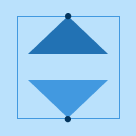
Last year, we launched foundation model support in Databricks Model Serving to enable enterprises to build secure and custom GenAI apps on a unified data and AI platform. Since then, thousands of organizations have used Model Serving to deploy GenAI apps customized to their unique datasets.
Today, we're excited to announce new updates that make it easier to experiment, customize, and deploy GenAI apps. These updates include access to new large language models (LLMs), easier discovery, simpler customization options, and improved monitoring. Together, these improvements help you develop and scale GenAI apps more quickly and at a lower cost.
Databricks Model Serving is accelerating our AI-driven projects by making it easy to securely access and manage multiple SaaS and open models, including those hosted on or outside Databricks. Its centralized approach simplifies security and cost management, allowing our data teams to focus more on innovation and less on administrative overhead - Greg Rokita, VP, Technology at Edmunds.com
Access New Open and Proprietary Models Through Unified Interface
We’re continually adding new open-source and proprietary models to Model Serving, giving you access to a broader range of options via a unified interface.
- New Open Source Models: Recent additions, such as DBRX and Llama-3, set a new benchmark for open language models, delivering capabilities that rival the most advanced closed model offerings. These models are instantly accessible on Databricks via Foundation Model APIs with optimized GPU inference, keeping your data secure within Databricks' security perimeter.
- New External Models Support: The External Models feature now supports latest proprietary state-of-the-art models, including Gemini Pro and Claude 3. External models allow you to securely manage 3rd-party model provider credentials and provide rate limiting and permission support.
All models can be accessed via a unified OpenAI-compatible API and SQL interface, making it easy to compare, experiment with, and select the best model for your needs.
client = OpenAI(
api_key='DATABRICKS_TOKEN',
base_url='https://<YOUR WORKSPACE ID>.cloud.databricks.com/serving-endpoints'
)
chat_completion = client.chat.completions.create(
messages=[
{
"role": "user",
"content": "Tell me about Large Language Models"
}
],
# Specify the model, either external or hosted on Databricks. For instance,
# replace 'claude-3-sonnet' with 'databricks-dbrx-instruct'
# to use a Databricks-hosted model.
model='claude-3-sonnet'
)
print(chat_completion.choices[0].message.content)
At Experian, we’re developing Gen AI models with the lowest rates of hallucination while preserving core functionality. Utilizing the Mixtral 8x7b model on Databricks has facilitated rapid prototyping, revealing its superior performance and quick response times." - James Lin, Head of AI/ML Innovation at Experian.
Discover Models and Endpoints Through New Discovery Page and Search Experience
As we continue to expand the list of models on Databricks, many of you have shared that discovering them has become more challenging. We're excited to introduce new capabilities to simplify model discovery:
- Personalized Homepage: The new homepage personalizes your Databricks experience based on your common actions and workloads. The 'Mosaic AI' tab on the Databricks homepage showcases state-of-the-art models for easy discovery. To enable this Preview feature, visit your account profile and navigate to Settings > Developer > Databricks Homepage.
- Universal Search: The search bar now supports models and endpoints, providing a faster way to find existing models and endpoints, reducing discovery time, and facilitating model reuse.
