Building an operationally efficient omnichannel business
in savings due to improved productivity
reduction in compute costs
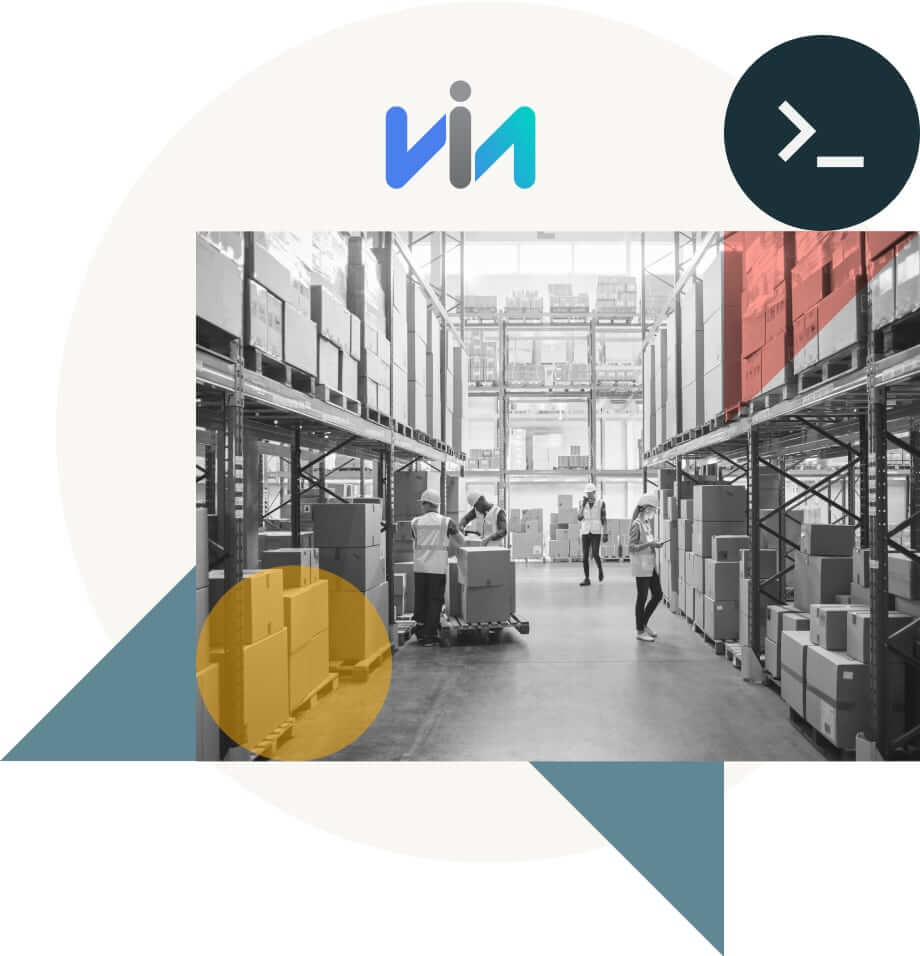
Brazilian customers rely on Via (formerly Via Varejo) for a wide selection of goods and services — from consumer electronics and home appliances to furniture and credit cards. But with decades of siloed data influencing different business decisions and a Hadoop data warehouse that was complex and didn’t foster ML innovation, Via struggled to accurately forecast demand, which impacted their entire supply chain. By unifying data and analytics on the Databricks Data Intelligence Platform, Via was able to develop a scalable architecture that enables data access throughout the organization. This enabled faster time-to-market to operationalize machine learning and provided insights that surface critical consumer trends to optimize business operations, prevent fraudulent activities and create a more targeted retail experience across touch points.
Bringing together decades of disconnected data
Consumers have been shopping with Via, a major Brazilian retailer with nearly 100 million customers, for over 60 years. But with decades of misaligned data and siloed channels impacting how decisions had been — and were still being — made across the organization, Via’s data team struggled to piece together a complete picture of consumer demand to shape business operations and supply chain considerations.
