Scaling global policy and intelligence for a better tomorrow
FiscalNote improves model deployment, helping clients succeed strategically
Faster ML model deployment
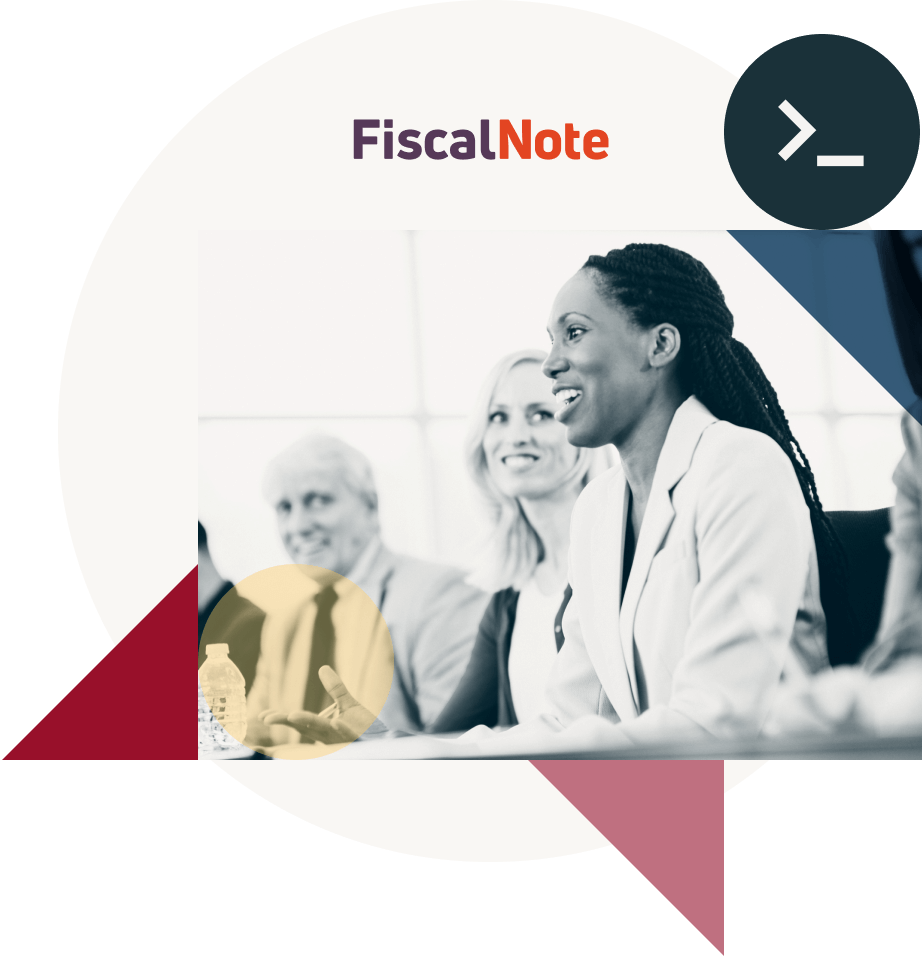
Established in 2013 to transform access to legislative and regulatory data — whether at the local, state or federal level — FiscalNote has developed machine learning (ML) and natural language processing (NLP) technologies to give customers access to policy and geopolitical insights. The software company serves a wide range of clients, including nonprofits, government agencies and over half of the Fortune 100. Before Databricks, FiscalNote had to stitch together various components necessary for their AI model deployments, slowing down their internal workflows. By adopting Databricks, the business streamlined model deployment, making the process 3x faster and increasing analyst productivity.
Facing major hurdles in AI/ML model deployment
FiscalNote is a software company at the forefront of analyzing government data and legislative processes. Leveraging data science and machine learning, FiscalNote provides actionable insights to businesses, advocacy groups and governments. They were one of the first to offer automated analysis of legislative outcomes, policymaker effectiveness and nuanced sentiment analysis. Their advanced analytical models transform how stakeholders engage with policymaking processes, making complex legislative environments accessible and actionable to a customer base that encompasses small nonprofits, government agencies and large corporations.
