Easily ingest and transform batch and streaming data on the Databricks Data Intelligence Platform. Orchestrate reliable production workflows while Databricks automatically manages your infrastructure at scale and provides you with unified governance. Accelerate innovation by increasing your team’s productivity with a built-in, AI-powered intelligence engine that understands your data and your pipelines.
“We’re able to ingest huge amounts of structured and unstructured data coming from different systems, standardize it, and then build ML models that deliver alerts and recommendations that empower employees in our call centers, stores and online.”
Related products
Trustworthy data from reliable pipelines
Built-in data quality validation and proven platform reliability help data teams ensure data is correct, complete and fresh for downstream use cases.
Optimized cost/performance
Serverless lakehouse architecture with data intelligence automates the complex operations behind building and running pipelines, taking the guesswork and manual overhead out of optimizations.
Democratized access to data
Designed to empower data practitioners to manage batch or streaming pipelines — ingesting, transforming and orchestrating data according to their technical aptitude, preferred interface and need for fine-tuning — all on a unified platform.
Build on the Data Intelligence Platform
The Data Intelligence Platform provides the best foundation for building and sharing trusted data assets that are centrally governed, reliable and lightning fast.
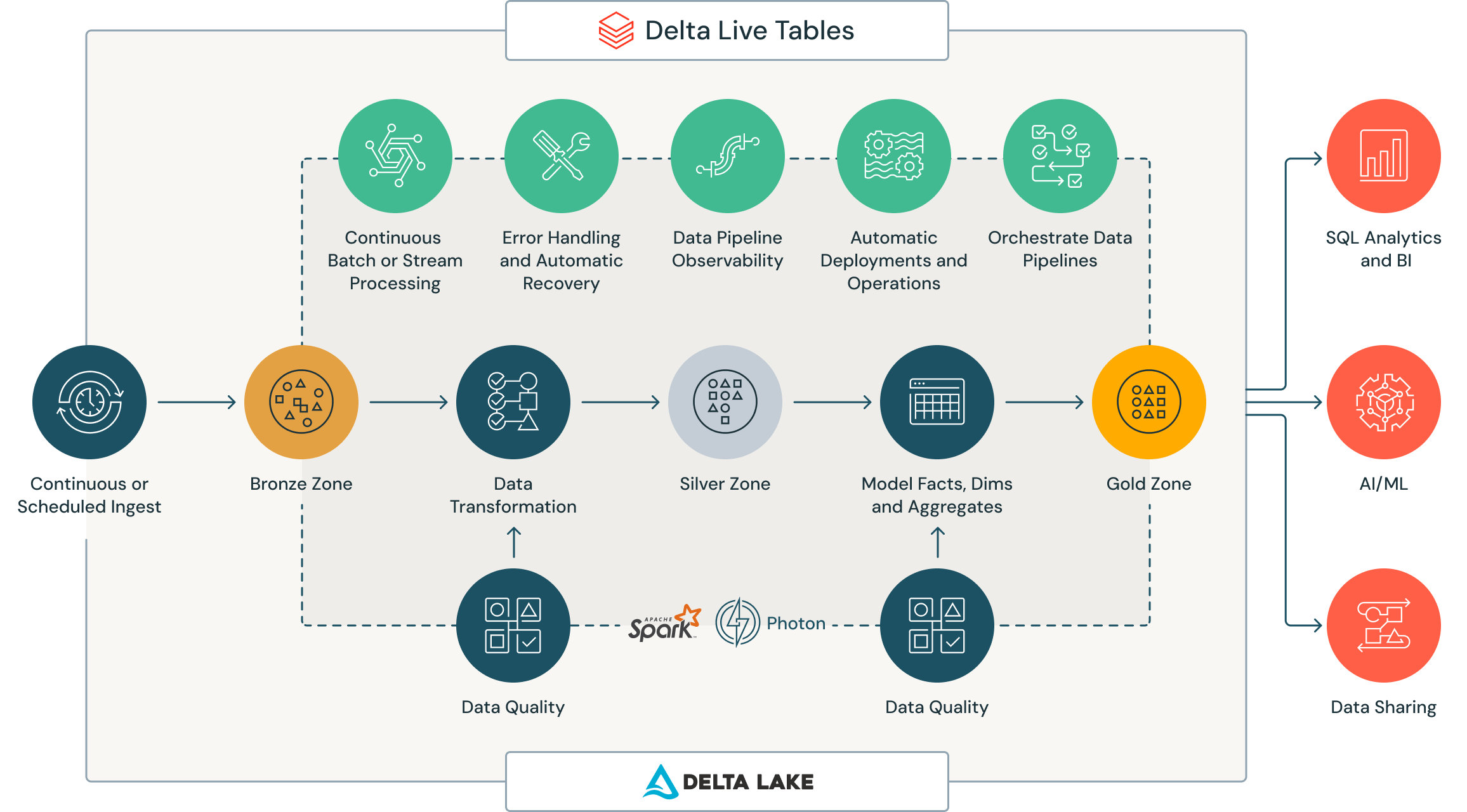
Managed data pipelines
Data needs to be ingested and transformed so it’s ready for analytics and AI. Databricks provides powerful data pipelining capabilities for data engineers, data scientists and analysts with Delta Live Tables. DLT is the first framework that uses a simple declarative approach to build data pipelines on batch or streaming data while automating operational complexities such as infrastructure management, task orchestration, error handling and recovery, and performance optimization. With DLT, engineers can also treat their data as code and apply software engineering best practices like testing, monitoring and documentation to deploy reliable pipelines at scale.
