Crafting bespoke content experiences for every customer
in infrastructure costs savings
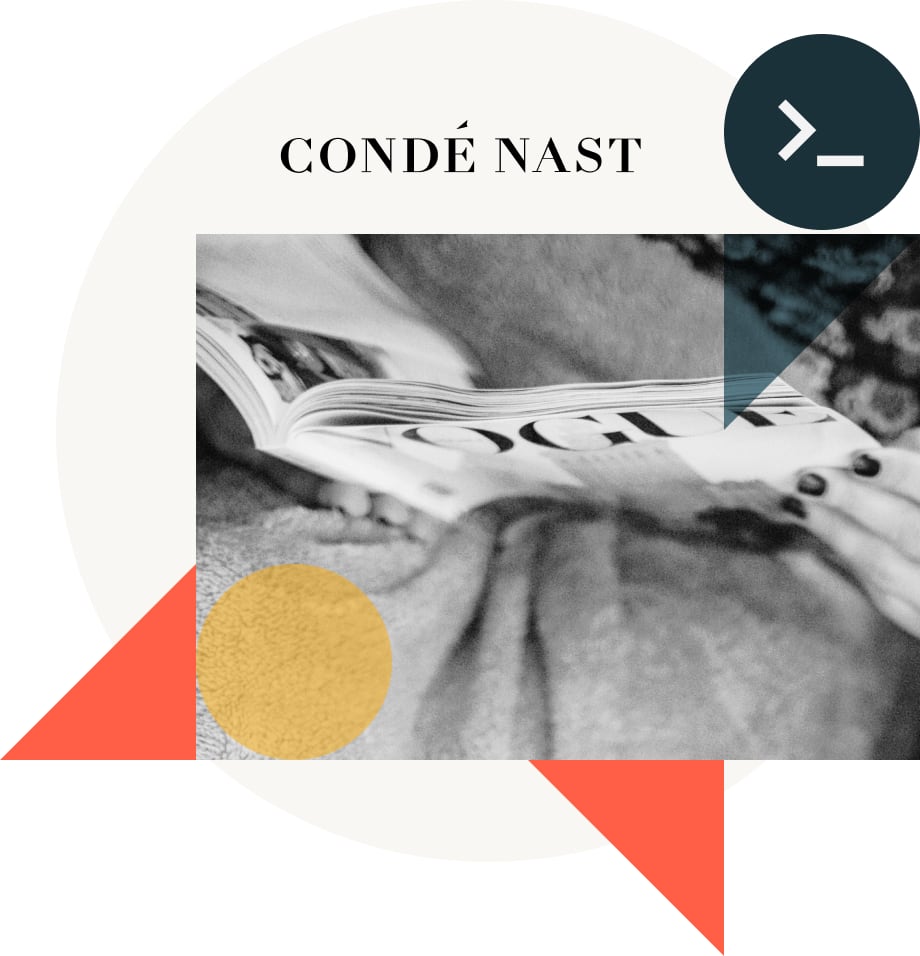
As a global media conglomerate housing over 37 distinct brands, Condé Nast faced the challenge of delivering targeted consumer experiences across their brands amidst fragmented data silos. To overcome this hurdle and remain competitive, they turned to Databricks — significantly accelerating time-to-insights and decision-making. Moreover, the ability to report consistently on a global scale improved data accuracy and operational efficiency. With Databricks, Condé Nast not only optimized its infrastructure to the tune of $6 million in cost savings, but also achieved greater agility in delivering personalized content experiences to a diverse audience, enhancing consumer engagement and loyalty.
Navigating data silos in the age of personalization
As the media industry evolves, so do consumer expectations. Condé Nast — a powerhouse in the world of media and entertainment with global brands like Vogue, The New Yorker, and GQ — realized the importance of delivering tailored content and experiences to its audience. However, their existing data infrastructure was fragmented, hindering their ability to analyze data efficiently, make informed decisions, and provide personalized content at scale. To remain competitive and relevant in a dynamic media landscape, they needed a solution to centralize their data and accelerate their data-driven capabilities. Condé Nast recognized that centralizing data was the key to unlocking a new level of personalization and efficiency. “We struggled to find ways to break out of our data silos across the organization,” said Nana Yaw Essuman, Senior director of data engineering at Condé Nast.
Data Intelligence Platform ushers new solutions that delight customers
Condé Nast chose the Databricks Data Intelligence Platform to better execute their vision of providing a unified view of their consumers. "The ability to leverage the exact same data that has been collected through our data pipelines allows for more consistent reporting in a global fashion,” said Essuman. The lakehouse architecture offered more than just the democratization of data. It has empowered their data team with a comprehensive suite of tools, including Unity Catalog and Databricks SQL, which enables them to securely govern data access and streamline analysis and decision-making processes across the organization, empowering their teams to move faster and smarter. With this newfound agility, Essuman’s team is able to respond more quickly to ever-evolving market trends and deliver content experiences that resonate with their diverse global audience.
Personalized content delivery at scale
Since adopting the Databricks Data Intelligence Platform, Condé Nast has harnessed the full potential of their data for analytics and AI while reducing annual infrastructure costs by approximately $6 million. This not only bolstered their financial health but also empowered them to reallocate resources for crafting personalized consumer experiences globally. Essuman concluded, "Databricks helps us understand the customer from a single view so we can provide the most compelling consumer experience." With a unified data view and robust analytics and AI tools at their disposal, Condé Nast's data team can now easily create personalized content experiences that enhance consumer offerings, fostering higher engagement and loyalty.