Optimizing touch points across the entire customer journey
Faster pace of new feature releases
Increase in customer engagement due to optimized message delivery
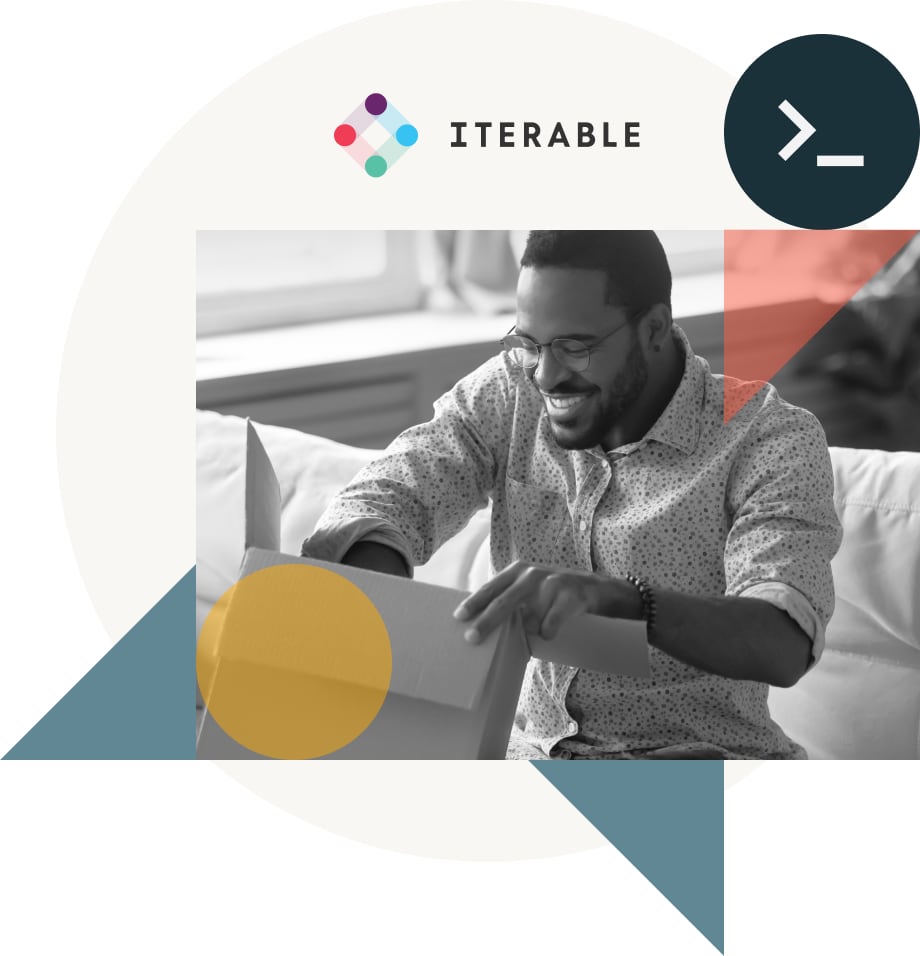
Captivating an audience and understanding customer journeys are essential to creating deeper brand-customer connections that drive growth, loyalty and revenue. From helping medical practitioners build trust with new patients to ensuring that food delivery users feel connected to their culinary community, Iterable helps more than 1,000 brands to optimize and humanize their marketing in today’s competitive landscape. This need to build personalized and automated customer experiences for its clients drove the company to find a fully managed platform that would simplify infrastructure management, make collaboration possible and give it the ability to scale for analytics and AI. With Databricks Data Intelligence Platform, Iterable can harness diverse, complex data sets — including conversion events, unique user labels, engagement patterns and business insights — and facilitate rapid prototyping of machine learning models that deliver top-notch and personalized user experiences for higher-converting marketing campaigns.
Rigid infrastructure stifles the use of ML to build customer-centric solutions
Consumers want to do business with brands that they feel connected to. And in today’s world, where most brand-customer interactions happen online, it’s critical that brands personalize digital customer touch points to build powerful and lucrative customer connections. Iterable helps brands build better relationships with customers by empowering marketers with the ability to deliver individualized cross-channel communications for customers at every stage along their journey with a brand. They accomplish this through customer segmentation and personalization, which delivers the right messages to the right audience, and campaign optimizations such as identifying the best times to send messages to customers and which engagement channels are working best. “We believe marketers know their business and their messaging best,” says Sinéad Cheung, Principal Product Manager at Iterable. “We’re here to augment a marketer’s ability to achieve their business objective through the use of their own data.”
