Databricks Customers
Discover how innovative companies across every industry are leveraging the Databricks Data Intelligence Platform for success
Adobe is using the Databricks Data Intelligence Platform to help bring creativity to life, with end-to-end data management that unifies all data and AI at scale for over 92 teams and with 20% faster performance.
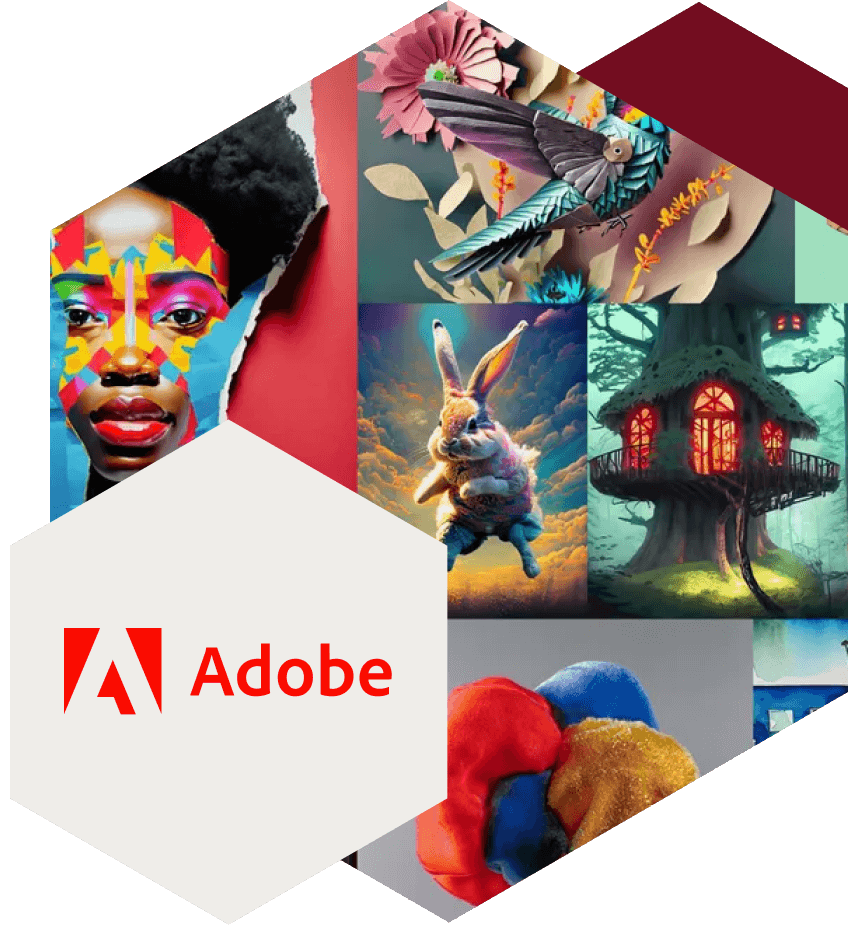
Featured Stories
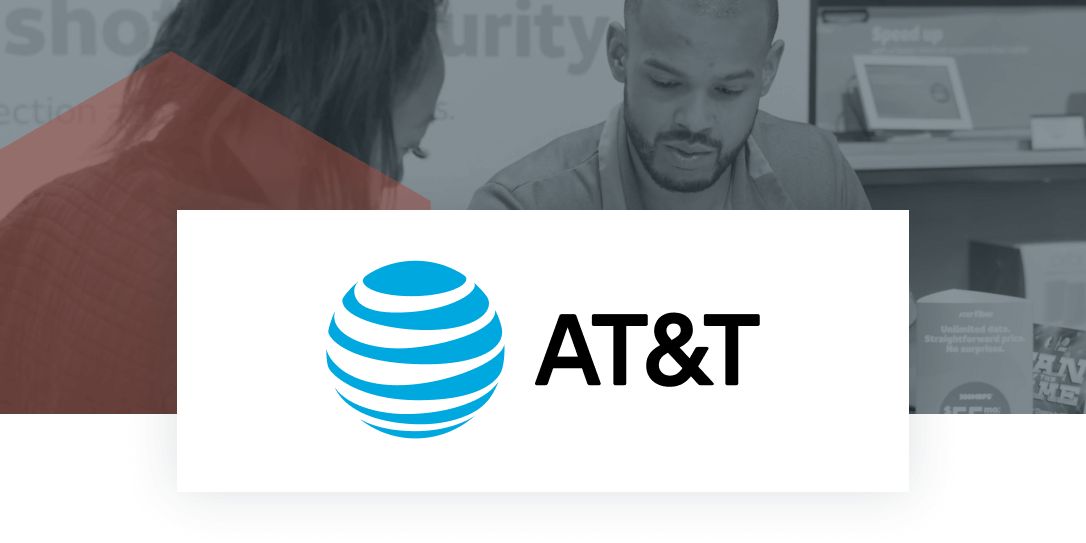
Customer Story
AT&T democratizes data to prevent fraud, reduce churn and increase CLV
