Migrating to the cloud to better serve millions of customers
ROI from OpEx savings and cost avoidance
Faster delivery of ML/data science use cases
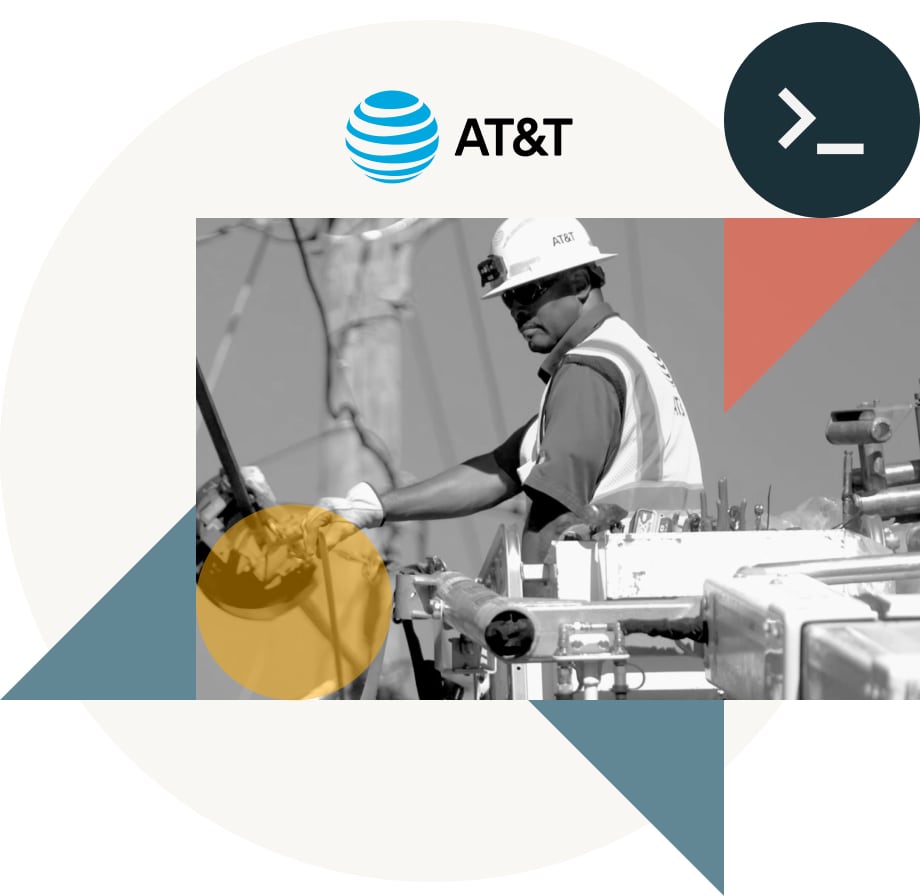
“The migration from Hadoop to Databricks enables us to bring more value to our customers and do it more cost-efficiently and much faster than before.”
Consistency in innovation is what keeps customers with a telecommunications company and is why AT&T is ranked among the best. However, AT&T’s massive on-premises legacy Hadoop system proved complex and costly to manage, impeding operational agility and efficiency and engineering resources. The need to pivot to cloud to better support hundreds of millions of subscribers was apparent. Migrating from Hadoop to Databricks on the Azure cloud, AT&T experienced significant savings in operating costs. Additionally, the new cloud-based environment has unlocked access to petabytes of data for correlative analytics and an AI-as-a-Service offering for 2,500+ users across 60+ business units. AT&T can now leverage all its data — without overburdening its engineering team or exploding operational costs — to deliver new features and innovations to its millions of end users.
Hadoop technology adds operational complexity and unnecessary costs
AT&T is a technology giant with hundreds of millions of subscribers and ingests 10+ petabytes[a] of data across the entire data platform each day. To harness this data, it has a team of 2,500+ data users across 60+ business units to ensure the business is data powered — from building analytics to ensure decisions are based on the best data-driven situation awareness to building ML models that bring new innovations to its customers. To support these requirements, AT&T needed to democratize and establish a data single version of truth (SVOT) while simplifying infrastructure management to increase agility and lower overall costs.
