Helping companies develop exceptional leaders
DDI uses Databricks Mosaic AI to automate behavioral analysis for clients
to generate a simulation report, down from 48 hours
in recall score using DSPy prompt optimization
in f1 score with Databricks Mosaic AI Model Training
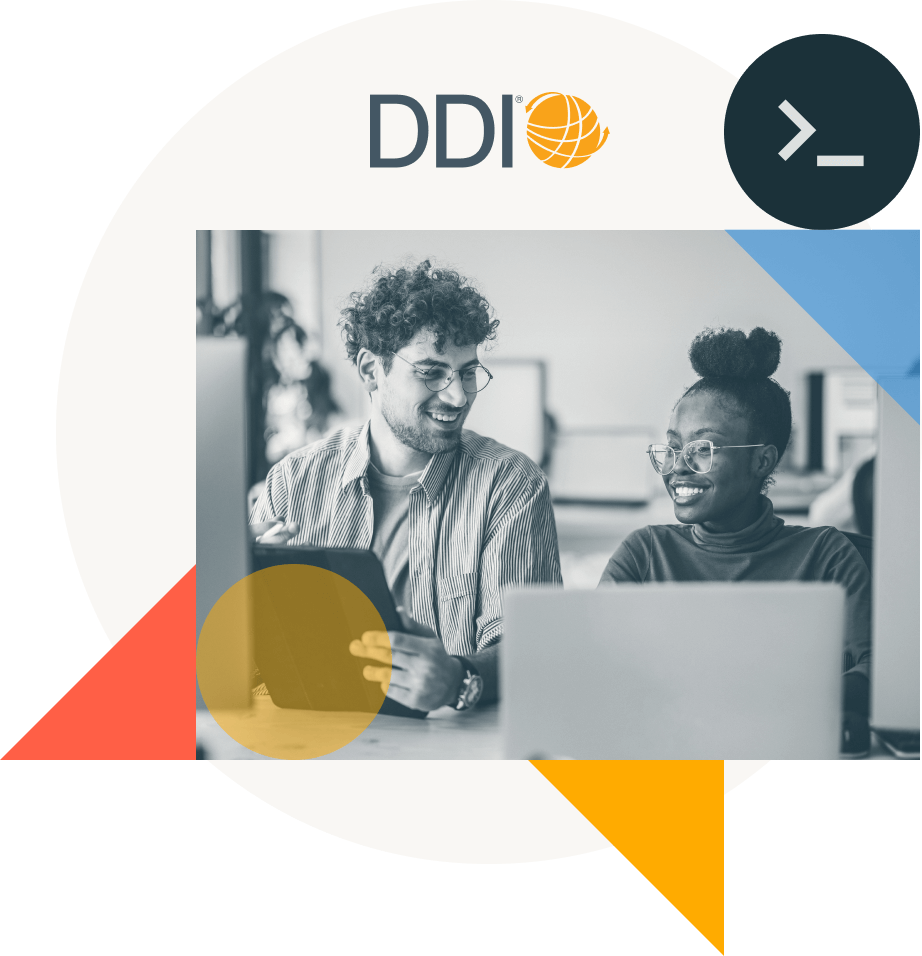
DDI, a global trailblazer in leadership development and assessment, has been serving thousands of clients across various industries for over 50 years. With a reach of more than 3 million leaders annually, including many Fortune 500 companies, DDI sought to automate the analysis of behavioral simulations. These simulations are designed to mimic real-life situations, allowing individuals to demonstrate their decision-making and problem-solving skills. DDI had been relying on human assessors to score simulation responses but sought to leverage machine learning (ML) models to speed evaluation. Partnering with Databricks, DDI used GenAI to quickly deliver more accurate behavioral simulation reports.
Overcoming manual workflow hurdles for behavior assessments
DDI’s mission is to develop exceptional leaders who deliver top results for their organizations. A vanguard in leadership development and talent management, they support clients across a range of industries, including manufacturing, healthcare and finance. Yet, DDI was up against significant hurdles when it came to automating the analysis of its behavioral simulations. Behavioral simulations are structured scenarios crafted to replicate real-world situations in which individuals showcase their decision-making, problem-solving, and interpersonal skills. Chris Coughlin, Senior Director of Assessment Content Design and Development, explained the existing process in detail, "Candidates complete an assessment and submit their responses. Trained human assessors then evaluate these submissions, scoring them based on thorough analysis. Due to the depth of evaluation required and the process of inputting scores into our system, this typically takes 24 to 48 hours."