The success of Iterable’s customers is fueled by its commitment to constant innovation — it creates and deploys cutting-edge solutions that help brands stay ahead of their competition and meet the growing and evolving expectations of their customers. Iterable’s ML-powered solutions, as an example, help brands achieve business goals by revealing consumer preferences and providing marketers with the tools to use those insights to boost engagement and conversion. But while such a revolutionary approach to data and AI application is incredibly impactful for the brands leveraging this technology, the creation and maintenance of these solutions is quite complex. Aside from accounting diverse data variables and drifts in the model, AI solutions in the marketing space have to meet the demands of new regulatory changes and the growing appetite for more privacy protection.
Iterable initially built its data infrastructure with AWS native tools, including EMR. This created significant operational overhead, as building its environment was resource-intensive and costly to maintain. As a result, it took a lot of work for Iterable to scale the level of data ingestion and rapid prototyping of machine learning models needed not only to support its customer requirements but to be able to respond quickly to changes in the market. Ankur Mathur, Senior Engineering Manager for AI and Experimentation at Iterable, explained, “It was really hard to leverage our data and rapidly build models with EMR, as we spent significant resources maintaining infrastructure and debugging Spark issues rather than developing solutions to help our customers win.”
The need to identify the correct signals, adjust to new market variables, and pivot to new requirements and features quickly required Iterable’s data team to have the correct tools to meet the various operational and development demands. This is where Databricks Data Intelligence Platform came in.
Creating deeper customer engagement with data, analytics and AI
Since moving to Databricks Data Intelligence Platform, Iterable has been able to wrangle their diverse data to create Predictive Goals — a data-enriching segmentation tool that allows marketers to create bespoke, goal-oriented predictive segments with their first-party data.
Revitalizing data infrastructure would typically be an incredibly time- and work-intensive process for this small task force. However, with the help of Databricks Data Intelligence Platform, rapid prototyping was made possible and collaboration was facilitated throughout the model lifecycle — from simplified access to data and automated cluster management to streamlined experimentation and iteration on models. Not only did Databricks Data Intelligence Platform make the data infrastructure revitalization process quick and painless for the AI team, but the experience helped instill data engineering and machine learning best practices for Iterable’s wide Product and Engineering teams, ensuring Databricks-inspired optimizations continue across the organization.
“Databricks Data Intelligence Platform makes collaboration very easy,” says Cheung. “It has made communication much smoother and helps us operate faster. This increase in speed and efficiency has not only improved productivity but also creativity across our team.”
Delta Lake, a component of the lakehouse architecture, provides Iterable with the means to create a unified view of all its data in a secure and compliant manner. This heightened data governance is key, as strict client contractual obligations prohibit data mixing across customers. Consequently, Iterable is able to glean an accurate and complete view of its customers’ needs and can develop effective solutions to meet them. With reliable and consistent data being fed by over 5,000 pipelines at its disposal, the data science team harnesses MLflow to then train, experiment and track hundreds of models across more than 2,000 projects for the company’s customers. From model development, experiment tracking, model management and model deployment, Databricks MLflow has simplified the machine learning lifecycle management process for Iterable.
“Being shepherds of a customer’s data is very important to the company,” said Mathur. “With Databricks Data Intelligence Platform, we have the architecture to effectively and securely manage customer data and leverage it to create ML solutions that will help them build stronger relationships with their clients.”
Expanding the adoption of lakehouse fuels new human-centered AI solutions
Iterable now has the means to handle its variable data volumes and rapidly growing machine learning road map thanks to the lakehouse architecture. This ensures consistency and reliability in the data provided to create better models for continued innovations that aid in customer conversion, engagement and retention.
This impact can be observed with its audience segmentation tools, like Predictive Goals and Brand Affinity, where it has seen improved customer engagement by 30% in some cases. Similarly, with its AI campaign optimization suite featuring Send-Time Optimization, Iterable is experiencing a 50% adoption rate across its customers.
The Iterable team now has a centralized view of all data, code and models, and its solutions are powered by ML models built in the Databricks Data Intelligence Platform. As a result, Iterable’s marketers have a limitless capacity to create personalized user experiences at scale.
“We’re here to provide marketers with powerful AI solutions that help connect people with products that bring them joy,” concludes Cheung.