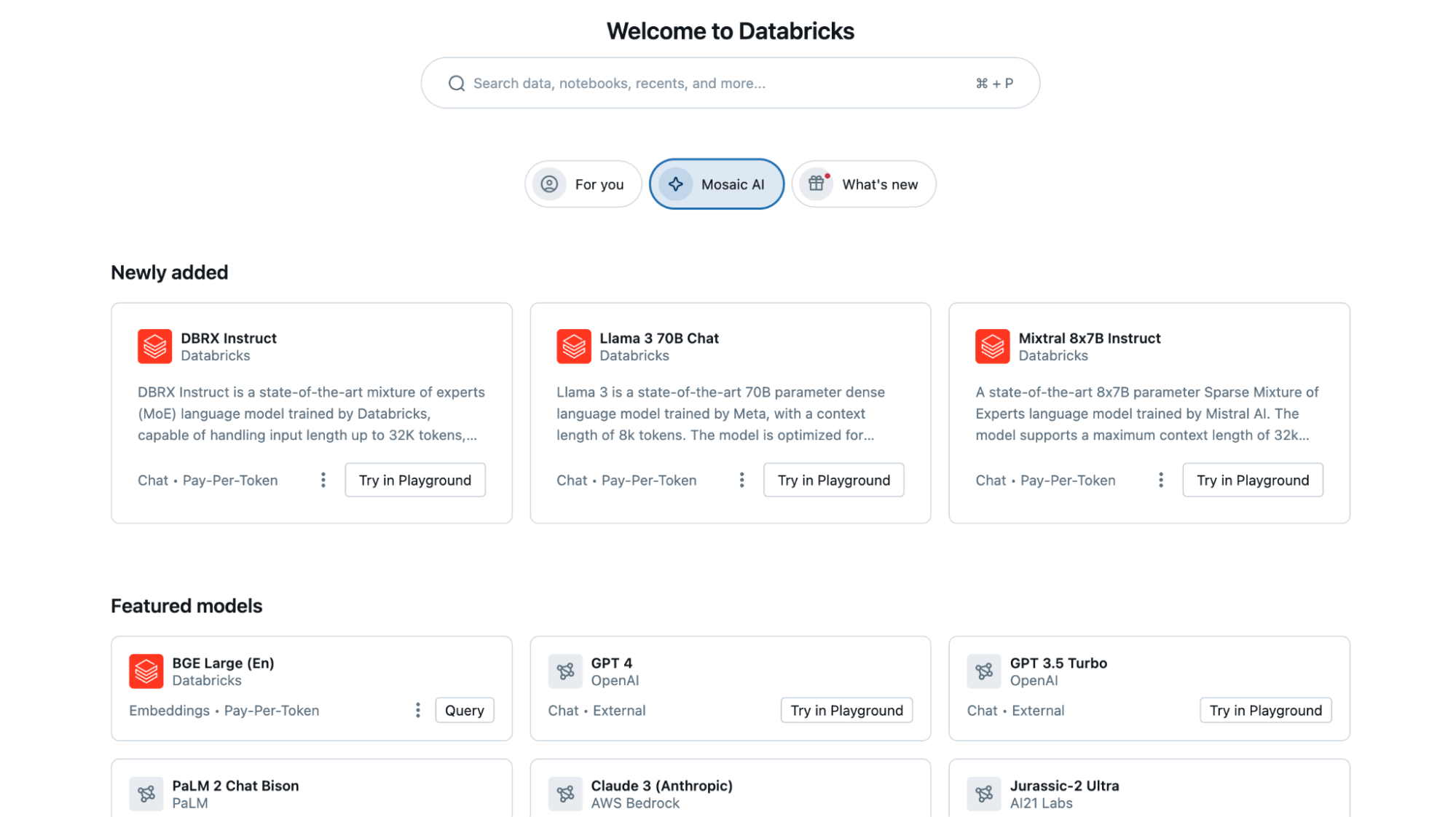
Build Compound AI Systems with Chain Apps and Function Calling
Most GenAI applications require combining LLMs or integrating them with external systems. With Databricks Model Serving, you can deploy custom orchestration logic using LangChain or arbitrary Python code. This enables you to manage and deploy an end-to-end application entirely on Databricks. We're introducing updates to make compound systems even easier on the platform.
- Vector Search (now GA): Databricks Vector Search seamlessly integrates with Model Serving, providing accurate and contextually relevant responses. Now generally available, it's ready for large-scale, production-ready deployments.
- Function Calling (Preview): Currently, in private preview, function calling allows LLMs to generate structured responses more reliably. This capability allows you to use an LLM as an agent that can call functions by outputting JSON objects and mapping arguments. Common function calling examples are: calling external services like DBSQL, translating natural language into API calls, and extracting structured data from text. Join the preview.
- Guardrails (Preview): In private preview, guardrails provide request and response filtering for harmful or sensitive content. Join the preview.
- Secrets UI: The new Secrets UI streamlines the addition of environment variables and secrets to endpoints, facilitating seamless communication with external systems (API is also available).
