Building smarter decision intelligence for manufacturers
Circuitry.ai uses Databricks to help manufacturers optimize sales and service outcomes
Reduction in time searching for information
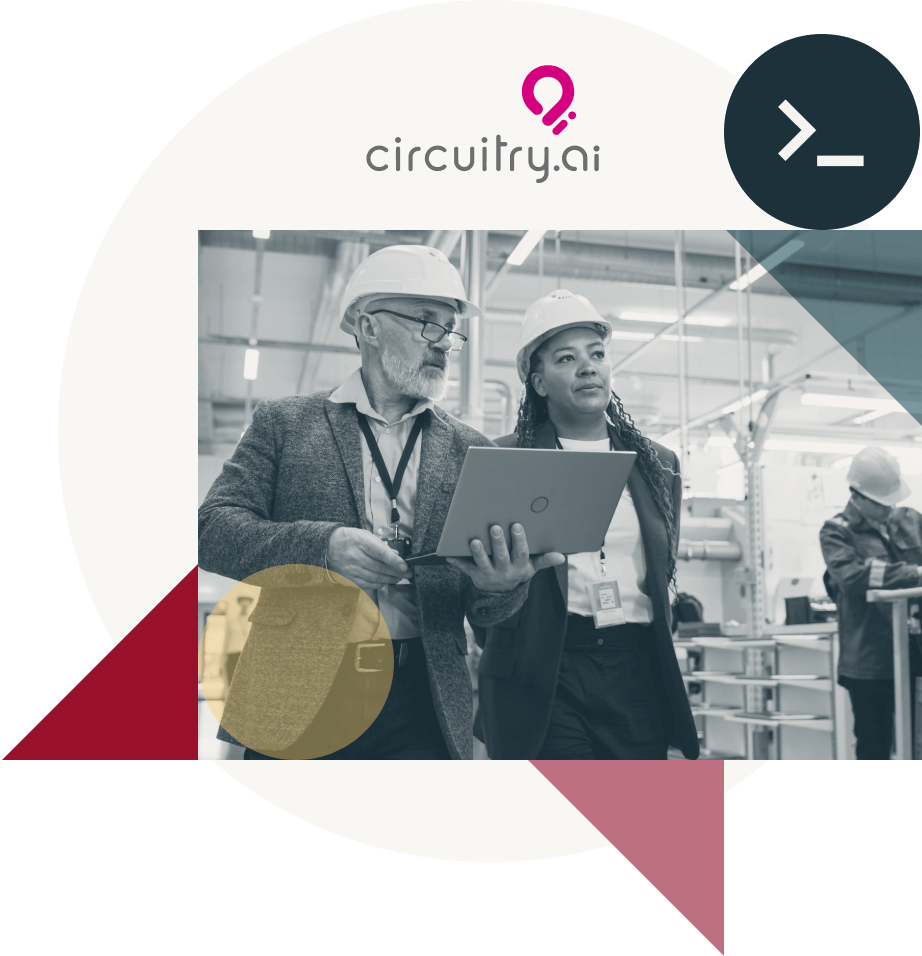
Circuitry.ai — a new company inspired by recent advancements in GenAI — aims to provide decision intelligence to manufacturers of high-value, complex products. Their mission is to help analyze, augment and automate decisions throughout the buyer lifecycle. However, their small technical team was experiencing delays in developing retrieval augmented generation (RAG) chatbots due to the complexity of managing and updating vast amounts of proprietary customer data. Partnering with Databricks, Circuitry.ai utilized a suite of features to streamline data management, governance and model training. This collaboration resulted in a 60–70% reduction in time spent searching for information, significantly enhancing operational efficiency, customer experiences and employee productivity.
Facing complex information management challenges
Circuitry.ai’s target customers — manufacturers of high-value, complex products — have a lot to manage daily. Their intricate products (think heavy equipment and automotive) come with complex bills of materials, necessitating specialized knowledge for both sales and service. For these reasons, they must disseminate product information effectively across various stakeholders, including manufacturers, sales associates, channel partners and customers. This content distribution and training process is labor-intensive, requiring significant knowledge to sell and support these complex products.
Founded in 2023 by industry leaders, Circuitry.ai recognized an opportunity to solve their clients’ most significant obstacles using GenAI tools. Before they could succeed, Circuitry.ai had to navigate several issues in developing their AI-powered product and decision support tools. First, applying metadata filters on top of retrievers had proven challenging due to insufficient documentation. Second, the team needed to establish internal checks for AI chatbots to ensure they met evaluation criteria, and they sought to improve methods for handling knowledge base updates without disrupting internal RAG chains. This meant ensuring proper data segregation to protect sensitive information and maintain the accuracy and reliability of chatbot responses. Effective data management and governance were essential for building trust with customers and enabling the seamless flow of information across various stakeholders.
