Digitally optimizing energy assets for decarbonization and cost savings
Reduction in time spent on manual forecasting
Savings per year due to energy optimization predicted
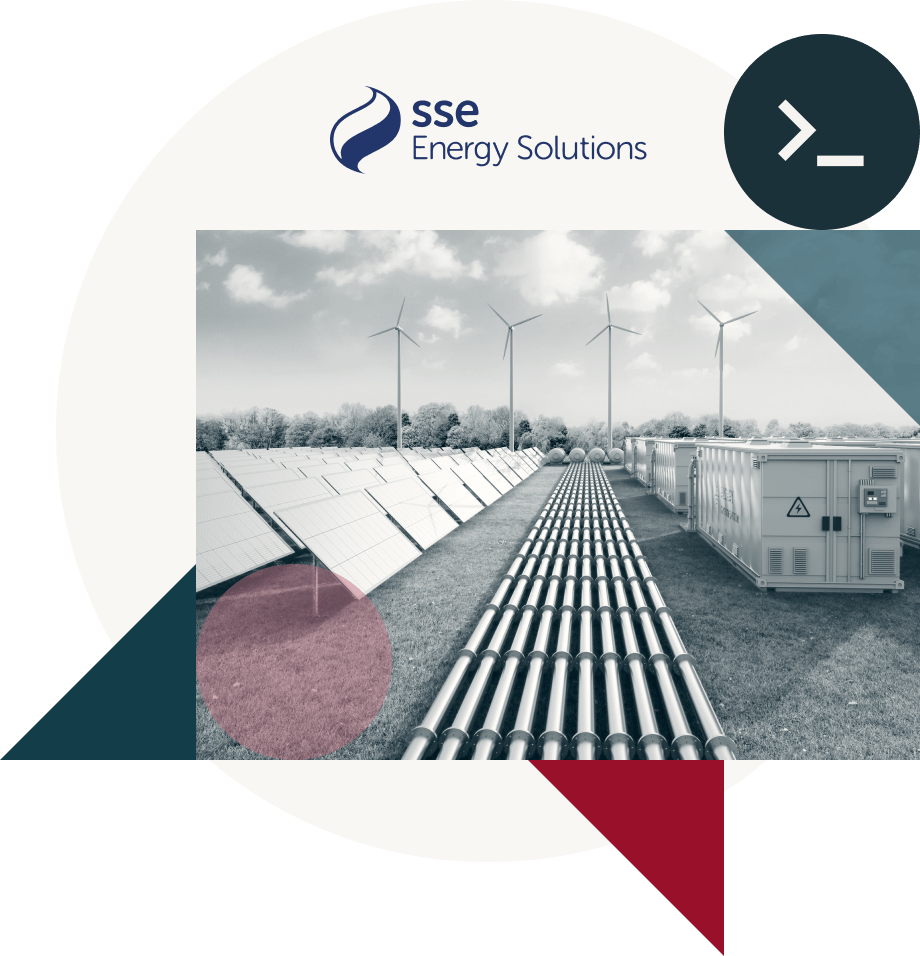
The drive for renewable energy and the mission to reach net-zero is decentralizing the generation of power. The energy generation mix of businesses is becoming increasingly complex, including onsite and offsite generation, battery storage and importing energy from the grid, as well as exporting to the grid at the most optimum time. As a renewable-focused energy company committed to reducing carbon emissions, SSE Energy Solutions recognizes the importance of the application of data and analytics in managing this mix, harnessing energy data for a range of purposes from assessing usage and forecasting future energy demand to measuring the output of renewable generation and benchmarking the consumption of green energy. But the use of virtual machines to optimize the machine learning (ML) workflow was not sustainable. With Databricks now part of the solution, SSE Energy Solutions can regularly run their optimizations, scaling the solution and helping to ensure their combined heat and power (CHP) systems run more effectively, with considerably reduced time, effort and associated costs.
