Giving clients more accurate market insights, faster
Kantar Worldpanel uses Databricks Mosaic AI to fine-tune decision-making
Parameter model fine-tuned on
Databricks Mosaic AI
Accuracy of training data generated
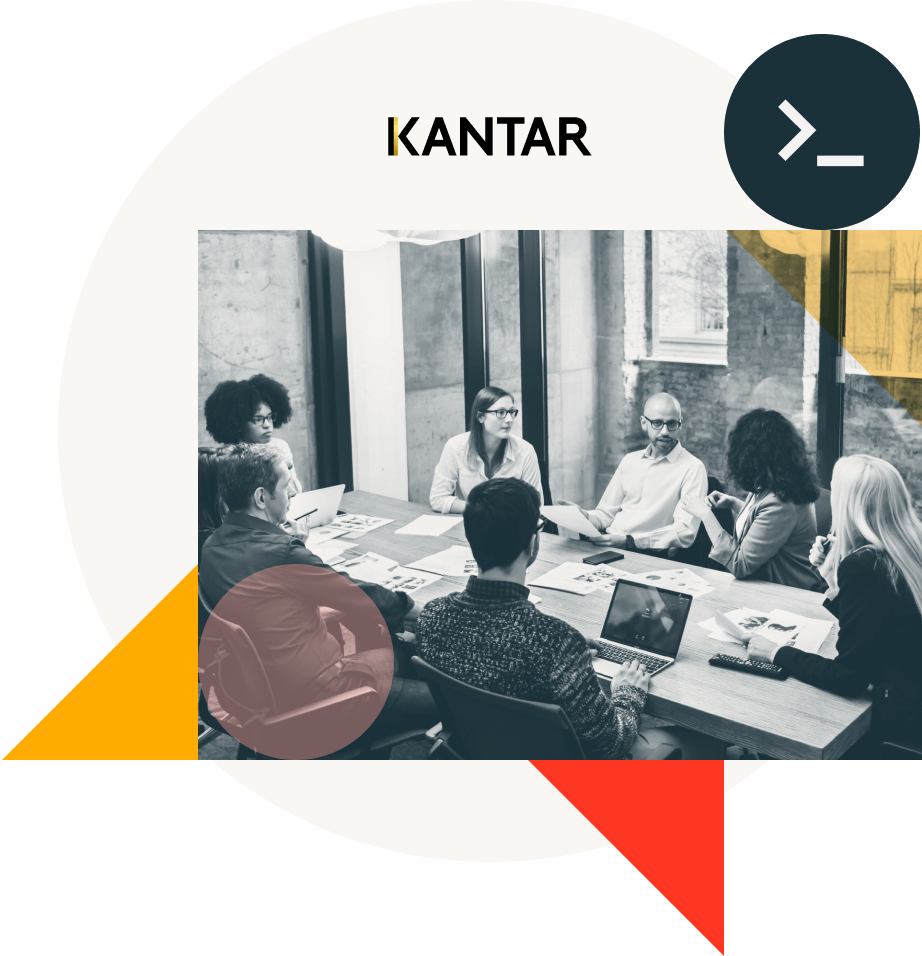
Kantar Worldpanel is a leading market research company specializing in consumer data analysis that helps clients make informed decisions. Kantar Worldpanel faced challenges with some of their legacy systems, which were inflexible, resource-intensive and required specialized skills. With help from the Databricks Data Intelligence Platform, they’ve seen enhanced data accuracy, streamlined workflows and optimized resource utilization. Now Kantar Worldpanel can experiment with advanced AI/ML models within Databricks Mosaic AI, generating training data faster and more efficiently. This has helped them deliver new use cases such as providing their clients with more accurate market insights with their newly developed product descriptions proof of concept, built and maintained in Databricks.
Proprietary systems limit data democratization and experimentation
Kantar Worldpanel is a prominent international market research company that collects and analyzes consumer data, primarily in the fast-moving consumer goods (FMCG) sector. Their mission is to provide actionable insights to manufacturers and retailers, enabling them to understand consumer behaviors and make informed business recommendations to clients. At the heart of Kantar Worldpanel’s operations is data and AI. Ana Portêlo, Head of Data Science at Kantar Worldpanel, explained, “We sell insights in the form of data. AI is helping us automate and extract higher quality insights from our data to serve our clients better.”
Kantar Worldpanel’s business use cases primarily revolve around generating insights from consumer data faster and more accurately. A significant proof of concept (POC) they’re working on involves fine-tuning a model that links descriptions from paper receipts to product barcode names. This upstream process allows Kantar Worldpanel to identify what products were purchased by which buyers, which is later converted into insights and sold to clients. Kantar is also exploring the use of Mosaic AI Vector Search to compare these product descriptions more effectively.