While working with disparate unstructured and structured data sources, FiscalNote encountered obstacles with AI model deployment. Their data teams were hampered by the cumbersome processes required to stitch together various components essential for model deployment, such as tracking artifacts and builds. This extensive, fragmented process limited the team’s cadence for deploying and updating certain AI models to around once a year. Vlad Eidelman, Chief Technology Officer at FiscalNote, explained, “We’re primarily focused on aiding organizations in understanding and responding to global legislative, regulatory and financial shifts. The challenge was meeting client expectations — which necessitated models in different locations, of different sizes and with different capabilities — to satisfy our clients’ need for accuracy and our desire for maintainability and efficiency.” Since each deployment phase extended over several weeks, these timelines restricted FiscalNote’s ability to respond to a rapidly changing legislative landscape and offer the most up-to-date ML models their clients needed.
Moreover, FiscalNote’s requirement for no-disruption deployments added another layer to already challenging processes. Simply put, their infrastructure required extensive custom coding efforts to ensure that model updates did not disrupt the operation of existing models. This not only increased the operational burden on team members but also posed risks to data integrity and service continuity. Finally, a lack of discoverability of essential data assets made it difficult for data scientists to access and use the necessary data, further impeding effective model development and deployment. To address these hurdles, FiscalNote chose Databricks — a unified data analytics platform with AI/ML components that promised to accelerate their model deployment capabilities.
Streamlining ML lifecycle management with advanced tools
Starting with MLflow — an open source platform developed by Databricks that helps manage and deploy ML models — FiscalNote focused their initial efforts on enterprise scalability and reliability. This helped the data science team reduce the amount of time they spent tracking artifacts, model versions and notebooks to focus on tasks that couldn’t be automated with artificial intelligence. Thanks to MLflow, FiscalNote teams spent less valuable time and resources on MLOps and invested more in data science. Using this feature alone, FiscalNote experienced faster time to market and scaled up their model deployments to an as-needed basis.
Additionally, FiscalNote leveraged Mosaic AI Model Serving, a comprehensive service used for deploying, managing and monitoring machine learning models, whether they are developed by Databricks or sourced from other providers. Model Serving has made it significantly easier for FiscalNote’s data teams to deploy a model without needing to worry about building an API, hosting and scaling their infrastructure or planning no-disruption deployments. With Model Serving, FiscalNote possessed the capability to roll out various ML models tailored to specific needs. As an example, their data teams used these ML models to support standard extract, transform and load (ETL) pipelines to ingest data from various sources, perform NLP tasks (e.g., summarizing and sentiment analysis) and execute binary classification workflows (e.g., analyzing whether a congressperson will/will not vote on a particular bill).
Eidelman elaborated on the progress FiscalNote has seen thus far, “We’ve only just started using Databricks Model Serving within the last six months. Our former workflows required our teams to manually store, serve and build models, which meant we also had to piece together all the infrastructure. Now, we can worry less about logistics and focus more on approach.” Because of Databricks tools like MLflow and Model Serving, FiscalNote reduced bottlenecks with their data platform teams to support innovation at scale.
Speeding time to insight and time to market
Databricks has dramatically improved FiscalNote’s operational efficiency and effectiveness in deploying machine learning models. Eidelman, highlighting the key benefits, stated, “Databricks allows our team to build and deploy ML models faster, reducing time to market and allowing our employees to be more productive and effective.” With increased speed in their processes and greater productivity from their employees, FiscalNote could boost their responsiveness to legislative changes, ensuring that their insights remain timely and relevant.
Since this shift toward faster model deployment directly correlates with a reduction in time to market for new models, FiscalNote can now deploy 3x the number of models annually, compared to their output before Databricks. Because of these substantial gains, the software company’s data science team feels empowered to freely experiment and rapidly iterate on models, ensuring that solutions remain perfectly aligned with current market needs.
Now, with the efficiencies gained from Databricks, FiscalNote has the bandwidth to be more dynamic in their response to legislative changes. This newfound agility enables FiscalNote to process and analyze legislative data quickly and accurately. As a result, they can deliver timely, actionable intelligence that helps clients swiftly adjust to new laws and regulations. Leveraging this newfound advantage, FiscalNote can significantly enhance the value they deliver to customers, reinforcing the company’s role as a critical resource in the legislative and regulatory domains.