Use of VMs for ML optimization proves to be manually intensive, costly
SSE PLC is a net-zero focused energy company that generates, transmits, distributes and supplies gas and electricity across the UK and Ireland. Sitting within SSE Energy Solutions, a division of the company that invests, builds and operates distributed energy infrastructure, SSE Digital Services has a dedicated data and analytics team. Individual data scientists, architects, engineers and analysts work together to produce business value from data held by different units within the organization, supporting customers to manage the increasingly diverse mix of energy generation methods and, ultimately, reduce carbon emissions.
One of these uses cases was designed to reduce the costs and improve the efficiency of operating SSE Heat Networks’ CHP distributed networks and, by doing so, lower carbon emissions. Through detailed analysis, the team saw an opportunity to optimize their heat networks through the application of new digital twins, process optimizations and the use of machine learning techniques.
The team initially ran the ML-powered optimization models on virtual machines (VMs). “We quickly realized that each run required large amounts of manual input,” said Rachel Hassall, Head of Data and Analytics at SSE Digital Services. “It was a hugely inefficient use of our data scientists’ time.” SSE needed a solution that would enable them to scale the optimization of their CHPs more efficiently — and cost-effectively — than their VMs. That’s where Databricks came in.
Lakehouse architecture improves the speed of response and prediction of future behavior
The introduction of unified data and analytics in the Databricks Data Intelligence Platform means SSE Energy Solutions can now perform a number of tasks, such as scheduling optimization jobs and product pipelines, that it simply wasn’t able to when using VMs.
The team now runs an ML workflow optimization model on Azure Databricks. This feeds into the optimization of the asset which is done on the edge to optimize four of SSE Heat Network’s CHP-powered networks across the UK.
The benefits, in terms of accuracy, speed and efficiency, are manifold. The team takes advantage of Databricks to automate the ETL of historic and current pricing regime data from one end of the pipeline to the other, for instance. Among other things, this allows their data scientists to produce baselines by using different slices of historic data and the application of simple rules, and to create digital twins using historic demand data to simulate and predict future behavior. Furthermore, the use of clustering and classification techniques to simplify the optimization results allows the team to update the edge optimization framework for implementation.
“Perhaps most importantly,” said Hassall, “Databricks’ integration with PowerBI means the data can be fed directly into dashboards, allowing stakeholders to view and act upon the results of each optimization run in a much timelier manner than before, which saves a significant amount of carbon, along with time, effort and money.”
Enhanced data and analytics lead to optimization across the company
Hassall’s team now runs weekly optimization jobs on each of the company’s CHP network sites to ensure they are performing efficiently. Unlike previously, where the VMs were limited by the amount of compute available, they are now able to spin up and schedule multiple runs at the same time. This not only represents valuable time savings but provides the team with insights for opportunities to optimize for carbon reduction.
Forecasting future demand is more efficient, too. A job that once took a day a week now takes no more than two hours. And using Databricks has also decreased the time to release. Running jobs on clusters rather than just on VMs has enabled the team to increase the amount of optimization runs it can perform.
“It’s not just the CHPs,” said Hassall. “Working with Databricks means we’ve been able to optimize our own performance. By transitioning from VMs to the lakehouse architecture, we’re saving on internal resources and time. Instead of babysitting a VM to do a job that Databricks can do more easily, we’re using our time more wisely on data analytics. And, as we scale the transition across all of SSE’s data and analytics capabilities, we anticipate considerable savings in internal resources across the group.”
SSE Energy Solutions could once optimize their assets almost a year in advance. But the increased uncertainty of the increasingly complex energy generation mix, coupled with current geopolitical unrest and the change in residential and commercial consumption behavior due to the pandemic, means the company has had to move to week-ahead optimization.
“Using Databricks is a very important part of this move,” said Hassall. “It means we can run our processes and optimizations efficiently every week. Looking ahead, we see this as a test case, an MVP for a broad optimization framework under which we scale the optimization on hundreds of different types of energy assets.”