Taking the guesswork out of buying and selling vehicles
Higher conversion rate for marketing offers
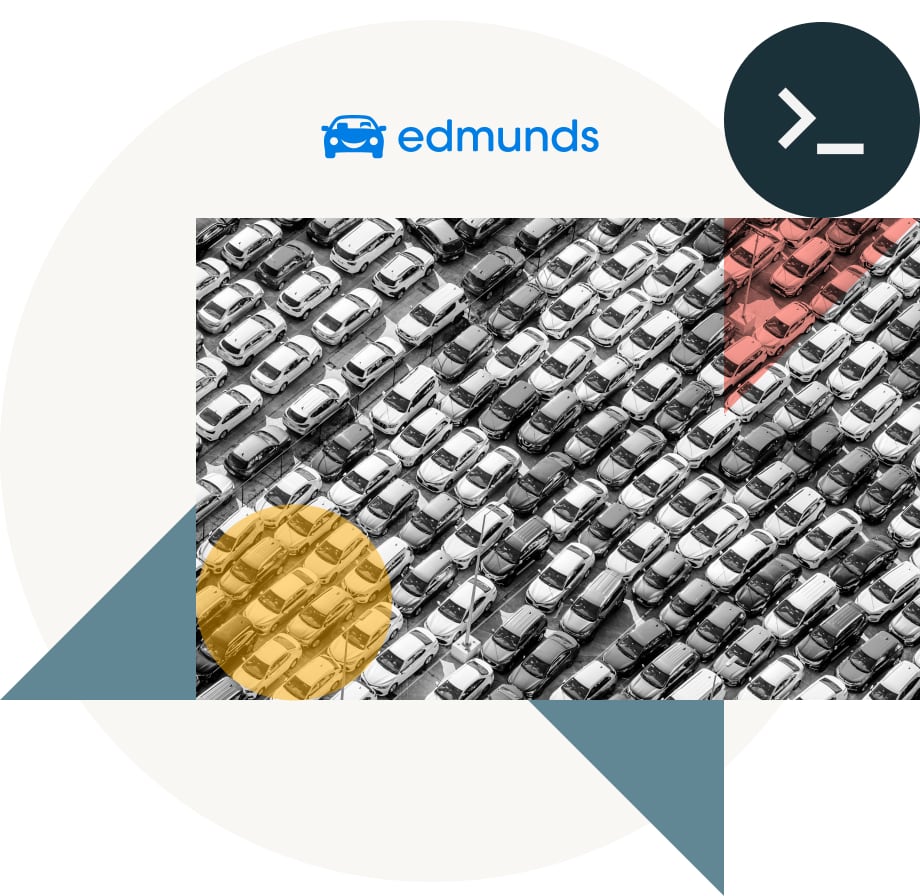
With over 50 years of experience, Edmunds is recognized as an industry leader for their innovations in digital car shopping and is the trusted resource for car shoppers online. Relying on a variety of data feeds at different frequencies and formats, Edmunds delivers market-based analytics to support customer decision-making. However, irregularities in the automobile industry have rapidly increased the rate of changing car prices and inventory. Edmunds needed to improve the speed, frequency, accuracy and access to data to empower and allow teams to improve pricing models, deliver impactful marketing offers and power self-service tooling for a variety of internal users to make smarter decisions. After migrating to the Databricks Data Intelligence Platform, Edmunds has increased their ability to ingest data at scale and has removed barriers to entry so that different users can apply insights to boost customer satisfaction and conversions, while reducing operational costs.
Struggling to provide actionable insights to users
Based on historical consistency, it’s a known fact that used cars depreciate in value. However, due to microchip shortages and supply chain deficiencies caused by the COVID-19 pandemic, today’s used cars are actually appreciating in value. According to Edmunds data from June 2022, the average transaction price for used vehicles increased 8.9% year over year (YOY), while new cars are up around 11.6% YOY. Additionally, new car inventory has shrunk notably due to these global supply chain issues. Responding to these irregularities, Greg Rokita, associate vice president of technology at Edmunds says, “In this challenging environment, we need to provide customers with optimal decision-making tools to help them find their dream car, get the best deal, trade-in at the right time, or buy a used car for the first time. With these new use cases popping up, our mechanisms to create customer value must be more flexible to accommodate variable circumstances.”
Critical to fulfilling these customer car shopping needs is Edmunds’ ability to not only provide accurate pricing models, but create a customer experience that drives conversion through marketing offers and new features within the car buying journey. However, without a centralized data repository, data access was complex which slowed ingestion and model development. Pricing updates only happened twice a month, which means they were basically obsolete as soon as customers saw them, and the process required multiple people, manual intervention and multiple days. And internal teams — from product managers to business analysts — weren’t able to discover insights that could help improve customer engagement and conversion on marketing offers.
In order for Edmunds to generate near real-time analytics in a timely and efficient manner, they needed an advanced platform that would allow them to unify their data, allow cross-team collaboration and empower non-technical users to make data-driven decisions. With a modern lakehouse approach, they would be able to support customer decision-making through use cases like pricing, inventory and recommended marketing offers.
Democratizing data access and AI with lakehouse architecture
Edmunds chose to modernize their data infrastructure on the Databricks Data Intelligence Platform, so that they could unify their environment and open up data access across various teams within the organization.
By eliminating the barriers that prevented collaboration and unobstructed data access, Edmunds is now able to optimize their machine learning models to better meet today’s car shopping needs with near real-time insights that inform pricing, marketing strategies, product roadmap and more. Greg explains, “With lakehouse architecture, we’re able to update pricing models within a matter of one or two hours, and we’re running it daily. That paired with our marketing offers and optimized user experiences, our customers have everything they need to make informed selling and buying decisions.”
Team silos have become a thing of the past with the ability to ingest diverse data sets — from structured to streaming — to be used for AI and advanced analytics use cases across the business. Now, Greg says, “Casual business users without a lot of technical knowledge can use Databricks SQL to create dashboards and monitor metrics that were previously too difficult to do. This has allowed us to make smarter decisions on behalf of our customers.”
Anh Dao Pham, VP of product and program management at Edmunds, is one of those casual users. Instead of distracting data teams for specific queries and then waiting days to have custom reports published, Ahn queries the data herself using basic SQL skills. Today, she’s created at least 40 different dashboards and numerous reports just by slicing and dicing performance data. Ahn says, “Multiple teams use Databricks regardless of scripting language, which makes data processing incredibly fast and collaborative. I use it to gain insights from a customer performance standpoint. Other teams use it to merge different data sources to make their processes perform better.” Leveraging democratized data in Databricks, Edmunds is able to optimize their machine learning models for the benefit of customer decision-making.
Delivering accurate customer insights at scale
With Databricks Data Intelligence Platform providing a unified approach to data and analytics, a variety of Edmunds’ teams are able to more proficiently drive bigger business impacts. Anh highlights results from the product team saying, “Our initial conversion rate for customers receiving marketing offers on our website was about 9%. Through iterating with the insights I received from Databricks, we’ve been able to improve the user experience, and now convert at about 28% for those customers.” Based on those results, Edmunds has scaled website offers to influence more customers and increase revenue.
Moving forward, Edmunds is building on their capabilities with Databricks to further expand data use throughout the organization. The transformation has been palpable as multiple teams, including their product, statistics, software development and marketing teams are all tapping into these newfound insights to boost productivity and make better decisions on behalf of their customers. With data and AI at the core of Edmunds’ mission to deliver value to customers, they will continue to rely on Databricks to stay at the forefront of innovation in their industry.