Safer oil exploration with AI
To process billions of data points from an oil well
Easily scale to meet massive data needs
To process large data sets, down from 2 days
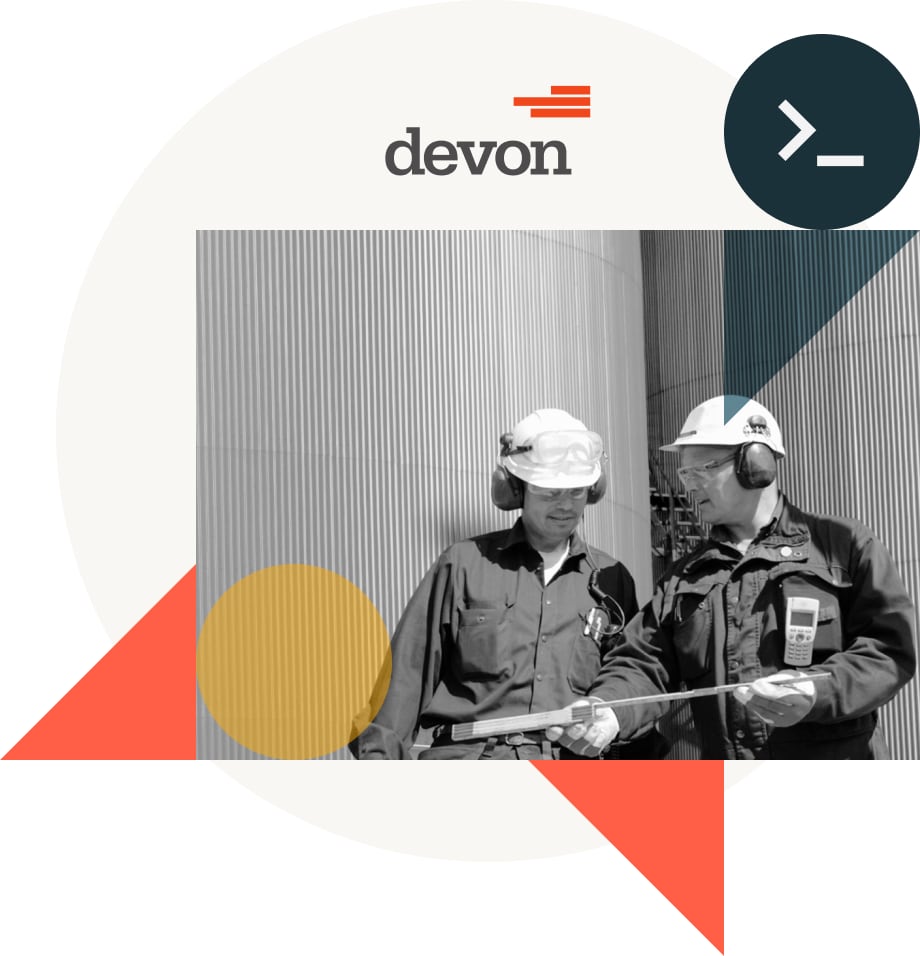
The oil and gas industry has long been plagued by operational inefficiencies and high costs. With the help of AI, forward thinkers in the industry, such as Devon Energy, are leveraging data to reduce operating costs, predict equipment failure, and increase oil and gas output. However, a legacy Hadoop environment challenged Devon Energy’s IT organization to make more data available to those who need it the most. Databricks Data Intelligence Platform has since helped them discover and mine oil more efficiently by reimagining their analytics and machine learning landscape, which has accelerated data pipeline performance at scale while significantly increasing productivity across teams.
Disparate records and disjointed systems couldn’t scale
Devon Energy is hyper-focused on the exploration of unconventional oil and gas reserves in ways that are safe, ethical and environmentally and socially responsible. Leveraging different kinds of data — geophysical data, geochemical data, drill rig/machinery data and seismic data — is key to their approach, with the goal of helping them find oil and gas more efficiently. In order to maintain growth and profitability, it was vital that they develop agile solutions to increase in-field activity while maintaining technical excellence and controlling costs.
Prior to implementing Databricks, Devon Energy was using an on-premises ETL engine to move data from one application to another. This created a web of disjointed applications that became difficult to upgrade, and caused traffic jams in the data flow. The ongoing struggles to access and federate their data for holistic insights inspired the team to take a step back and determine how to reinvent their data warehouse and data integration landscape, in order to improve agility and move workloads to the cloud.
“With billions of records to ingest on a regular basis, our previous systems buckled under the scale,” said Paul Bruffett, a data and analytics architect at Devon Energy. “This made it impossible for our data teams to extract actionable insights from our massive data sets. ” Not to mention, managing their own Apache SparkTM clusters resulted in substantial DevOps investments, developer friction, and wasted compute resources, as they weren’t able to efficiently scale resources to meet the needs of their workloads.
When it came to ML, building, training and deploying machine learning models in a repeatable and reproducible manner was impossible due to disjointed systems and different programming languages. In short, collaboration was nowhere to be seen.
Removing complexities with a fully managed platform
The team at Devon Energy knew that striking a balance between agility and reliability in a single platform was a critical step toward democratizing data access to users across the company, and driving the collaboration they were sorely missing.
With Azure Databricks, Devon Energy was able to streamline data engineering and rapidly accelerate AI innovation. Moving to the cloud simplified their operations and delivered superior data pipeline performance, while auto-scaling eased cluster management and significantly reduced time spent on data engineering and development.
“In the past, each of our data scientists would be allocated a GPU machine, which gets expensive to scale,” explained Bruffett. “With Databricks, we can now easily deploy clusters on-demand that auto-terminate, which helps manage our costs better.”
Finally, data analysts and scientists were able to collaborate, share, and track data and insights across various programming languages, fostering an environment of transparency and improving productivity. An integration with PowerBI allows analysts to easily unlock actionable insights across all their data. And MLflow dramatically reduced the time it takes to build, deploy and iterate on different machine learning models.
Unified data and analytics at scale improves oil exploration
“Our COO has said frequently that we’re a technology company that digs for oil,” said Bruffett. “What that means to me is that innovation, data analysis and technical excellence are really core to our business.” It was the sheer number of potential interpretations for Devon Energy’s data and the constant evolution of drilling machine schema and tactics that made a scaled-out compute platform the only possible solution.
Thanks to Databricks, the Devon Energy team is now able to unify their analytics journey by building reliable data pipelines that feed downstream business intelligence and machine learning models. They have been able to scale to over a thousand cores in order to process a full well in 1–2 hours, to help maximize oil and gas production. Queries that used to take two or more days now take less than half an hour. Empowering people with a self-service platform made everyone more productive and operations more efficient.
“The developers spoke loud and clear,” says Bruffett of his team’s reaction to the migration to Databricks. “Prior to Azure Databricks, it was taking two days or more to query a subset of data on a SQL Server instance. Now, it takes about 30 minutes for the entire data set.”
Databricks has given Devon Energy the platform necessary to successfully build an end-to-end data pipeline that drives frontline decisions, enabling them to more efficiently and safely identify new pockets of oil and gas for the communities they serve.