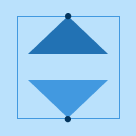
In the two decades since the completion of the first draft of the human genome, the landscape of biological research has undergone a revolutionary transformation. The field of genomics has expanded exponentially, giving rise to a broader "omics" revolution, encompassing diverse data types such as single-cell RNA sequencing, proteomics, and metabolomics to name a few.
These cutting-edge technologies are providing unprecedented insights into biological functions at the most granular level, offering a deeper understanding of disease mechanisms, organism adaptations, and interactions with environmental factors, including drugs and chemicals. The implications of this omics explosion are far-reaching, promising to revolutionize drug discovery, precision medicine, agriculture, and biomanufacturing.
However, the majority of life sciences organizations struggle to fully unlock these insights, due to a variety of challenges posed by the existing data infrastructure and technologies used. To overcome these challenges, modernizing data platforms is crucial for the successful application of multi-omics in research and development.
In this blog we explore how new technologies such as Databricks Data Intelligence Platform can address these issues, paving the way for more effective and efficient multi-omics data management.
Most organizations struggle to tap into this data due to legacy architecture
Legacy data infrastructures struggle to manage the complexities of multiomics data, particularly in providing a scalable solution for data integration and analyzing these massive datasets. Furthermore, they lack native support for advanced analytics and the rising demand for AI.
Issues such as data interoperability, accessibility, and reusability are common, exacerbated by the lack of standardization across siloed omics platforms. To make this even more complex, organizations must balance data accessibility with patient privacy and regulatory compliance in a highly regulated environment.
Key data challenges facing life sciences organizations
How are organizations currently addressing these issues? Today, most employ a range of technologies concurrently to handle omics data. This strategy, however, presents several challenges, including:
Data Volume and Complexity
Omics data is both vast and highly complex, requiring advanced computational methods for analysis. For example, with the rise of advanced deep learning methods for multi-omics data integration, the high dimensionality of these datasets can introduce significant "noise," making it difficult to derive actionable insights. In particular, the High-Dimensional Low-Sample-Size (HDLSS) problem is challenging in omics research, where the risk of overfitting in machine learning (ML) models can reduce the generalizability of findings. Addressing this issue requires robust data preprocessing and advanced computational techniques, that many legacy data infrastructures are not designed to handle.
Standardization and Interoperability
The absence of common standards across different omics platforms presents significant challenges in ensuring data interoperability and reusability. Without standardized protocols, integrating diverse datasets into a cohesive framework becomes an arduous task.
Regulatory Considerations
Ensuring that omics data are accessible while maintaining patient privacy and adhering to regulations such as HIPAA and GDPR is a complex balancing act. This challenge is heightened in a global research environment where data is often shared across different jurisdictions. In addition, as more genetics data are being used in diagnostic settings or for training machine learning models for predicting disease risk (such as polygenic risk scoring), the ability to track all aspects of the training process—from data acquisition and quality control to model training and explainability—has become increasingly critical.
User Experience
The pharmaceutical industry benefits from access to a diverse range of professionals, including IT specialists, data scientists, medical researchers, and bench scientists conducting complex experiments on various biological samples. Most existing data platforms, built on different technologies—spanning High-Performance Computing (HPC), traditional data warehouses and different native cloud services—require significant technical maintenance to adapt to the rapidly evolving landscape of omics data.
Moreover, access to insights by non-technical team members with domain knowledge is hindered due to the complexity of these systems and the steep learning curve associated with their use. This challenge creates a significant barrier to effective collaboration and data-driven decision-making within life sciences organizations.
Rise of GenAI Applications
Training new foundation models using multi-omics data is revolutionizing biomedical research and drug discovery. For example, with the rise of single-cell omics data, models like scGPT and Geneformer leverage large-scale multi-omics datasets to predict drug responses and identify new therapeutic targets, driving advancements in personalized medicine. Companies such as EvolutionaryScale and Profulent.bio have trained large language models (LLMs) for generating new synthetic proteins based on multiomics data. However, operationalizing these models presents significant challenges, particularly in terms of training efficiency and cost-effectiveness. The computational demands of processing vast datasets require advanced infrastructure, that can handle both data management and cost-effective training of such large models on massive amounts of multi-modal data.
