Data governance strategy
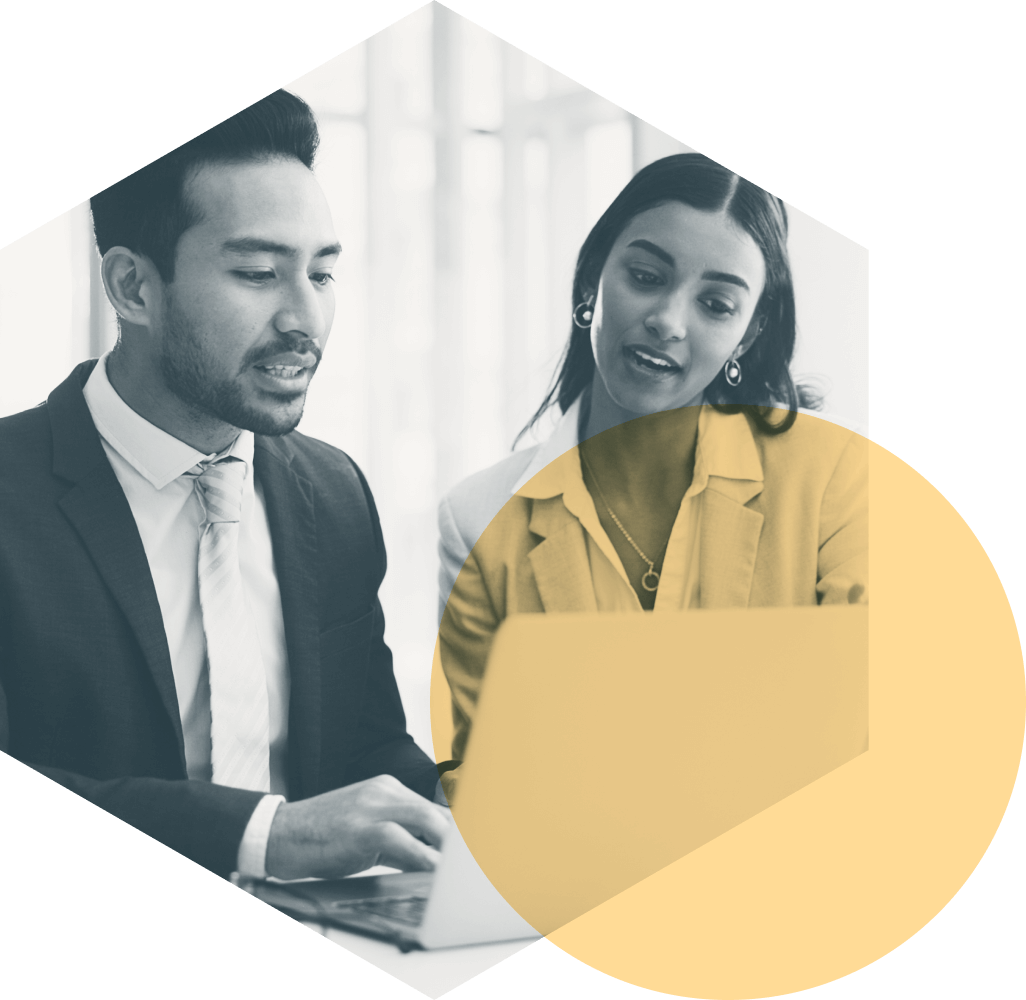
When data requirements are aligned with business strategy, data governance provides the data management, quality, visibility, security and compliance needed to make data-driven decisions while democratizing data access and analytics across the organization and beyond.
A data governance strategy clearly defines the policies and procedures to determine how data is collected, stored, accessed and used. This helps to ensure compliance with data protection regulations and reduces the risk of data breaches and other dangers associated with the use of data.
This guide details the steps and considerations in implementing a robust data governance strategy and the value it brings in democratizing the power of data for everyone in an organization.
What is a data governance strategy?
A data governance strategy is a comprehensive approach that comprises the principles, practices and tools to manage an organization’s data assets throughout their lifecycle. The strategy defines the tasks undertaken every day to ensure the organization can use, share and protect its data.
A comprehensive data governance strategy will specify policies for processing and sharing data and establish the processes by which it will be named and stored. It will also determine who is responsible for implementing these processes and policies, and who is responsible for which data domains.