Databricks has helped AT&T accelerate AI across operations, including decreasing fraud by 70%–80%
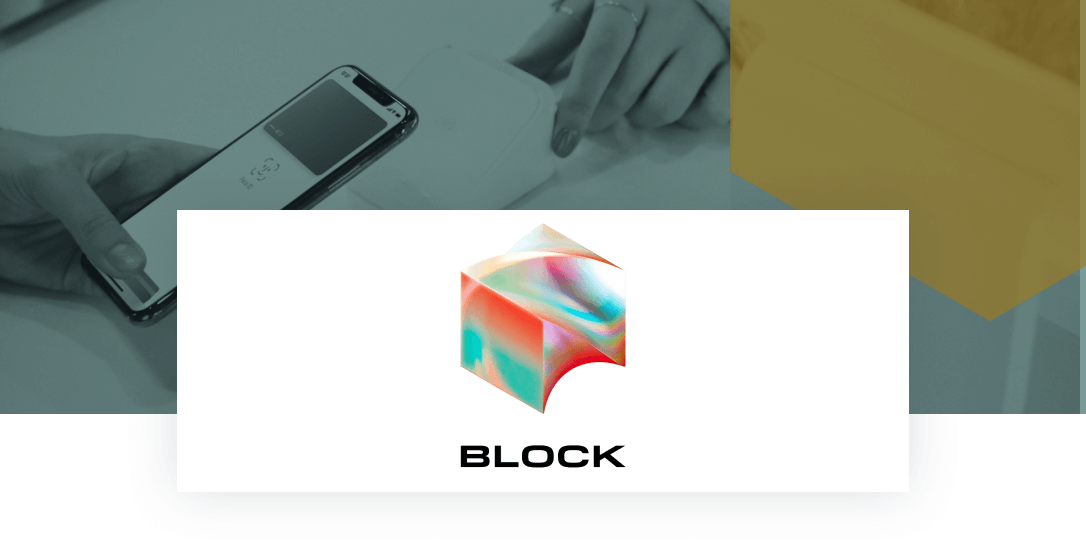
Customer story
Expanding access to the economy with data and AI
Block (Square, CashApp, Tidal) uses Databricks to deliver data + AI-driven financial services that facilitate access to economic opportunities for millions of businesses
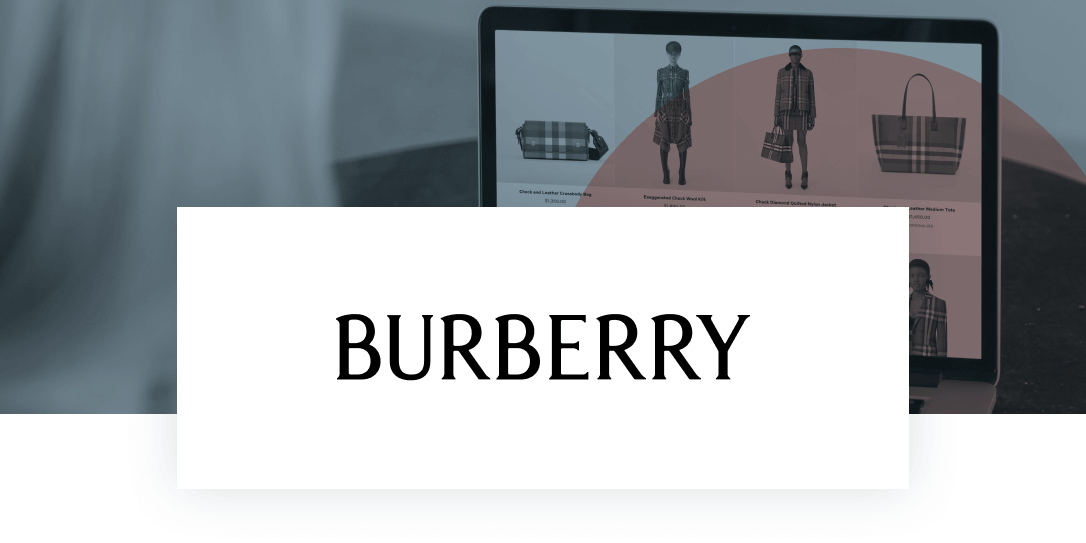
Customer Story
Burberry creates a more personalized shopping experience
Burberry sees a 99% reduction in latency for customer clickstream data with Databricks
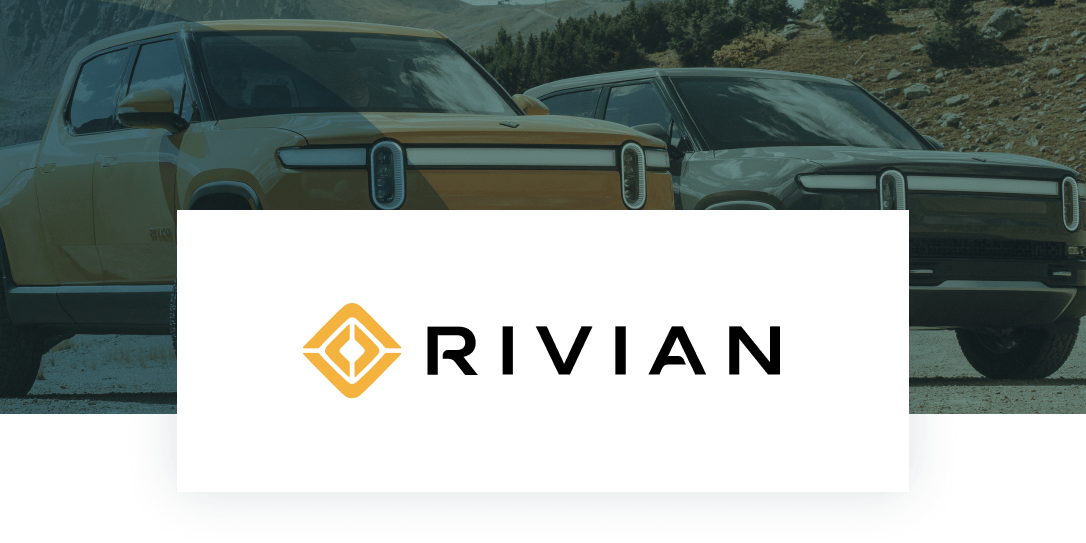
Customer Story