Introducing the Databricks Data Intelligence Platform for Omics
The Databricks Data Intelligence Platform offers a powerful foundation for a multi-omics data platform, effectively addressing the complexities that researchers and IT professionals encounter when managing omics data. Here's how Databricks can help overcome each of the key challenges:
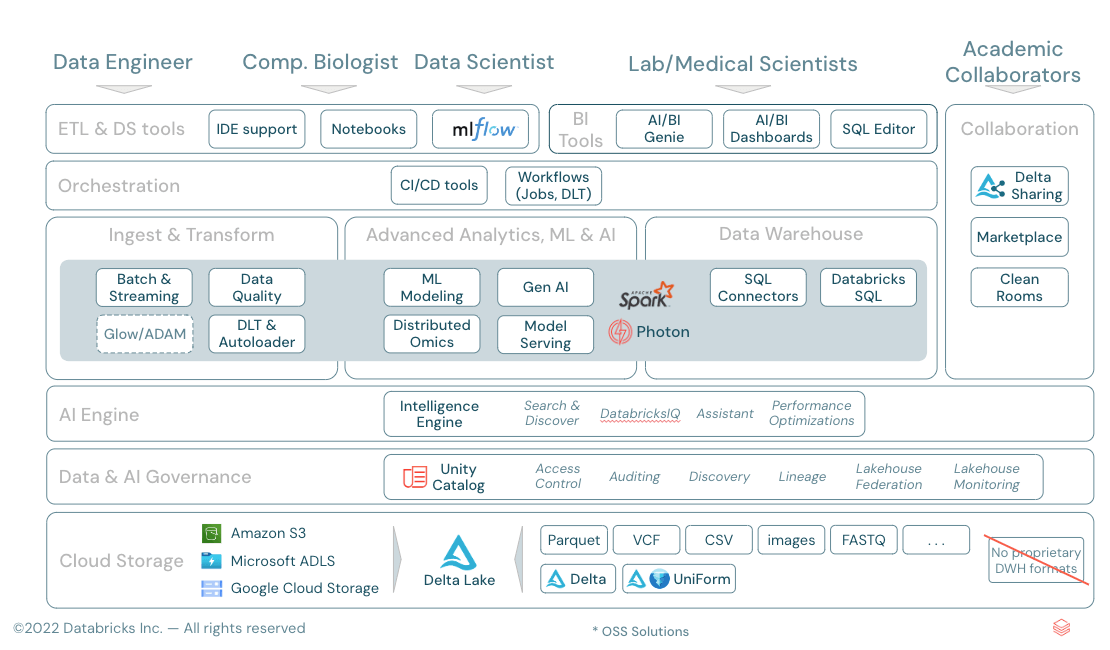
Data Volume and Complexity
Databricks is built on a scalable cloud infrastructure that can handle the vast and complex datasets typical of omics research. With its integration with Apache Spark and a high-performance compute engine powered by Photon, Databricks enables cost-effective distributed data processing. Additionally, by having the ML/AI stack built on top of a powerful data management infrastructure, it reduces the friction of managing separate tech stacks for data management and advanced analytics while accelerating time to value.
The Databricks Photon engine provides a significant boost to Spark-based genomic pipelines and tools such as Project Glow, accelerating and simplifying the analysis of large genomic datasets, particularly for genetic target identification via Genome-Wide Association Studies (GWAS).
Standardization and Interoperability
The Databricks lakehouse architecture enables seamless interoperability by integrating unstructured, semi-structured, and structured data from data lakes and data warehouses into a single, unified platform based on open-source technologies such as Delta Lake and Unity Catalog. This approach facilitates the integration of diverse datasets, supporting open data formats and interfaces to reduce vendor lock-in and simplify data integration across different systems.
By leveraging open-source technologies and providing a centralized data catalog, Unity Catalog, Databricks ensures that data is easily discoverable, accessible, and can be integrated with external systems in a compliant and auditable manner. This enables researchers to deliver on the FAIR principles (Findability, Accessibility, Interoperability, and Reusability) for scientific data management, promoting collaboration, reproducibility, and data-driven insights.
Regulatory Considerations
Databricks Unity Catalog enables organizations to meet stringent regulatory requirements, such as HIPAA and GDPR, while enhancing data findability and accessibility. With its centralized metadata repository and powerful semantic search capabilities, users can quickly locate relevant data assets based on context and meaning. The platform's fine-grained access controls, identity federation, and comprehensive audit logging ensure data security and compliance.