“[With DLT] the team collaborates beautifully now, working together every day to divvy up the pipeline into their own stories and workloads.”
— Dr. Chris Inkpen, Global Solutions Architect, Honeywell Energy & Environmental Solutions
Unified workflow orchestration
Databricks Workflows offers a simple, reliable orchestration solution for data and AI on the Data Intelligence Platform. Databricks Workflows lets you define multistep workflows to implement ETL pipelines, ML training workflows and more. It offers enhanced control flow capabilities and supports different task types and triggering options. As the platform-native orchestrator, Databricks Workflows also provides advanced observability to monitor and visualize workflow execution along with alerting capabilities for when issues arise. Serverless compute options allow you to leverage smart scaling and efficient task execution.
“With Databricks Workflows, we have a smaller technology footprint, which always means faster and easier deployments. It is simpler to have everything in one place.”
— Ivo Van de Grift, Data Team Tech Lead, Ahold Delhaize (Etos)
Powered by data intelligence
DatabricksIQ is the Data Intelligence Engine that brings AI into every part of the Data Intelligence Platform to boost data engineers’ productivity through tools such as Databricks Assistant. Utilizing generative AI and a comprehensive understanding of your Databricks environment, Databricks Assistant can generate or explain SQL or Python code, detect issues, and suggest fixes. DatabricksIQ also understands your pipelines and can optimize them using intelligent orchestration and flow management, providing you with serverless compute.
Next-generation data streaming engine
Apache Spark™ Structured Streaming is the most popular open source streaming engine in the world. It is widely adopted across organizations in open source and is the core technology that powers streaming data pipelines on Databricks, the best place to run Spark workloads. Spark Structured Streaming provides a single, unified API for batch and stream processing, making it easy to implement streaming data workloads without changing code or learning new skills. Easily switch between continuous and triggered processing to optimize for latency or cost.
State-of-the-art data governance, reliability and performance
Data engineering on Databricks means you benefit from the foundational components of the Data Intelligence Platform — Unity Catalog and Delta Lake. Your raw data is optimized with Delta Lake, an open source storage format providing reliability through ACID transactions, and scalable metadata handling with lightning-fast performance. This combines with Unity Catalog, which gives you fine-grained governance for all your data and AI assets, simplifying how you govern, with one consistent model to discover, access and share data across clouds. Unity Catalog also provides native support for Delta Sharing, the industry’s first open protocol for simple and secure data sharing with other organizations.
Integrations
Leverage an open ecosystem of technology partners to seamlessly integrate with industry-leading data engineering tools.
+ Any other Apache Spark™️ compatible client
Customers
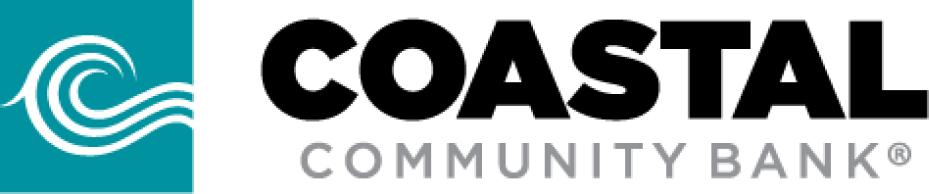
“Time and time again, we find that even for the most seemingly challenging questions, we can grab a data engineer with no context on the data, point them to a data pipeline and quickly get the answers we need.”
— Barb MacLean, Senior Vice President, Coastal Community Bank
“Delta Live Tables has greatly accelerated our development velocity. In the past, we had to use complicated ETL processes to take data from raw to parsed. Today, we just have one simple notebook that does it, and then we use Delta Live Tables to transform the data to Silver or Gold as needed.”
— Advait Raje, Team Lead, Data Engineering, Trek Bicycle
“We use Databricks Workflows as our default orchestration tool to perform ETL and enable automation for about 300 jobs, of which approximately 120 are scheduled to run regularly.”
— Robert Hamlet, Lead Data Engineer, Enterprise Data Services, Cox Automotive

“Our focus to optimize price/performance was met head-on by Databricks. The Data Intelligence Platform helped us reduce costs without sacrificing performance across mixed workloads, allowing us to optimize data and AI operations today and into the future.”
— Mohit Saxena, Co-founder and Group CTO, InMobi
FAQ
Data engineering is the practice of taking raw data from a data source and processing it so it’s stored and organized for a downstream use case such as data analytics, business intelligence (BI) or machine learning (ML) model training. In other words, it’s the process of preparing data so value can be extracted from it. An example of a common data engineering pattern is ETL (extract, transform, load), which defines a data pipeline that extracts data from a data source, transforms it and loads (or stores) it into a target system like a data warehouse.