Delivering AI-powered entertainment that captivates users for hours
Scatter Lab accelerates proprietary LLM development with Databricks Mosaic AI
To build a proprietary LLM with Mosaic AI
Secured in just nine months after launching
Of average weekly engagement on Zeta
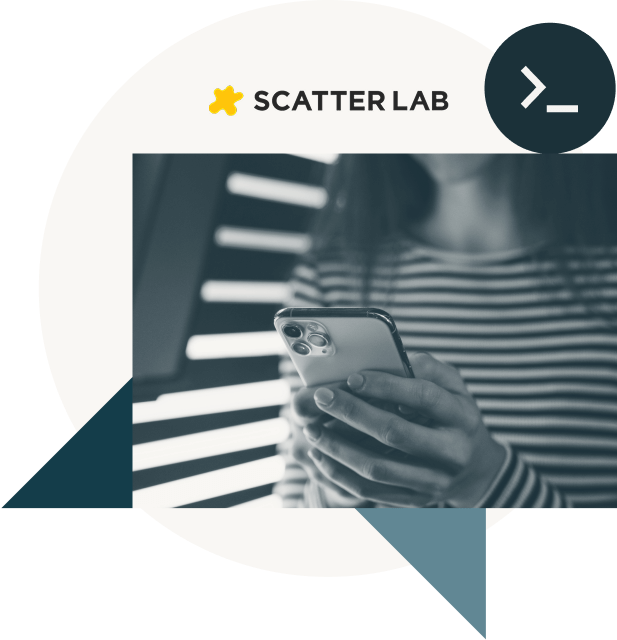
Scatter Lab, a conversational AI startup, is transforming entertainment with their AI-powered B2C platform. Since launching in 2023, their flagship chatbots, Iruda and Nutty, have driven 2 million downloads and 1.1 billion conversations. Their latest innovation, Zeta, blends AI-driven storytelling, character customization and immersive engagement to enhance chat experiences. However, development budget constraints posed a challenge. With Databricks Mosaic AI, Scatter Lab built a large language model (LLM) in just three months, fueling rapid growth — 1.5 million users within nine months, each spending over 12 hours per week on Zeta. Now, the company is poised for global growth, including expansion into Japan.
Facing the challenges and realities of developing a proprietary LLM with limited resources
Instead of adopting a widely available LLM, Scatter Lab chose to build a proprietary LLM, which allowed them to develop chatbots that have more empathetic and human-like conversations, ensure research freedom and data security and sustain operational cost efficiency and reliability. First, Scatter Lab decided that if they wanted to create an AI character that could have fun conversations, adopting traditional, ChatGPT-style conversations wasn’t the way to go. They also found that utilizing the GPT API limited their ability to implement custom features, such as multimedia conversations (e.g., images and emojis). This led to the need for a proprietary LLM to ensure research freedom and feature development.
In terms of data security, the robust data protection and privacy controls established by their legal team and external counsel could not be met by using an external LLM API. In addition, using the external API for conversational inference was expected to be costly. In particular, OpenAI’s API was not cost-effective because it counts one Korean character as one token, and it was not suitable for running large-scale services due to server stability issues. For these reasons, Scatter Lab decided to build their own LLM that can ensure conversation quality, research freedom, data security, cost-effectiveness and operational reliability.
However, as a startup, building their own LLM presented a number of practical constraints. Scatter Lab had a large amount of Korean-language data, but lacked the GPUs, experience and know-how required to train large language models. The budget was also quite limited, at less than 1 billion KRW, compared with the typical cost of training a large language model.
Scatter Lab requested a minimum of 1,024 A100 GPUs from multiple cloud service providers to secure GPUs, but was told that they were unable to supply this amount of GPUs due to increased global demand. The company also lacked the experience to address possible obstacles during language model training. If the company failed to build the model, there was a high risk of stagnation in technology development and lost opportunity cost. For example, in a development using a 30B model, two failures could have resulted in a loss of approximately 1.4 billion KRW or more. As such, Scatter Lab was in a desperate situation where they needed to succeed in just one attempt.
Built a proprietary LLM with Databricks Mosaic AI
To overcome these limitations, Scatter Lab adopted Databricks Mosaic AI solution. Databricks offered GPU clusters flexibly, allowing Scatter Lab to reserve and use GPU clusters on an hourly basis, as opposed to the six-month subscription commonly required by other cloud service providers. Databricks also addressed resource scarcity with multiple GPU options, including the A100 and H100, and thousands of dedicated GPU clusters for LLM training.
In addition, Databricks has a wealth of LLM training experience and know-how from working with dozens of clients, providing hyperparameters optimized for data size and model scale, and supporting the latest and fastest training scripts. Databricks’ 24/7 response and monitoring system checks training progress in real time and supports automatic recovery in case of failure, ensuring a stable environment for continued training. In particular, Databricks has demonstrated accumulated training know-how and the reliability of resources with open source trained models.
“Databricks was the only solution that met our needs for GPU resources, training know-how and cost-effectiveness, all of which are essential to building a high-quality LLM,” Jongyoun Kim, CEO of Scatter Lab, said.
Scatter Lab has built their own LLM based on the Databricks Mosaic AI solution, enabling them to provide users with AI services that have excellent storytelling and conversational skills. The AI chatbot, powered by the proprietary LLM, is able to synthesize various factors, such as the context of conversations, the personality of characters and the user’s background, to carry on more natural conversations with users, allowing for a more immersive experience.
Built LLMs of various sizes in just three months
By leveraging Databricks’ GPU resources and optimized training system, Scatter Lab overcame the challenges of limited budget and resources and was able to build proprietary LLMs of various sizes in just three months.
Scatter Lab’s “Zeta has surpassed 1 million user-created characters and currently has 1.5 million users in Korea alone. In Japan, the new service has a total of 150,000 users. In particular, users are using Zeta for about 12 hours a week or more. Junseong Kim, Strategy Manager at Scatter Lab, said, “The combination of Scatter Lab’s vast amount of high-quality data and Databricks’ optimized training environment allowed us to build our own LLMs. The collaboration between Scatter Lab and Databricks proved that startups can build their own LLMs efficiently and cost-effectively.”
Moving forward, Scatter Lab expects to work with Databricks to operate their own LLM-powered AI B2C platform and drive continued growth, including updates to new backbone models, multi-language support and fine-tuning with user data.