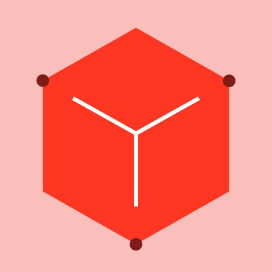
Data, Data Everywhere and Not an Insight to Consume
This is a guest post from Databricks partner Wipro. We thank Krishna Gubili, Global Head - Databricks Partnership, for his contributions.
In Samuel Taylor Coleridge's poem The Rime of the Ancient Mariner, sailors find themselves lost at sea. Although they're surrounded by water, they're parched because none of it is drinkable. Perhaps you've heard the most famous lines: "Water, water, everywhere, / Nor any drop to drink."
The plight of these sailors reminds me of the plight of many of today's business users. They find themselves surrounded by volumes of data they couldn't even fathom 30 years ago. But they can't use this data because they lack the right technology to extract its insights.
In the late 1990s, I was part of a team tasked with building a gigantic 25-gigabyte data warehouse for a large retailer. This retailer needed to gather insights on average basket size and number of trips - a true single view of the customers. Our team built a cool star schema and a huge data warehouse, and then celebrated our apparent victory. Our celebration ended when the client requested some statistical models around pricing and customer segmentation that wouldn't work on the schema.
We proposed another solution to our client: build an analytical data warehouse. I still have nightmares about the weekend data processing tasks. We had to complete all our data downloads from the mainframe in less than a day, and the data in both warehouses needed to be perfectly in sync. Every few weeks a job would fail, and all hell would break loose. The analytical data warehouse enabled this retailer to ingest different data sets from point-of-sale systems, sales tools, promotions and markdowns to one central database for the first time. At last, our client had a single view of the customer. But it was a hollow victory because they still needed another system to run the SAS-based statistical models that could deliver insights on customers.
The lesson learned? The data warehouse, as useful as it is, has always had its limitations.
Time passes, but challenges remain
Fast forward 25 years to the present day. The data warehouse is still omnipresent, but organizations that want to store structured and unstructured data in one place and get outstanding processing speed need to focus on deploying data lakes, too.
For example, a large financial organization recently hired us at Wipro to deploy a data warehouse on a leading cloud data warehouse platform. We also needed to build customer acquisition models by integrating internal and external data. Although this organization is in the lending business, its sales and loan servicing departments lacked a single view of the customer. As a result, the company struggled to drive revenue through cross-selling and upselling.
We soon discovered it would be too complex and expensive to run customer acquisition models on the legacy data warehouse platform we were using. So we migrated everything to a cloud data warehouse that would support artificial intelligence and machine learning. That's when we discovered we would need to pull the data out of the data warehouse to run the models. To make a long story short, we ended up augmenting our cloud data warehouse with an analytics zone and a data lake.
"Data, data, everywhere, nor any insight to consume"
Notice the pattern here? For decades, data teams have craved technology that could combine the flexibility, cost-efficiency, and scale of data lakes with the data management and transactional capabilities of data warehouses. Even after so many years, most organizations around the world still struggle to move data between platforms, manage redundant pipelines from source systems, and create separate platforms to meet needs ranging from business analytics to data science.
Along the way, we've somehow become obsessed with dumping data into lakes and ponds and migrating it into and out of clouds. Meanwhile, business users feel like the ancient mariner as they complain, "Data, data, everywhere, nor any insight to consume."
Combining the best of data warehouses and data lakes on one platform
Just when I was losing hope, I found the answer: the Lakehouse, a single platform that can support all data types and data workloads. This means data engineering, data analysis via SQL, and AI/ML workloads can all run on a simple, open and multicloud platform. The Databricks Lakehouse is built on scalable open source Apache Spark, Delta Lake and MLflow. By offering the best of the data warehouse and data lake without the drawbacks, the Lakehouse helps organizations work more quickly because they can use all their data without accessing multiple systems. Having the Lakehouse while working on my retail project would have been more beneficial to what the retailer was asking, but also more beneficial to my stress and anxiety.
