
@udtf(returnType="num: int, squared: int")
class SquareNumbers:
def eval(self, start: int, end: int):
for num in range(start, end + 1):
yield (num, num * num)
In the above code, we've created a simple UDTF that takes two integers as inputs and produces two columns as output: the original number and its square.
The first step to implement a UDTF is to define a class, in this case
class SquareNumbers:
Next, you need to implement the eval
method of the UDTF. This is the method that does the computations and returns rows, where you define the input arguments of the function.
def eval(self, start: int, end: int):
for num in range(start, end + 1):
yield (num, num * num)
Note the use of the yield
statement; A Python UDTF requires the return type to be either a tuple or a Row
object so that the results can be processed properly.
Finally, to mark the class as a UDTF, you can use the @udtf
decorator and define the return type of the UDTF. Note the return type must be a StructType with block-formatting or DDL string representing a StructType with block-formatting in Spark.
@udtf(returnType="num: int, squared: int")
How to use a Python UDTF
In Python
You can invoke a UDTF directly using the class name.
from pyspark.sql.functions import lit
SquareNumbers(lit(1), lit(3)).show()
+---+-------+
|num|squared|
+---+-------+
| 1| 1|
| 2| 4|
| 3| 9|
+---+-------+
In SQL
First, register the Python UDTF:
spark.udtf.register("square_numbers", SquareNumbers)
Then you can use it in SQL as a table-valued function in the FROM clause of a query:
spark.sql("SELECT * FROM square_numbers(1, 3)").show()
+---+-------+
|num|squared|
+---+-------+
| 1| 1|
| 2| 4|
| 3| 9|
+---+-------+
Arrow-optimized Python UDTFs
Apache Arrow is an in-memory columnar data format that allows for efficient data transfers between Java and Python processes. It can significantly boost performance when the UDTF outputs many rows. Arrow-optimization can be enabled using useArrow=True
.
from pyspark.sql.functions import lit, udtf
@udtf(returnType="num: int, squared: int", useArrow=True)
class SquareNumbers:
...
Real-World Use Case with LangChain
The example above might feel basic. Let’s dive deeper with a fun example, integrating Python UDTFs with LangChain.
from langchain.chains import LLMChain
from langchain.llms import OpenAI
from langchain.prompts import PromptTemplate
from pyspark.sql.functions import lit, udtf
@udtf(returnType="keyword: string")
class KeywordsGenerator:
"""
Generate a list of comma separated keywords about a topic using an LLM.
Output only the keywords.
"""
def __init__(self):
llm = OpenAI(model_name="gpt-4", openai_api_key=<your-key>)
prompt = PromptTemplate(
input_variables=["topic"],
template="generate a couple of comma separated keywords about {topic}. Output only the keywords."
)
self.chain = LLMChain(llm=llm, prompt=prompt)
def eval(self, topic: str):
response = self.chain.run(topic)
keywords = [keyword.strip() for keyword in response.split(",")]
for keyword in keywords:
yield (keyword, )
Now, you can invoke the UDTF:
KeywordsGenerator(lit("apache spark")).show(truncate=False)
+-------------------+
|keyword |
+-------------------+
|Big Data |
|Data Processing |
|In-memory Computing|
|Real-Time Analysis |
|Machine Learning |
|Graph Processing |
|Scalability |
|Fault Tolerance |
|RDD |
|Datasets |
|DataFrames |
|Spark Streaming |
|Spark SQL |
|MLlib |
+-------------------+
Get Started with Python UDTFs Today
Whether you're looking to perform complex data transformations, enrich your datasets, or simply explore new ways to analyze your data, Python UDTFs are a valuable addition to your toolkit. Try this notebook and see the documentation for more information.
Future Work
This functionality is only the beginning of the Python UDTF platform. Many more features are currently in development in Apache Spark to become available in future releases. For example, it will become possible to support:
- A polymorphic analysis wherein UDTF calls may dynamically compute their output schemas in response to the specific arguments provided for each call (including the types of provided input arguments and the values of any literal scalar arguments).
- Passing entire input relations to UDTF calls in the SQL FROM clause using the TABLE keyword. This will work with direct catalog table references as well as arbitrary table subqueries. It will be possible to specify custom partitioning of the input table in each query to define which subsets of rows of the input table will be consumed by the same instance of the UDTF class in the eval method.
- Performing arbitrary initialization for any UDTF call just once at query scheduling time and propagating that state to all future class instances for future consumption. This means that the UDTF output table schema returned by the initial static "analyze" method will be consumable by all future __init__ calls for the same query.
- Many more interesting features!