However, physical infrastructure was too resource intensive. The combination of a highly complex hardware setup (12,500 data sources and 1,500+ servers) coupled with an on-premises Hadoop architecture proved complex to maintain and expensive to manage. Not only were the operational costs to support workloads high, but there were also additional capital costs around data centers, licensing and more. Up to 70% of the on-prem platform had to be prioritized to ensure 50K data pipeline jobs succeeded and met SLAs and data quality objectives. Engineers’ time was focused on managing updates, fixing performance issues or simply provisioning resources rather than focusing on higher-valued tasks. The resource constraints of physical infrastructure also drove serialization of data science activities, slowing innovation. Another hurdle faced in operationalizing petabytes of data was the challenge of building streaming data pipelines for real-time analytics, an area that was key to supporting innovative use cases required to better serve its customers.
With these deeply rooted technology issues, AT&T was not in the best position to achieve its goals of increasing its use of insights for improving its customer experience and operating more efficiently. “To truly democratize data across the business, we needed to pivot to a cloud-native technology environment,” said Mark Holcomb, Distinguished Solution Architect at AT&T “This has freed up resources that had been focused on managing our infrastructure and move them up the value chain, as well as freeing up capital for investing in growth-oriented initiatives.”
A seamless migration journey to Databricks
As part of its due diligence, AT&T ran a comprehensive cost analysis and concluded that Databricks was both the fastest and achieved the best price/performance for data pipelines and machine learning workloads. AT&T knew the migration would be a massive undertaking. As such, the team did a lot of upfront planning — they prioritized migrating their largest workloads first to immediately reduce their infrastructure footprint. They also decided to migrate their data before migrating users to ensure a smooth transition and experience for their thousands of data practitioners.
They spent a year deduplicating and synchronizing data to the cloud before migrating any users. This was a critical step in ensuring the successful migration of such a large, complex multi-tenant environment of 2,500+ users from 60+ business units and their workloads. The user migration process occurred over nine months and enabled AT&T to retire on-premises hardware in parallel with migration to accelerate savings as early as possible. Plus, due to the horizontal, scalable nature of Databricks, AT&T didn’t need to have everything in one contiguous environment. Separating data and compute, and across multiple accounts and workspaces, ensured analytics worked seamlessly without any API call limits or bandwidth issues and consumption clearly attributed to the 60+ business units.
All in all, AT&T migrated over 1,500 servers, more than 50,000 production CPUs, 12,500 data sources and 300 schemas. The entire process took about two and a half years. And it was able to manage the entire migration with the equivalent of 15 full-time internal resources. “Databricks was a valuable collaborator throughout the process,” said Holcomb. “The team worked closely with us to resolve product features and security concerns to support our migration timeline.”
Databricks reduces TCO and opens new paths to innovation
One of the immediate benefits of moving to Databricks was huge cost savings. AT&T was able to rationalize about 30% of its data by identifying and not migrating underutilized and duplicate data. And prioritizing the migration of the largest workloads allowed half the on-prem equipment to be rationalized during the course of the migration. “By prioritizing the migration of our most compute-intensive workloads to Databricks, we were able to significantly drive down costs while putting us in position to scale more efficiently moving forward,” explained Holcomb. The result is an anticipated 300% five-year migration ROI from OpEx savings and cost avoidance (e.g., not needing to refresh data center hardware).
With data readily available and the means to analyze data at any scale, teams of citizen data scientists and analysts can now spend more time innovating, instead of serializing analytics efforts or waiting on engineering to provide the necessary resources — or having data scientists spend their valuable time on less complex or less insightful analyses. Data scientists are now able to collaborate more effectively and speed up machine learning workflows so that teams can deliver value more quickly, with a 3x faster time to delivery for new data science use cases.
“Historically you would have had operations in one system and analytics in a separate one,” said Holcomb. “Now we can do more use cases like operational analytics in a platform that fosters cross-team collaboration, reduces cost and improves the consistency of answers.” Since migrating to Databricks, AT&T now has a single version of truth to create new data-driven opportunities, including a self-serve AI-as-a-Service analytics platform that will enable new revenue streams and help it continue delivering exceptional innovations to its millions of customers.