Reinventing mobile banking with ML
Seconds to perform complex analytics compared to 6 hours
Delta Lake has replaced 14 databases
Improvement in engagement on the app
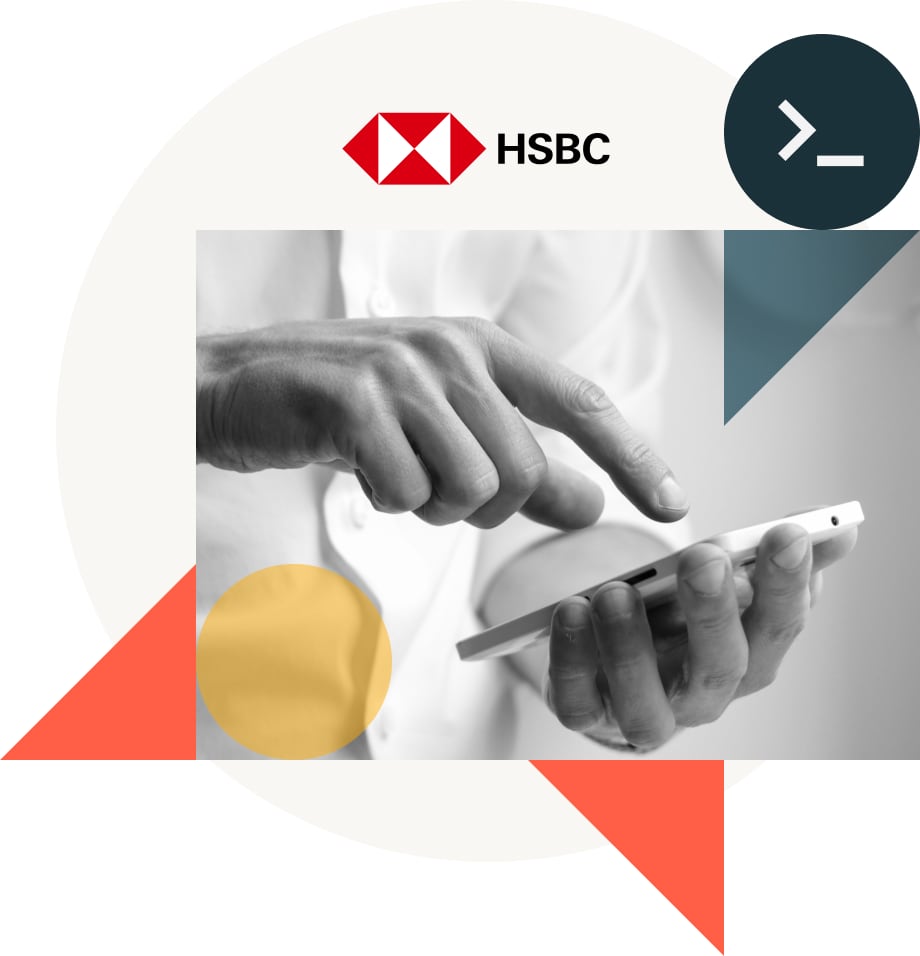
As one of the largest international banks, HSBC is ushering in a new way to manage digital payments across mobile devices. They developed PayMe, a social app that facilitates cashless transactions between consumers and their networks instantly and securely. With over 39 million customers, HSBC struggled to overcome scalability limitations that blocked them from making data-driven decisions. With Databricks, they are able to scale data analytics and machine learning to feed customer-centric use cases including personalization, recommendations, network science, and fraud detection.
Data science and engineering struggled to leverage data
HSBC understands the massive opportunity for them to better serve their 39+ million customers through data and analytics. Seeing an opportunity to reinvent mobile payments, they developed the PayMe, a social payments app. Since its launch in their home market of Hong Kong, they have become the #1 app in the region amassing 1.8+ million users.
In an effort to provide their fast growing customer base the best possible mobile payments experience, they looked to data and machine learning to enable various desired use cases such as detecting fraudulent activity, customer 360 to inform marketing decisions, personalization, and more. However, building models that could deliver on these use cases in a secure, fast and scalable manner was easier said than done.
-
Slow data pipelines resulted in old data: Legacy systems hampered their ability to process and analyze data at scale. They were required to manually export and sample data, which was time consuming. This resulted in the data being weeks old upon delivery to the data science team which blocked their ability to be predictive.
-
Manual data exporting and masking: Legacy processes required a manual approval form to be filled out for every data request which was error-prone. Furthermore, the manual masking process was time consuming and did not adhere to strict data quality and protection rules.
-
Inefficient data science: Data scientists worked in silos on their own machines and custom environments, limiting their ability to explore raw data and train models at scale. As a result, collaboration was poor and iteration on models were very slow.
-
Data analysts struggled to leverage data: Needing access to subsets of structured data for business intelligence and reporting.
Faster and more secure analytics and ML at scale
Through the use of NLP and machine learning, HSBC is able to quickly understand the intent behind each transaction within their PayMe app. This wide range of information is then used to inform various use cases from recommendations to customers to reducing anomalous activity.
With Azure Databricks, they are able to unify data analytics across data engineering, data science, and analysts.
-
Improved operational efficiency: features such as auto-scaling clusters and support for Delta Lake has improved operations from data ingest to managing the entire machine learning lifecycle.
-
Real time data masking with delta lake: With Databricks and Delta Lake, HSBC was able to securely provide anonymized production data in real-time to data science and data analyst teams.
-
Performant and scalable data pipelines with Delta Lake: This has enabled them to perform real-time data processing for downstream analytics and machine learning.
-
Collaboration across data science and engineering: Enables faster data discovery, iterative feature engineering, and rapid model development and training.
Richer insights leads to the #1 app
Databricks provides HSBC with a unified data analytics platform that centralizes all aspects of their analytics process from data engineering to the productionization of ML models that deliver richer business insights.
-
Faster data pipelines: Automating processes and increased data processing from 6 hours to 6 seconds for complex analytics.
-
Descriptive to predictive: Ability to train models against their entire dataset, has empowered them to deploy predictive models to feed various use cases.
-
From 14 databases to 1 Delta Lake: Moved from 14 read replica databases to a single unified data store with Delta Lake.
-
PayMe is #1 app in Hong Kong: 60% market share of the Hong Kong market making PayMe the #1 app.
-
Improved consumer engagement: Ability to leverage network science to understand customer connections has resulted in a 4.5x improvement in engagement levels with the PayMe app.