Additionally, Unity Catalog provides advanced metadata management, tagging, and data lineage tracking to enhance the discoverability and reproducibility of experiments. To further ensure regulatory compliance, Databricks offers robust data encryption and secret management features. The platform also integrates open-source technologies, such as the Delta Sharing Protocol, which enables secure data sharing between parties. Databricks Clean Rooms facilitates secure collaboration among researchers from different organizations while meeting data residency requirements.
These capabilities collectively enable organizations to uphold strict data protection standards while allowing authorized users to efficiently discover, access, and share necessary data for analysis and research in a secure, compliant environment—even across organizational boundaries.
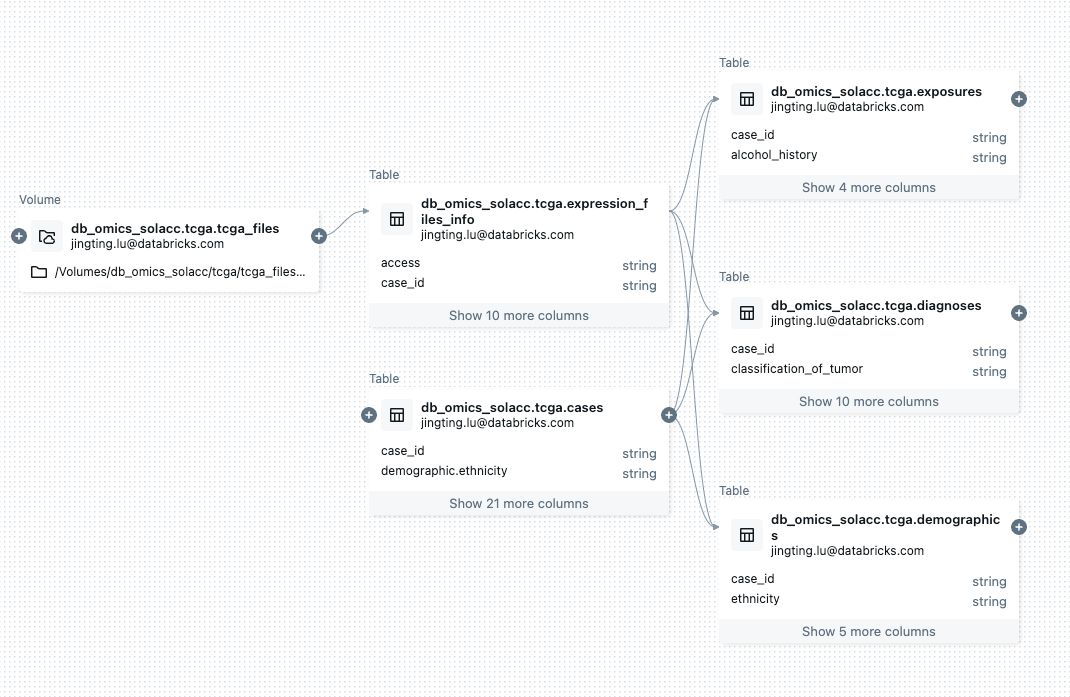
User Experience
Databricks offers a comprehensive, self-service data platform that simplifies infrastructure management and integrates various data types. Its user-friendly interfaces, featuring natural language querying and context-aware AI-powered assistance, enable straightforward data access and analysis. This approach demystifies data interactions, making the platform accessible not only to technical users but also to domain experts without a technical background.
By simplifying data access and reducing IT overhead while enhancing collaboration among different teams, Databricks accelerates decision-making and innovation in drug discovery and development.