To reduce manual workflows, DDI sought to use ML models, which could provide faster results and reduce operational costs by eliminating the need for human assessors. At a high level, the challenge included finding a partner capable of supporting the operations required for serving ML models securely, cost-effectively and easily. Previous experiments with GenAI workloads — such as prompt engineering, retrieval-augmented generation (RAG), fine-tuning, and pretraining — had been conducted, but a more comprehensive, end-to-end solution was needed.
DDI faced specific deployment challenges in their ML engineering efforts. These included hardware orchestration, infrastructure management, and scaling issues for exploration and model training. It was also crucial to ensure data privacy and security for data science purposes while managing operational costs. Moreover, the complexity of coordinating with different vendors and partners to achieve these objectives proved inefficient. Fortunately, Databricks offered a unified data intelligence and AI solution, emerging as the ideal partner for infrastructure and support to simplify AI processes.
Training, deploying and maintaining models efficiently
DDI chose the Databricks Data Intelligence Platform to develop and deploy ML models for automating behavioral simulation analysis. With this decision made, the team began experimenting with prompt engineering using OpenAI’s Chat GPT-4. One approach DDI experimented with was few-shot learning, or prompt engineering an existing model with a limited number of examples to perform a new task. This method was particularly useful for quickly adapting models to different types of behavioral simulations. Another prompt engineering technique the team used was chain of thought (COT) prompting, which structures prompts to mimic human reasoning and break down complex problems into smaller, manageable steps. DDI also employed self-ask prompts that allowed the model to generate questions and answers to better understand and process the simulations.
The vast range of experimentations wouldn’t have been possible without the Databricks Data Intelligence Platform. With Databricks, DDI could easily manage the entire ML lifecycle. To start the process of building and experimenting with these models, the leadership company used Databricks Notebooks, which are interactive, web-based interfaces that let them write and execute code, visualize data and share insights seamlessly. Databricks Notebooks facilitated a highly collaborative environment where experimentation became the norm. With Notebooks, DDI orchestrated prompt optimization and instruction fine-tuning for large language model (LLM) at scale, with ease of managed infrastructure support. Prompt optimization with DSPy improved the recall score from 0.43 to 0.98. The instruction fine-tuned Llama3-8b achieved an F1 score of 0.86, compared to the baseline score of 0.76.
Alongside Databricks Notebooks, DDI employed MLflow, an open-source platform developed by Databricks, to streamline the LLM Operations lifecycle. MLflow significantly aided in tracking experiments, logging artifacts as pyfunc
models (i.e. Python function models), tracing LLM applications, and automated GenAI evaluation. Better yet, Databricks’ comprehensive nature allowed DDI to register models to Unity Catalog, a unified governance solution that provides fine-grained access controls, centralized metadata management and data lineage tracking. Moreover, DDI deployed them as endpoints to streamline operations. Groups from Azure Active Directory (ADO) were synced with the Databricks account console via a SCIM provisioner, ensuring secure and organized data management. Coughlin observed, "Unity Catalog, combined with the Model Serving feature, has been particularly beneficial for deploying models with auto-scaling and serverless computing capabilities. These integrated components of Databricks have enabled us to efficiently manage data and deploy AI models to facilitate uninterrupted workflows, from data ingestion to model deployment."
Boosting profit margins and reliability with AI automation
The switch to Databricks has significantly improved DDI's operations, particularly in the automation of behavioral simulations. The implementation of ML models has drastically reduced the simulation report delivery time from 48 hours to just 10 seconds. This automated workflow has enhanced efficiency and productivity. Best of all, the LLMs have demonstrated high reliability and precision in their scoring. This drastic reduction in turnaround time and labor allows leaders to receive immediate feedback, providing customer value that helps DDI scale its operations and invest in future AI applications.
AI and ML are now integral to DDI's strategic objectives, thanks to Databricks' comprehensive support. Databricks provided an end-to-end solution for DDI's data science needs, with tools like Databricks Notebooks to write and execute code, MLflow for tracking experiments and Unity Catalog for data governance and management. According to Coughlin, "Databricks provided us with a dual advantage: the ability to build models and a repository to serve them from. It essentially became our workshop for developing and leveraging AI classification models."
DDI intends to enhance the capabilities of open source base models, such as Llama 3.1, by continuing pretraining (CPT) with Mosaic AI Model Training. CPT learns domain-specific language from a proprietary corpus. The resulting base model trained with DDI’s data will incorporate internal knowledge, allowing it to be further fine-tuned for various simulation analysis use cases. By leveraging increasingly sophisticated models, DDI aims to push the boundaries of what is possible with GenAI in the fields of leadership development and assessment.