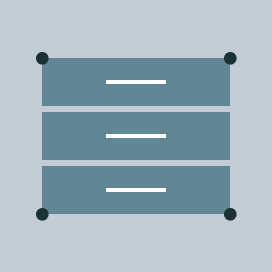
The top four companies by market cap today (MSFT, APPL, AMZN, GOOG) are all software companies, running their businesses on data. This is a radical departure from a decade ago. Enterprises in every segment are beginning to establish dominance through data. As a bank executive recently told me: “We’re not a finance company, we are a data company.”
Why is every company a data company (and every product a data product)?
The next wave of dominant companies in every segment will, underneath the covers, be data companies. This requires a data platform that drives the decisions of every employee and, just as important, powers data products. What are data products? A financial instrument, such as a credit card with a credit limit, can become a data product. Its competitive edge comes from crunching enormous amounts of data. Genomic sequencing is a data product. Finding life on Mars is a data product.
To enable the massive data transformation we talk about, you need to bring all your users and all of your data together. And then give them the tools and infrastructure they need to draw insights while following the enterprise security protocols. You need an Enterprise Data platform that scales across every department and every team. So why is that getting harder, not easier?
Your data has become more sensitive — The scale of data is increasing exponentially, yet it’s siloed across different systems in different departments. How do you make sure the right users have access to the right data, and it’s all monitored and audited centrally? And, at the same time, how do you stay in compliance with international regulations?
Your costs are difficult to control - Every organization is under pressure to do more with less. Exponential growth in data does not justify exponential growth in data infrastructure costs. When you have no visibility into who is doing what with what data, it results in uncontrolled costs — infrastructure costs, data costs and labor costs.
Data projects are difficult to manage - How do you track an initiative from start to finish when disparate teams — business analysts, data scientists, and data engineering — deploy disparate technologies managed by IT, Security and DevOps?? Which projects are in production? How are we monetizing them? What happens if an app goes down?
The complexities in going from small-scale success to enterprise-wide data transformation are enormous. A survey by McKinsey reveals that only 8% of enterprises have been successful at scaling their Data and Analytics practices across the organization1.
Executives need a holistic strategy to scale data across the organization
Enterprises new to these challenges may take an incremental approach, or take on-premises solutions and move them to the cloud. But without a holistic approach, you are setting yourself up for replacing one outdated architecture for another that is not up to the challenge long term. The following 5 steps can ensure you are progressing towards a system that can stand the test of time.
Step 1: Bring all your data together
Data warehouses have been used for decades to aggregate structured business data and make decisions by creating BI dashboards on visualization tools. The arrival of data lakes —with their attractive scaling properties and suitability for unstructured data — were vital for enabling data science and machine learning. Today, the Data Lakehouse model combines the reliability of data warehouses with the scalability of data lakes using an open format such as Delta Lake. Regardless of your specific architecture choices, choose a structure that can store all of your data — structured and unstructured —in open formats for long-term control, suitable for processing by a rapidly evolving set of technologies.
Step 2: Enable users to securely access the data
Make sure every member of your data team (data engineers, data scientists, ML engineers, BI analysts, and business decision-makers) across various roles and business units have access to the data they need and none of the data they’re not authorized to access). This means complying with various regulations, including GDPR, CCPA, HIPAA, and PCI).
It is important that all of your data — and all people that interact with it — remain together, in one place. If you are fragmenting the data by copying it into a new system for a subset of users (e.g. a data warehouse for your BI users) you have data drift, which leads to issues in Step 3. It also means you have drift of “truth”, where some information in your organization is stale or of a different quality, leading to (at best) organizational mistrust and (more likely) bad business outcomes.
Step 3: Manage your data platform like you manage your business
When you onboard a new employee, you set them up for success. They get the right computer, access to the right systems, etc. Your data platform should be the same.
Since all of your data is in one place, every employee can see a different facet of the data, according to their roles and responsibilities. And this data access needs to be aligned with how you manage other employee onboarding; everything must be tied to your onboarding systems, automated, and audited.
Step 4: Leverage cloud-native security
As cloud computing has become the de facto destination for massive data processing and ML, core security principles have been reformulated to Cloud-Native security. The DMZ and perimeter security of “on-premise” security are replaced with “zero-trust” and “software-defined networking.” Locks on physical doors have transformed into modern cryptography. So you must ensure your data processing platform is designed for the cloud and leverages best-in-class cloud-native controls.
Moreover, the cloud auditing and telemetry provide a record of data access and modification through the cloud-native tools, since every user accesses data with their own identity. This makes Step 3 possible - the groups that you manage your company with are enforced and auditable down to the cloud-native security primitives and tools.
Step 5: Automate for scale
Whether rolling out your platform to hundreds of business units, or many thousands of customers, it needs to be automated from the ground up. This requires that your data platform can be deployed with zero human intervention.
Further, for each workspace (environment for a business unit), data access, machine learning models, and other templates must be configured in an automated fashion to be ready for your business.
But powering this scale also demands powerful controls. With the compute of millions of machines at your fingertips, it is easy to run up a massive bill. To deploy to departments across the enterprise the right spend policies and chargebacks need to be designed to ensure the power is being deployed as the business expects.
APIs can automate everything from provisioning users and team workspaces to automating production pipelines, controlling costs, and measuring business outcomes. A fully automatable platform is necessary to power your enterprise.
Become the data company you must be
It is time to begin the journey to compete as a data company. Enterprises around the world are making this journey by placing Databricks at their core. A large modern bank uses Databricks to process 20 million transactions across 13 million end users every day to detect credit card fraud and many other use cases. They have been able to democratize data access, so 5000 employees can make data-based decisions on Databricks. One of the largest food & beverage retailers in the world operates over 220 production pipelines, with 667 TB of data and 70+ published data products on the Databricks platform. We are glimpsing the beginning of the data revolution in business and are excited to see where the road takes us from here.
Regardless of the platform choices you make, incorporate these five steps to ensure you are designing a platform that delivers for years to come.
Resources:
To learn more and watch actual demos sign up for the webinar
Learn more about each one of the steps in detail by reading the following blogs