Due to the longevity and nature of the business, Via had many traditional transaction environments, including a mainframe built on Hadoop. Over the years, they had consolidated this with a Teradata data warehouse that was complex to manage and unable to support the needs of their data scientists, forcing them to build and train models on individual laptops. Without the ability to collaborate and scale training against their entire data set, data scientists could not wholly assess insights in context with related information.
“Collaboration was a big pain point, and so was scalability,” said Cezar Steinz, Manager of MLOps at Via. “We needed to train our models with complete data. That can be more than 24 billion rows of information. It’s impossible to train those kinds of models on a laptop.”
This led to decreased accuracy in analysis, which destabilized everything from the customer journey to fraud prevention — eventually undercutting revenue and slowing growth. Via realized that in order to fully empower their data scientists and analysts, they needed not only a common data layer, but also the ability to efficiently and collaboratively operationalize their data to help with supply chain optimization, demand forecasting and more. To build a unified data structure and streamline analytics and ML, Via turned to Databricks.
Data governance, fraud prevention and consumer demand
The Databricks Data Intelligence Platform on Azure has enabled Via to access insights that lead to better business decisions — including calculating churn, determining the next best offer or action (NBO/NBA), detecting and mitigating fraud, and developing pricing models that drive conversions and finance services (credit personalization and other payment methods).
With the lakehouse architecture, Via now has a common view of their data for analytics and ML. Delta Lake is used to provide data consistency and reliability as the company builds ETL pipelines to feed BI dashboards via integrations with Power BI as well as support the training of ML models. More importantly, they are able to deliver with high performance and confidence, which is paramount to meeting their daily decision-making needs.
“Databricks gives us better data governance,” said Steinz. “With Delta Lake, an analyst can access the exact data needed to address the specific use case at hand — if we’re talking about a logistics carrier, for example, we access just the relevant logistics data tables. It’s ideal that we can share specific data with the right people.” Delta Lake also offers Via better traceability so that they can have a better understanding of how their data is being used and whether they can reuse some of their data in different ML models.
Feature Store, a centralized repository of features, has provided Via’s data scientists and analysts with the ability to easily share and discover features. This has allowed them to truly unify not only their data, but also the teams that are accessing and using the data to make better and smarter decisions for the business. “Databricks Feature Store enables us to create a robust and stable environment for creating and reusing features consumed by models,” said Steinz. “This has enabled our data scientists and analysts to be more productive, as they no longer have to waste time converting data into features from scratch each time.”
With their data centralized in the lakehouse, their analysts use Databricks SQL to quickly analyze data and share insights with the rest of the business through Power BI dashboards and reports. And with MLflow, Via’s data scientists are able to easily manage the entire ML lifecycle — from tracking model versions to running experiments and reviewing results. “Creating a project with MLflow is of paramount importance, as it makes it feasible to package a model for us to run on any platform,” explained Steinz. “This means we are no longer limited to how we deploy ML to influence our business.”
Data democratization and streamlined ML models
With data performance humming and data science working with all their data, Via has been able to rapidly develop and deploy ML models that help with churn prediction, product recommendations, fraud and credit analysis, and customer behavioral analysis. These various use cases have allowed Via to deliver a shopping experience that is secure and highly targeted to meet the needs of their fickle customers — resulting in increased conversion and customer lifetime value. On the analytics front, a unified view into their data gives them the ability to extract insights that help guide strategies from streamlining supply chain operations to opportunities for new product innovations that delight their customers.
From an operational perspective, Databricks has helped reduce compute costs and boost cross-team collaboration. Across the entire data organization, Via has realized a 30% increase in productivity, resulting in an estimated total savings of R$ 3.9 million. With the help of Feature Store and automated cluster management, they have also seen a drop in data processing costs of 25%.
“Our data department has grown exponentially as we meet business expectations with fast, consistent and high-value deliveries,” expressed Steinz. “This is only possible because of the Databricks Data Intelligence Platform and the experts behind it.”
Looking ahead, with the democratization of data deeply ingrained in their culture, Via is well prepared to achieve their mission to make their customers’ dreams come true by leveraging data and AI to deliver the best possible buying experience.