Driving into the future of electric transportation
Rivian uses Databricks to harness IoT streaming data insights from 25,000+ vehicles, delivering AI innovations to market faster and providing better experiences for drivers
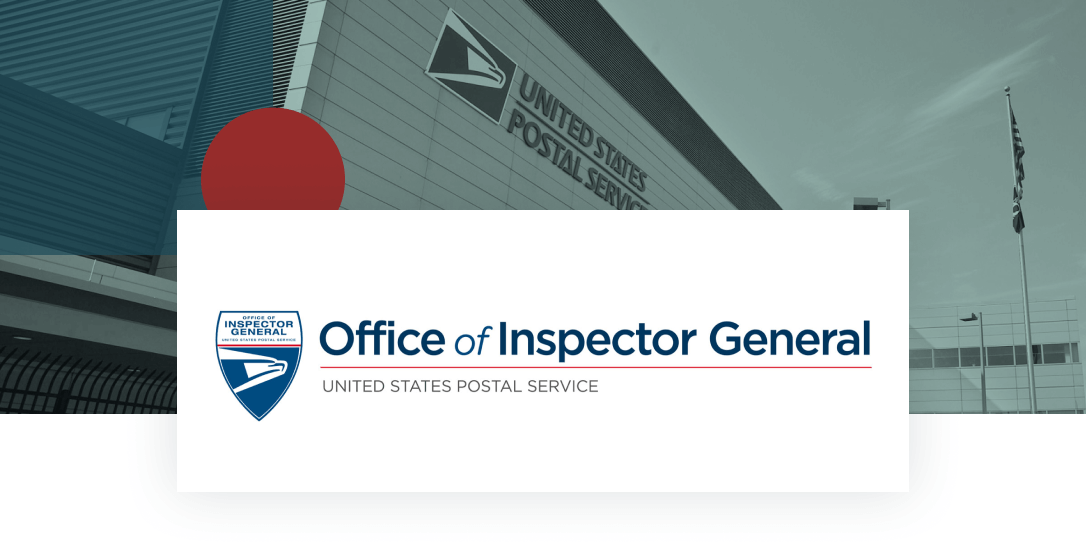
Customer Story
Delivering integrity and efficiency for the U.S. Postal Service
USPS OIG supports efficient postal service to millions with Databricks
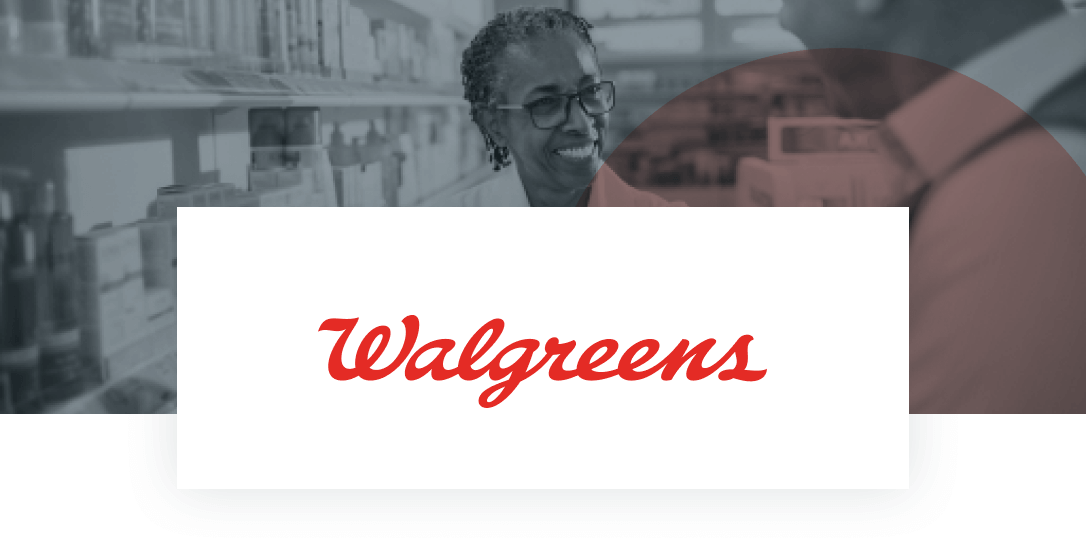
Customer Story
Walgreens personalizes pharmacy care to improve patient outcomes