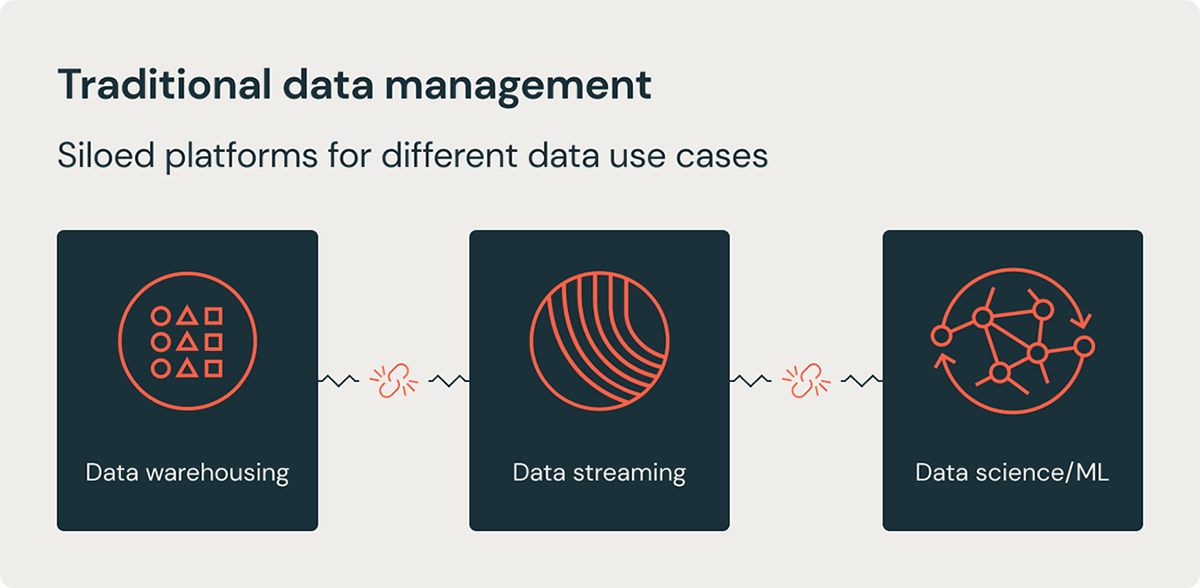
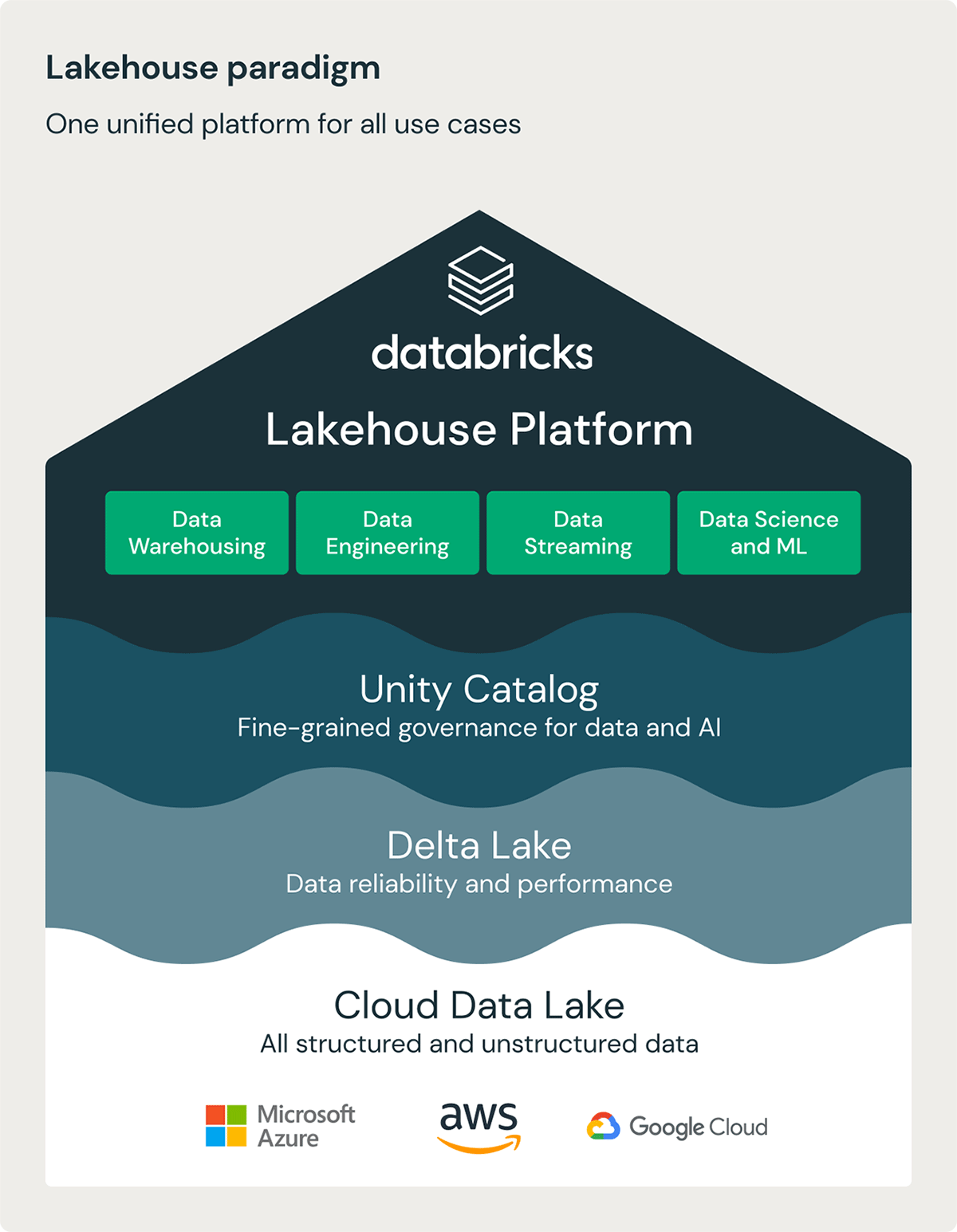
Client example of the benefits of migrating to the lakehouse
Wipro has successfully migrated many large corporations to Databricks Lakehouse Platform. A most recent example is from a larger surgical and medical device manufacturer. After their migration to Databricks, the client was able to improve supply and demand planning, enhance customer experience and drive and develop new products based on profitability and revenue growth. Their technology benefits included:
- Decreased data availability lag by 52%
- Reduced operating costs by more than 45%
- Reduced the warehouse loading time by 60%, from 8 hours to 1 hour and 45 minutes
- Reduced software costs by 50%
Wipro partners with organizations around the world to leverage Databricks in ways that create business impact. The company's proven Hadoop migration tools and frameworks help companies migrate more quickly from big data platforms to Databricks. Wipro is Databricks' AMER Partner of the Year for 2022. Wipro is a Leader in the Gartner and Forrester quadrants in data and analytics. For more information, please visit www.wipro.com