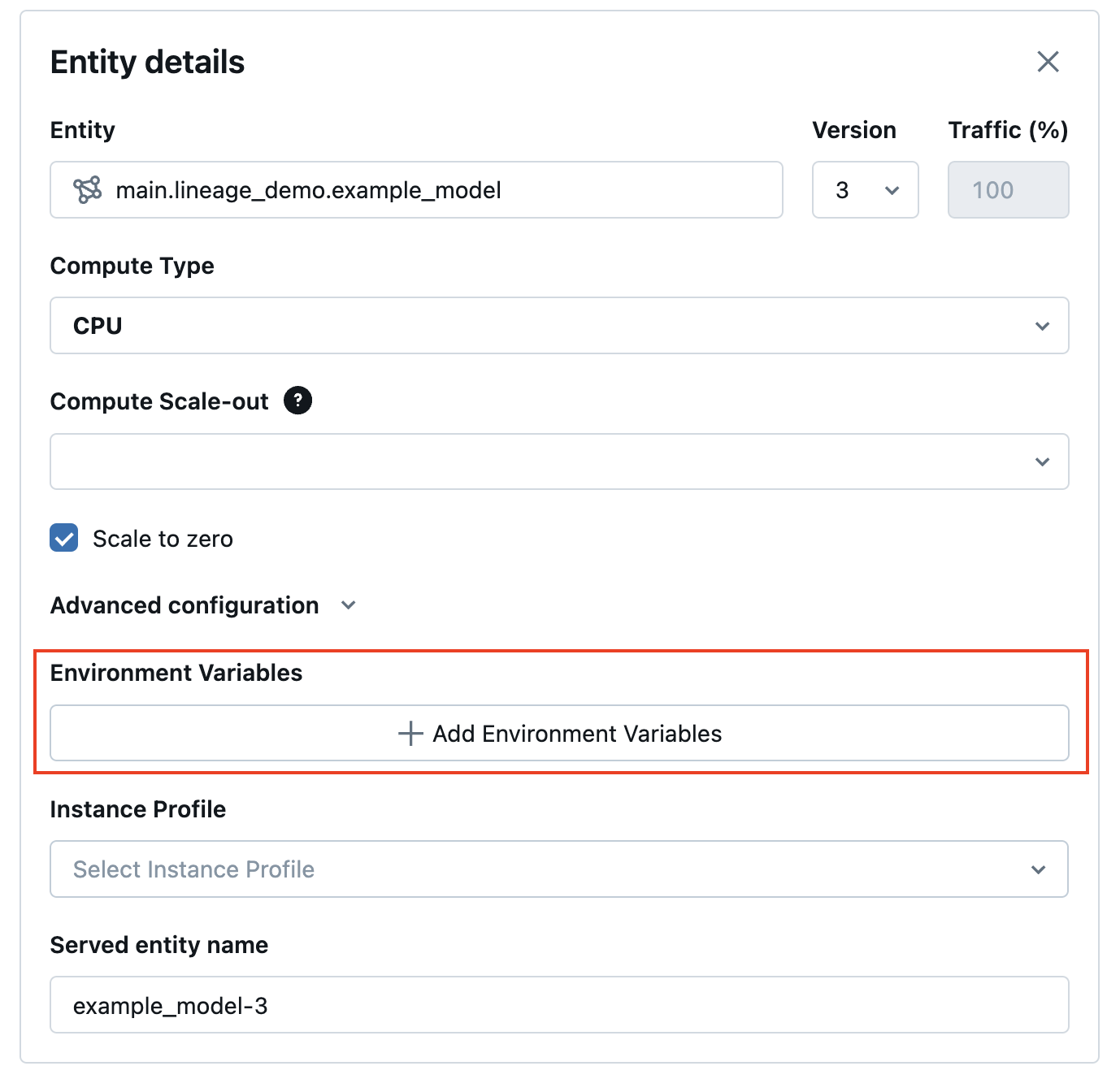
More updates are coming soon, including streaming support for LangChain and PyFunc models and playground integration to further simplify building production-grade compound AI apps on Databricks.
By bringing model serving and monitoring together, we can ensure deployed models are always up-to-date and delivering accurate results. This streamlined approach allows us to focus on maximizing the business impact of AI without worrying about availability and operational concerns. - Don Scott, VP Product Development at Hitachi Solutions
Monitor All Types of Endpoints with Inference Tables
Monitoring LLMs and other AI models is just as crucial as deploying them. We're excited to announce that Inference Tables now supports all endpoint types, including GPU-deployed and externally hosted models. Inference Tables continuously capture inputs and predictions from Databricks Model Serving endpoints and log them into a Unity Catalog Delta Table. You can then utilize existing data tools to evaluate, monitor, and fine-tune your AI models.