Databricks helps Walgreens personalize patient experiences for over 825 million prescriptions filled annually
The Data Team Effect
Data teams are the united force that are solving the world’s toughest problems.
Be the next success story
Resources
Customer Story
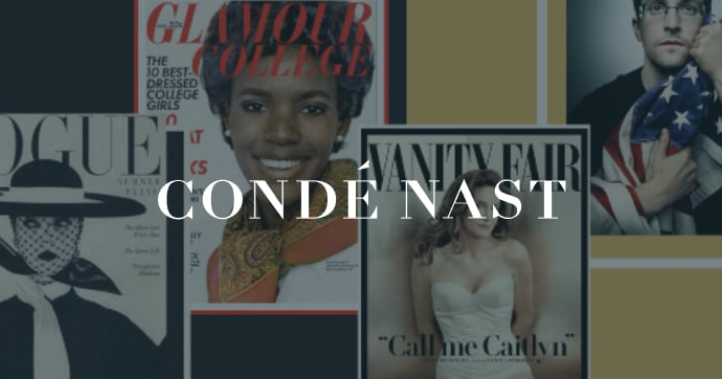
Learn how Databricks enables Condé Nast to deliver personalized content to its customers.
Webinar
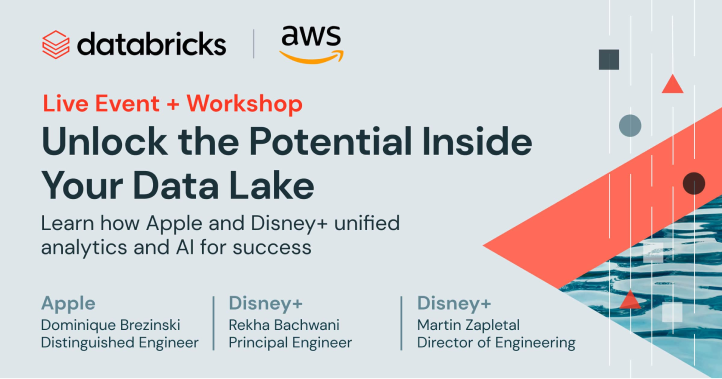
Learn how Apple and Disney+ unified analytics and AI for success
Podcast
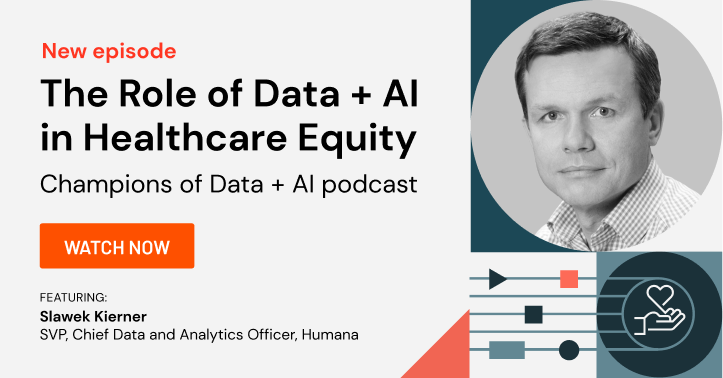
Hear about the role of data and AI in healthcare equity from the CDAO at Humana