The best data governance strategies build a framework through which the people in an organization are connected to its processes and technology. It should establish the processes, standards and documentation structures for collecting and managing data within the organization.
Here’s more to explore
Why is data governance strategy important?
A strong data governance strategy leads to data that is cleaner, more consistent and ultimately more usable. It enables organizations to draw accurate conclusions and make informed business decisions, secure in the knowledge that they are based on solid evidence.
The strategy helps define the data team’s data management practices, which become the technical implementation of data governance. A solid strategy will break down departmental silos, making data more readily available across business units in a shared language. This will foster better collaboration and allow more value to be extracted from data across the business.
A robust governance approach allows organizations to confidently leverage their data assets to gain competitive advantage and gain customer trust. Moreover, a data governance strategy focuses on one or more of these operational benefits:
Improved productivity and faster decision-making
Data governance promotes data democratization by ensuring data accuracy, consistency and trustworthiness. It helps data users find high-quality data quickly, promoting a better understanding of the data’s meaning and context, leading to increased productivity and faster decision-making.
Increased operational efficiency and reduced costs
Effective data governance allows organizations to create a single source of truth for their data estate, preventing data sprawl and silos and reducing duplication. This leads to increased efficiency, reduced costs and easier management of security and governance concepts across the data estate.
Enhanced collaboration and value realization
A strong data governance program lays the foundation for enhanced data collaboration and sharing across teams, business units and partners. This helps organizations promote knowledge sharing and build a better data culture, leading to increased innovation, better decision-making and maximizing the value of their data.
Enhanced security and privacy
Data governance mitigates security and privacy risks by implementing controls and processes to prevent unauthorized access and misuse of sensitive data. It promotes a culture of trust and transparency with stakeholders.
Better compliance with regulations and standards
Effective data governance results in better compliance with regulatory requirements such as HIPAA, FedRAMP, GDPR or CCPA. This protects the organization’s reputation, avoids potential financial and legal consequences and increases stakeholder trust.
Steps in creating a data governance strategy
A data governance strategy guides the best use of an organization’s data throughout the entire data lifecycle. It takes the business objectives into account against a host of operational considerations and plans for how the following steps/tasks will be achieved:
Set data governance objectives
Clearly define the goals and priorities of the data governance strategy, which, in turn, should align with the overall business objectives. For instance, one goal might be to improve data quality for better decision-making, which is directly tied to the organizational goal of value creation in a competitive market. A related goal might be to make the data more accessible and actionable to improve efficiency and productivity to support compliance and reporting for the organization’s sustainability goals.
Secure the go-ahead from the senior executive team and data governance committee
To ensure a data governance program is uniform across the entire organization, those at the very top need to be involved and support any data governance initiative. It’s important to highlight the benefits of a strong data governance strategy and stress the importance of putting processes around it to maintain consistency rather than creating information silos.
Set up a data governance committee to create the relevant policies and procedures. A data governance strategy only works if it’s adopted by the entire organization. A strong communications strategy can ensure everybody is on the same page about data governance, and that the strategy is upheld in every department.
Hire or train essential staff members
Executive support is crucial, but it’s equally important to gain bottom-up support and the best way to achieve that is by improving the data literacy and skills of all users. Identify key stakeholders and roles for the data governance program and who will need to be involved in its execution, including the data owners, administrators and data stewards who are responsible for the day-to-day running of the data governance workflows. Improved data literacy gives users the knowledge to find and reuse existing data, reducing duplicated instances of reports, spreadsheets and databases.
Identify all sources of data
Effective data governance requires knowledge of the data that exists within an organization. This is where a data catalog comes in, as it provides a centralized metadata repository for an organization’s data assets. It acts as a searchable index of all the data available, including information about its format, structure, location and usage, providing semantic value to an otherwise unidentifiable sea of information.
Look at what data assets you have and who oversees them and use this information to start building a directory data list. When you’re finished, you’ll have a comprehensive list of data assets, roles and accountabilities.
Prepare metadata for the data and organize a metadata storage option
Strong metadata management will help in understanding the data definitions of the information assets and will improve their findability and usability.
To properly govern enterprise data assets, it’s essential to clean and organize the metadata for data visualization and feature engineering. Data cleansing, data integration and data migration are often steps to ensure that data is compliant with the new strategy, and any transformations made to data going forward should follow the new framework. It’s also necessary to set up the data architecture to store and update the datasets efficiently for management, collaboration and analytics. Data storage includes the physical components that go into storing data, as well as the data security features surrounding it, and the access controls used to interact with it. A data lake architecture has proven more effective for storing metadata and building scalable, reliable data pipelines.