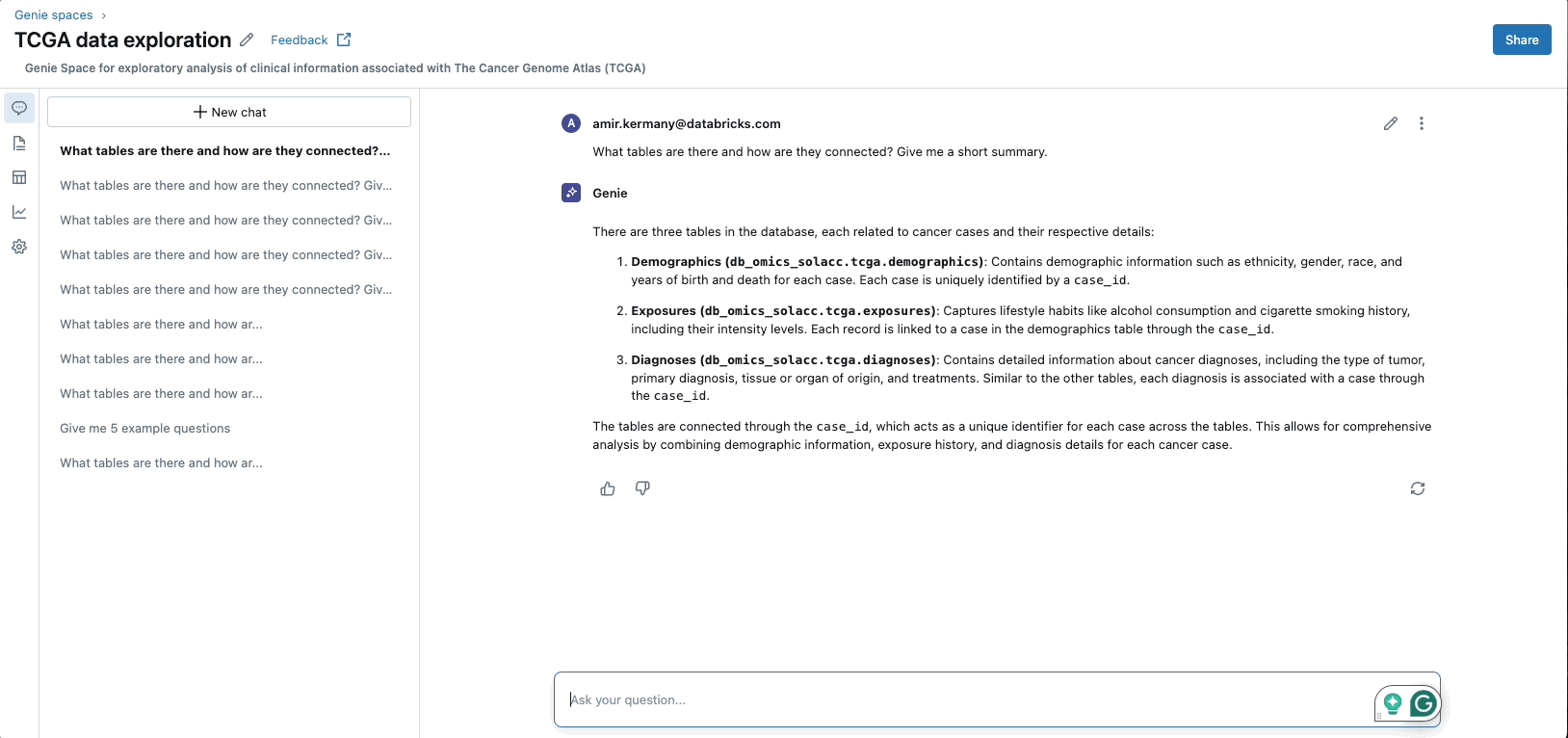
Rise of GenAI Applications
Databricks’ MosaicAI platform enables the pre-training, fine-tuning, and deployment of generative AI models by providing a scalable and secure computational infrastructure. With MosaicAI, Databricks offers solutions specifically designed for cost-effective training of foundation models on an organization's proprietary datasets. Additionally, MosaicAI offers highly scalable vector search and an AI Agent Framework for building compound AI systems, along with LLMOps/MLOps capabilities for managing the entire lifecycle of AI models.
This ensures that they are operationalized effectively, efficiently, and at scale, allowing organizations to unlock the full potential of generative AI and drive business value from their AI investments.
Looking ahead
In the upcoming technical blogs, we will explore the use of Databricks technologies for multi-omics. This will include running Genome-Wide Association Studies and pre-training the Geneformer foundation model with MosaicAI.
In summary, Databricks offers a comprehensive platform that addresses the various challenges of managing omics data. With its scalable infrastructure, support for interoperability, strong security features, and advanced AI capabilities, Databricks enables pharmaceutical companies to extract practical insights from complex omics datasets. By utilizing Databricks, organizations can expedite their research and development (R&D) efforts, leading to innovation and improved patient outcomes.
Learn more about our data and AI solutions for healthcare and life sciences.