To join the preview, go to your Account > Previews > Enable Inference Tables For External Models And Foundation Models.
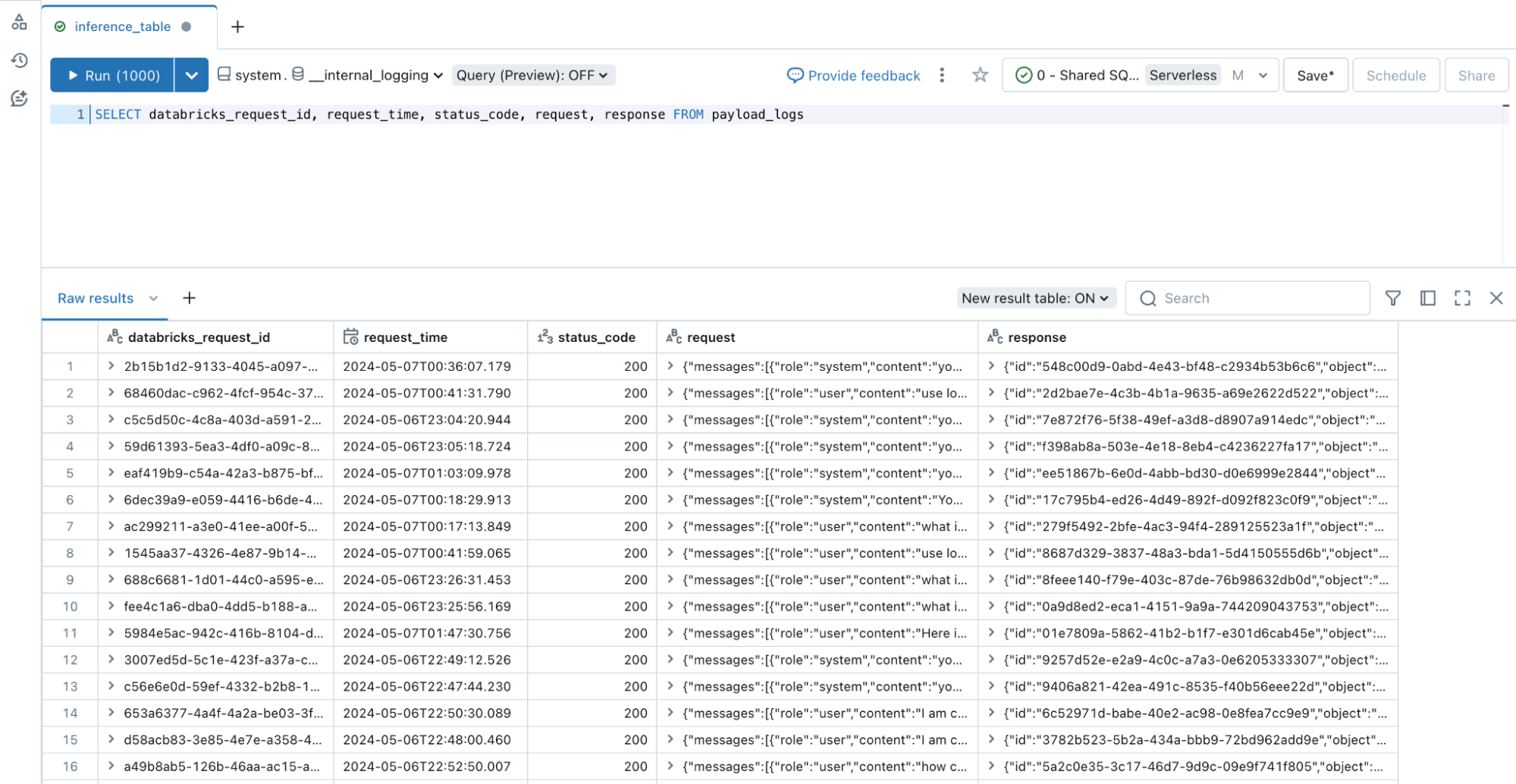
Get Started Today!
Visit the Databricks AI Playground to try Foundation Models directly from your workspace. For more information, please refer to the following resources:
- Explore the Foundation Model Getting Started Guide
- Join previews by going to Account > Previews
- Discover invoking LLMs from SQL using AI functions
- View the pricing page