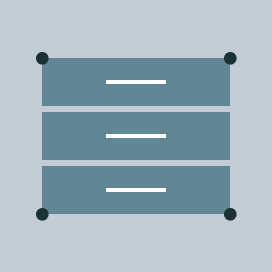
Databricks’ mission is to “democratize access to data analytics and AI.” Not only does that statement give meaning to the everyday work of data professionals, but it is also relevant — reflecting the state of today’s data and AI space because scaling data and AI is hard. Multiple independent surveys and research notes from the likes of McKinsey, Deloitte and Accenture point to the same conclusion: while data and AI demand and interest is at an all-time high, most companies are struggling to achieve enterprise value for data and AI at scale.
One such study is the 2022 Accenture report called “The art of AI maturity”, which showed that only 12% of the 1,200 companies surveyed are realizing a strong competitive advantage and could call themselves data and AI achievers. That’s 88% of them leaving the full value of data and AI untapped.
Challenges enterprises face in achieving enterprise value for data and AI at scale
- Modernizing legacy architectures built via organic growth to support evolving business priorities
- “Keeping the lights on” with disjointed tooling and siloed infrastructure taking too much time and effort
- Lack of talent to operationalize data + AI initiatives
- Inability to quickly leverage new products, services and better customer experience to unlock potential revenue
- Harnessing the pace of change in the technology landscape (e.g., Generative AI) to gain a competitive advantage
Enterprises need to steer the best path forward in managing the people, process and technology aspects of transformation as a whole to maximize value from data and AI investment. In this blog, we’ll walk you through how Databricks have helped many customers in this journey.
4 Tenets of a successful data and AI Business
We’ve been working with the world’s top enterprises to help them solve their toughest data and AI problems on a massive scale. Drawing upon these experiences and lessons learned over the last 10 years, we’ve formed our point of view and methodology for how we can optimally help customers build their data and AI practice at scale. Seeing hundreds of customers embark on the lakehouse journey, we saw a pattern exhibited by the ones that are most successful — the true game changers — in how they manage the following four areas in what we refer to as the four tenets of a successful data and AI business.
In each of the four tenets, Databricks have partnered with customers with the following end goals in mind.
The key organizational construct that is found in these data-native companies is the creation of a center of excellence (CoE) that is designed to establish in-house expertise around ML and AI, and which is then used to educate and scale the rest of the organization on their data and AI practice embodied by the four tenets. It does so by bringing different stakeholders together, providing the right expertise to business units, tracking key projects, helping them move faster, and sharing best practices.
Creation of CoE and Its Building Blocks
These companies take the stand that building CoE capabilities is not just a one-time exercise. Successful customers treat it as a journey, going through different phases as laid out below in “Establish, Scale and Autonomy.” So the following figure represents the “What” of the Lakehouse CoE framework at a high level and provides a summary view of the key CoE capabilities customers should build and validate along their journey. This represents what a “good” looks like and how customers get there through different phases as they mature.
Each red rectangle highlighted above represents CoE milestones. For example, for the “Data & AI Blueprint” tenet, during the “ESTABLISH” phase, customers should build and document robust data models and governance along with the adoption of a well-architected Lakehouse. You need such a blueprint established at this early stage to inform your downstream activities in how you build your data products and applications and in how you run your platform optimally aligned to meet your business objectives. In the “SCALE” phase, then you apply the outcome of the ESTABLISH phase to help business units scale their key business initiatives and day-to-day data activities. For example, with the “Integrate DevOps Practices” milestone for the “Lakehouse Operations” tenet, customers should fully adopt CI/CD in their development practices for developing data products that can be leveraged and reused by other business units.
These milestones serve as CoE building blocks with their supporting work breakdown structure and effort required informed by work that has already been done, validated with our experts and mutually agreeing on the right level of help customers need. This approach along with an assessment of the customer's maturity helps Databricks and the customer put together a comprehensive success plan/services roadmap that addresses both short-term needs balanced with long-term data and AI vision. What it really comes down to in measuring success in this endeavor is based on customers developing robust CoE capabilities with self-sufficiency in managing their data and AI practice at scale.
Building a strong data and AI culture
While we’ve been largely talking about the Lakehouse CoE framework and approach, it’s equally important for customers to consider how they should organize their people and process for scale: customers need to build a strong data and AI culture.
To tie together all of the points above, you need to create a Lakehouse Center of Excellence, which will consolidate cross-functional proficiency in digital technologies such as AI and IoT by bringing different stakeholders together, prioritizing and tracking projects, helping them move faster, sharing with the rest of the organization best practices gleaned from business units within and what Databricks is seeing in the industry — along with talent transformation driving upskilling through data and AI education.
Organizing and running the CoE
So if this idea makes sense, in what manner should customers organize and run the CoE? CoE operating models can take on different flavors such as a centralized or distributed approach. Some customers have taken the distributed approach further by leveraging data mesh architecture by organizing data and data products by specific business domains.
A centralized model is shown below, where a central, shared team supports use cases across the organization. Key benefits include the relative ease of developing and governing processes, consistent definitions and use of KPIs, and manageable effort in establishing a single source of truth. While it may not fit everyone, if you are getting started with CoE, this might be a good option to explore further.
Success Stories
So where have we done this? Let’s highlight some of the representative engagements where the Lakehouse CoE partnership with customers has made a meaningful impact.
We’ll cover the first example from the table below. For this multinational investment bank and financial services company, Databricks has partnered with them across four tenets over three years. Toward the middle of the engagement, we observed plateauing of platform usage uptake due to a lack of skills in using the platform. We worked with the customer to help define a comprehensive enablement strategy. In addition to offering customer-tailored training, we defined learning pathways for utilizing self-paced training leading to certification goals integrated as part of their personal development in support of their Certified Engineer and Engineering Excellence initiatives.
Now we have 1,800+ upskilled users and 700+ badges with around 350 in the last 6 months where these users are using the platform to get faster insight into managing their day-to-day activities. In addition, we collaborated in building Data & AI blueprints, focused on use case accelerators to help define reusable components and publish them on an internal portal for consumption across business units. This portal also curates contents and links to the training, recordings from customer user community events and other sources, making it accessible and scalable in a self-service manner. Databricks Professional Services has been partnering with business units as a multi-skilled team to drive optimizations and cost savings in the Lakehouse Operations tenet. These close partnerships have resulted in Databricks being attributed to a $715M three-year value forecast.
These CoE engagements demonstrate how customers across different industries were able to reduce TCO, drive efficiency and scale, and accelerate their business outcomes.
Customer benefits and value realization
- Increased productivity and faster time to insight and market
- Reduced risk resulting from better governance and transparency
- Robust throughput as the organization attracts, maintains and develops talent
- Reduced TCO through reusable blueprints and best practices
- New AI use cases unlocked
- Analytical workloads well-architected and aligned to business benefit
Tiering and schedule
At its core, the Lakehouse CoE engagement is made up of 3 components:
- Professional Services co-delivering with C&SI partners
- Databricks expert coach
- Learning and enablement
These components are used at varying levels of engagement reflecting customer's needs, summarized in the image below.
In closing, Lakehouse CoE is a proven delivery framework and methodology that has been hardened by helping many customers solve their toughest data and AI problems at massive scale. Let us know how we can help you accelerate scaling your data and AI practice.
What’s Next?
We invite readers of this blog whether you are a data engineer, data scientist, analyst, or business/IT leader such as CIO, CDO and CTO to engage with us in discovering how we can partner with you to achieve enterprise value for data and AI at scale. We can be reached at [email protected].
We also encourage you to check out the Databricks Professional Services page to learn more.