Establish a means of data distribution
A solid data governance strategy must include a secure and compliant means for everyone in your organization to locate data and unlock its true value. The emergence of modern data assets like dashboards, machine learning models, queries, libraries and notebooks has made data discovery a key pillar of a robust data governance strategy, as is the ability to share, collaborate and control how sensitive data is accessed and used. Open format, interoperable and multicloud data-sharing technologies are becoming essential to facilitate the discovery and distribution of datasets.
Track data lineage for data flow and usage
Data lineage is the ability to trace the lifecycle of data back to its origin. The ability to capture data lineage for all workloads is a fundamental capability for tracking sensitive data for compliance and to ensure data quality and get insights into how the datasets are being used, and by whom.
Scan for sensitive data in the data estate
Metadata can be used to capture detailed information about a dataset’s content, structure, usage, dependencies and permissions. It can also be used to identify potential data quality, compliance and security or privacy issues.
Create a governance framework
The governance framework defines processes and technologies for implementing a data governance strategy with a set of policies, procedures, best practices, data quality requirements, access controls, compliance standards, search and sharing controls, data lineage, monitoring and auditing requirements.
Check for compliance with relevant regulatory requirements
Meeting regulatory compliance requirements is perhaps one of the key drivers for data governance initiatives. Depending on the business or government domain, compliance can be a complex undertaking with a fragmented data estate without controls to meet the unique data requirements of regulated industries, which can include how data is stored, monitored, logged and shared. In the United States, the financial services and education sectors are subject to legislation dictating what types of inputs may be included in the development and training of AI/ML models.
Perform data quality checks
Data quality directly impacts the reliability of data-driven decisions and is a key aspect of data governance. Enforcing data quality rules helps trace data lineage and track changes. To maintain effective data governance, organizations must prioritize the evaluation of key data-quality attributes such as accuracy, completeness, freshness and compliance with data-quality rules. Have a rules library that is centrally managed and versioned. Update the rules library periodically with new rules.
Regularly audit data entitlements and access to ensure compliance
By understanding who has access to what data and tracking recent access, organizations can proactively identify overentitled users or groups and adjust their access accordingly, minimizing the risk of data misuse. By understanding who has access to what data and tracking recent access, organizations can proactively identify over-entitled users or groups and adjust their access accordingly, minimizing the risk of data misuse and maintaining the trust of their customers.
Establish regular reviews
Set up a process for regular feedback from senior executives, the data governance committee, data owners, data stewards and relevant stakeholders. Decide how to measure the success of the data governance efforts and policies and consider who is responsible for measuring the success of the data governance strategy.
Start by tracking the accuracy, consistency, completeness and instances of duplication of your company’s data. Also track the number of people with access to a system, their permissions and how often they are used, paying particular attention to the number of analytics users as it might indicate increased data literacy.
Determine what metrics you’ll use to assess the success of your data governance strategy and monitor these consistently, making changes where necessary as the needs of your business change.
Establish a process for constantly adapting the governance model
A data governance strategy is always evolving. With new business demands come new datasets that may require different levels of control and security. New technologies and evolving technical roles require agility while implementing new policies and launching new data applications. Automation and machine learning features can help optimize how data owners extract value from enterprise data by streamlining processes at every stage of the data lifecycle.
The best data governance frameworks align with the needs of the business. As business needs change, update the data governance strategy to reflect the new goals.
A unified, open approach
With all that to consider when creating a data governance strategy, businesses need a unified view of their data estate and tools that simplify management and collaboration across teams, such as Databricks Unity Catalog, an open governance solution for data and AI built into the Databricks Data Intelligence Platform.
Unity Catalog promotes interoperability, simplifies access management and streamlines the onboarding process with a unified interface to define access policies on data and AI assets and consistently apply and audit these policies on any cloud or data platform. It harnesses the power of AI to automate monitoring, diagnose errors and uphold data and ML model quality.
Democratization of data, analytics and AI is at the core of data governance. It’s about making data accessible, and usable for everyone without compromising on quality or security. Employees, clients and customers should have full confidence that their information is kept in accordance with regulations. This blend of accessibility and security is at the heart of data governance.