Kantar Worldpanel faced several challenges with their existing systems that prompted them to explore Databricks for new AI-driven use cases. “The nature of some of our legacy systems isn’t as flexible and scalable as what we’re currently getting from Databricks,” said Portêlo. Maintaining and managing them can be resource-intensive for the engineers. Requiring an outdated programming skillset not only limits the accessibility of a system but also makes it challenging to find and retain the necessary talent.
Rui Teixeira, Senior Data Scientist at Kantar Worldpanel, highlighted the urgency of adopting new technologies. “We’re in a place where AI is taking this field by storm, and we want to leverage these GenAI tools because we know that the results will be much better. We want to be able to experiment with different POCs to see how we can improve our business and our results.” The combination of an inflexible system, resource-intensive maintenance and the need for specialized skills made it clear that Kantar Worldpanel needed a more modern, scalable solution.
Supercharging GenAI experimentation in one platform
The Databricks Data Intelligence Platform provides a scalable, flexible and integrated environment that supports Kantar Worldpanel’s advanced AI and machine learning initiatives.
The data science team leverages MLflow, an open source platform developed by Databricks, to manage the full machine learning lifecycle. This component allows them to track experiments, reproduce runs and deploy models more efficiently. “Databricks simplifies many processes, particularly cluster management and storing results using MLflow,” explained Teixeira. “It’s very easy to integrate and check the results. When downloading models, it’s also very simple to replicate code. When you use Databricks, processes are more high level, which is nice.”
The team is also exploring using Mosaic AI Vector Search to perform detailed comparisons and linkages between receipt and reference product descriptions. This stands to improve the accuracy and efficiency of their data processing and serve more comprehensive insights to manufacturing and retail clients.
The flexibility and comprehensive nature of the Databricks Platform supports multiple AI initiatives simultaneously — which is exactly what the data science team was excited to do. “We’re able to experiment with different AI POCs all within the same platform. Now we can compare and optimize various models effectively — and it’s easy,” added Texeira. “We just download the models from Databricks Marketplace and we run an experiment. We can easily understand which one performs better because the labels tell us at the end if the results are correct or wrong.”
Unity Catalog, the Databricks unified governance solution, wraps all Kantar Worldpanel’s data sharing and collaboration up in a secure package. “We work in very different environments. It’s nice to share data across teams and know that it’s protected,” said Texeira.
Generating optimized results, faster
Teixeira highlights the effectiveness of their GenAI models, stating, “We've experimented with Llama, Mistral, GPT-4 and GPT-3.5, all within the Databricks Platform. Ultimately, GPT-4 provided better answers, with an accuracy of 94%.” This data accuracy translates directly into better insights for Kantar’s clients, enabling them to make more informed business decisions.
“Now that we know the model that produces the best quality outputs for our task, we can use it to generate training data to fine-tune a smaller model and serve it in our production pipeline. Smaller models are not only more cost-effective but more performant,” explained Portêlo.
Additionally, use of the Databricks Data Intelligence Platform has led to significant resource optimization. “The reason we wanted to experiment with all of these models in the first place was to generate training data faster, without using a lot of human resources. And we’ve done that — we’ve automatically generated a training dataset of about 120,000 pairs of receipt descriptions and barcode names with an accuracy of 94% in just a couple of hours,” said Portêlo. “We can allow our manual coding teams to focus on more discrepant results instead of generating masses of training data. At the same time, we can free up our engineering resources to focus more on core developing tasks, like modernizing other model serving approaches within our current data processing platform on Databricks. This seamless interaction allows us to focus our resources on more specialized, impactful tasks.”
Portêlo’s team has also been able to streamline their workflow. According to Portêlo, “As data scientists, we don’t need to rely so much on engineers to set up clusters with all the parameters or set up services. With one or two lines of code, we can download models, work and experiment with them and have a cluster that can handle the models — all in a single place and in a very seamless way.”
As they look to the future, Kantar Worldpanel remains committed to exploring new AI-driven solutions and expanding their capabilities, ensuring they continue to provide cutting-edge insights to their clients. “The product descriptions POC is just one of many use cases that we’re trying to leverage with GenAI. Being able to have a partner like Databricks enables us to experiment and put models into production in a cost-effective way,” concluded Portêlo.
To learn more about how Kantar Worldpanel gives their customers deep insights into their audience through trusted market research, visit: https://www.kantar.com/