Finally, the process of training these AI models to accurately handle vast and evolving datasets was challenging. Circuitry.ai’s GenAI-powered advisors needed to provide reliable, contextually accurate responses, which required sophisticated data management and continuous updates to the knowledge base. However, integrating multiple data sources complicated matters further. Each source had different structures and formats, necessitating a stable data integration framework that ensured data consistency. As noted by Ashok Kartham, Founder and CEO at Circuitry.ai, ”Most of the data that we wanted to use was actually our customer’s proprietary data. They provided their internal knowledge resources. In return, we wanted to use that specialty knowledge to train our AI solution to solve their most pressing issues.” Therefore, Circuitry.ai models had to be adaptable to new information and able to keep up with the constant addition of new products and knowledge assets. This required the implementation of a data scalable infrastructure that could support real-time data processing and updates and advanced machine learning techniques. Databricks met both of these requirements.
Improving data infrastructure for AI chatbots
Partnering with Databricks, Circuitry.ai was able to easily manage this wealth of proprietary customer data to train RAG chatbots. This approach started with Delta Lake, an open source storage layer that brings reliability to data lakes through ACID transactions and unified batch and streaming data processing. Delta Lake supported the incremental data updates needed, which were crucial for keeping the knowledge base current when new products and knowledge articles were added. This workflow alone took up much of the Circuitry.ai team’s time before launch. Alongside Delta Lake, Unity Catalog, a unified governance solution for data and AI assets, facilitated effective data management and governance, ensuring proper data segregation and security of proprietary information that was valuable to Circuitry.ai’s model training processes. MLflow and Databricks Model Serving further enabled the deployment and management of machine learning models.
To advance their AI offerings, Circuitry.ai next developed custom knowledge bases and RAG chatbots within the platform’s ecosystem. These were tailored to specific domains and use cases based on customer requirements. Customers could upload their own documents or references, and the bot could then interact with this data and respond to queries. The workflow began when customers uploaded documents via the user interface, triggering three key tasks: ingesting raw data, processing and generating embeddings and deploying the serving endpoint. Each task was supported by associated notebooks handling required inputs and dependencies from upstream tasks. Once the endpoint was ready, it was published to the application stack for customer use.
On top of this application stack, Circuitry.ai implemented a RAG pipeline to enhance the accuracy and relevance of the answers provided by their AI chatbot tools. This pipeline utilized generative AI models, specifically Llama and DBRX, which are known for their precision and reliability. Kartham explained, ”Databricks has made it easier by supporting multiple models, and we saw this as an immediate benefit — the ability to switch between models and extensively test has been a real plus for us.” Circuitry.ai then incorporated a feedback mechanism to ensure continuous improvement, where users could rate the GenAI-generated responses. For example, this feedback loop helped Circuitry.ai improve their Decision AIdvisor tool by simplifying the querying process and customized prompts for different customers and scenarios, making AI accessible even to nontechnical users.
The success of these initial projects encouraged Circuitry.ai to take advantage of metadata, along with Model Serving, a highly performant serverless vector database with governance built in. With assistance from Databricks, they could filter results and make them more geared toward individual clients — even those with a multitude of products and applications.
Revolutionizing both employee and customer experiences
Leveraging the Databricks Platform has led to substantial improvements in efficiency and productivity for Circuitry.ai. Customers of the decision intelligence software experienced a 60–70% reduction in the time spent searching for information. This efficiency gain is particularly evident in the onboarding process for their customers’ new employees, who now have easier access to relevant information. The streamlined data management and querying process allows them to become productive quickly, enables them to focus more on decision-making and customer service and enhances overall operational efficiency.
Better yet, customer feedback from proof-of-concept trials of the newest AI chatbot rollouts has been overwhelmingly positive. Circuitry.ai customers have praised the speed and relevance of the AI-driven responses, which have greatly improved their ability to find accurate information. Since the AI system reduces the need to search through large PDF files, happy customers receive answers in seconds rather than minutes. This positive reception has encouraged Circuitry.ai to plan further enhancements to their Decision AIdvisor tool, including guided sales, service recommendations and predictive analytics. These planned extensions aim to provide even more value to customers by helping them streamline their workflows in the current financial environment, where employees are expected to do more with less. Kartham concluded, “We couldn’t keep building upon this project without the Databricks team. They’ve been a tremendous help in this whole process, and our team at Circuitry.ai has experienced unwavering support since the beginning of the implementation.”
For the future, Circuitry.ai is working on handling more complex data types — such as images and schematics in manuals and videos — to provide even richer and more comprehensive information to their customers. Additionally, the company plans to integrate advanced analytics for customer lifetime value, quality control and predictive maintenance, helping manufacturers identify and address potential issues before they become significant problems. Automation for order management, service scheduling and dynamic pricing is also on the roadmap, as Circuitry.ai leverages the power of GenAI to drive greater profitability for their customers. To learn more about Circuitry.ai’s suite of AI-powered product discovery and decision intelligence tools, visit Circuitry.ai.