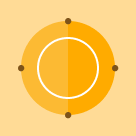
AI has quickly moved from an emerging technology to a business imperative as organizations recognize its potential to transform operations and keep them competitive. However, effective adoption of AI requires more than just bringing on new technology. To realize AI’s potential, you need a comprehensive AI strategy. This strategy, aligned with business objectives, provides the “big picture” your organization needs to make smart decisions and capitalize on AI’s capabilities.
What is an AI business strategy?
An AI business strategy lays out the approach that a business will take to implement and operate AI across the organization. In the process of creating an AI strategy, the organization can explore important questions such as “Why AI?” and “What does the organization need to fuel AI success?”
Technology and business objectives are central to any AI strategy, but it’s also important to address how AI will affect people. AI strategies should define ethical principles and provide guidance on how to minimize potential human-harming effects.
A robust AI strategy provides a blueprint specific to the organization’s needs and provides clear direction for AI implementation and operation. This framework guides how AI will be used to achieve the company’s most important long-term goals.
How does an artificial intelligence strategy add value?
AI is here to stay, and businesses are going to have to adopt it in one way or another to stay competitive. With a carefully crafted AI strategy in place, businesses can make sure they are taking an approach that fits their business and adopt tools that will have the right impact. A clear strategy helps to accelerate implementation, save time and money, manage risk, speed user adoption and achieve ROI faster. It also ensures that AI initiatives are not just reactive but proactive, driving value across the organization.
Building your AI strategy framework
Organizations don’t want to miss out on AI advantages, but taking the time to create an AI strategy is well worth it to create a foundation for optimizing AI’s advantages. We’ve outlined a process for building your AI strategy, step by step, to lay the groundwork for AI operations that can help transform your organization.
Define aims
Businesses are well aware that AI is a disruptive technology that can add immense value. But an AI strategy needs to start with your business, not the technology. This means looking beyond AI capabilities to first define what you want to accomplish with AI on a high level. Your AI objectives should be tailored to the organization’s unique needs and align with its broader strategic vision and values. Examples of strategic objectives include:
- Getting better insights to increase business impact
- Reducing risks
- Controlling costs
- Sustaining competitive advantage
- Driving innovation
Once you have the bigger picture in mind, you can focus on more specific use cases tied to challenges in the organization. Start by identifying problems and focusing on those where AI can provide solutions with significant value. Areas where AI can make a big impact include:
- Driving decisions with data
- Boosting efficiency and productivity
- Expanding the business model with new products and capabilities
- Reducing errors and increasing quality
- Offering better customer service
While AI solutions exist for a multitude of business functions, they don’t all provide the same value for your business. Knowing exactly where AI can make a real difference sets you up for choosing the right approach to help you meet your larger organizational goals.
Assess Data
Your company’s internal data is the raw material you can leverage to produce unique insights and innovation to propel your organization forward. It’s a treasure trove you can use to create and maintain competitive advantage. Impactful AI depends on quality data, so it’s critical to take a good look at the state of your data and systems as part of your AI strategy.
Ensure that your data is clean, accurate, and aligned with your AI objectives. High-quality data is the foundation for reliable AI insights. Data is at the heart of any AI system. Data quality affects the accuracy of AI outputs and determines the reliability of the insights generated. As a leader, you must ensure that your department prioritizes data readiness as a core aspect of its AI strategy development. Areas to examine include:
- Data quality: Your data should be able to support your AI objectives and use cases. Data needs to be clean, accurate, relevant and timely to optimize AI outputs.
- Collection and storage: It’s crucial to understand where your data comes from and how it’s stored and processed. Often, data is widely distributed across an organization, making it difficult to know which data is available or get it into the hands of the people who need it. Data infrastructure should support accessibility and AI readiness.
- Governance: Clear, robust data governance policies are key to ensuring security and regulatory compliance. Effective governance makes data accessible to the appropriate people while protecting sensitive information.
If your data assessment reveals gaps, your AI strategy should address them—updating systems, tools and processes if needed. Enterprises are increasingly looking to next-generation data infrastructures such as lakehouses, which can increase data and analytics accessibility, improve security and merge affordable storage with powerful querying capabilities.
Identify risks
While AI offers the potential for game-changing transformation, it can also create risks, both operational and ethical. Identifying these as part of your AI strategy allows you to take risks into consideration and minimize them as much as possible. Some common risks include:
Bias: Bias in models and/or data can produce biased outputs that can lead to discrimination or ethical issues.
Security: AI systems can be vulnerable to cyber threats. Robust, integrated security measures are critical to protecting valuable assets.
Transparency: Lack of transparency can lead to faulty, opaque or toxic insights that can negatively influence business decisions or jeopardize regulatory compliance.
IP: Sharing proprietary data with large third-party AI models can create risks for exposure of trade secrets, IP and copyrighted materials.
As AI matures, these risks may evolve, so constantly monitoring the AI risk landscape is central to any AI strategy.
Explore and assess technologies
Once you know the problems you’re looking to solve, the next step is exploring potential AI solutions. As you research, you’ll want to consider several factors, such as fit for your particular use case, accuracy and performance, cost, ease of integration, scalability and flexibility, ethical and compliance, and user experience.
One key consideration is whether to use open-source vs. third-party tools. Third-party vendors may have the biggest and most well-known large language models (LLMs), but these use general data from the internet and can pose risks in terms of privacy, IP protection, relevance and accuracy. Using an open-source model allows you to use your own data to produce AI results that are relevant to your specific goals, providing a strategic advantage while protecting proprietary data.
Once you’ve narrowed down your choices, create a proof of concept and test the solution’s real-life performance. This offers the opportunity to make an informed decision before fully committing to a particular technology.
Integration
Integration with existing systems and processes is key to successful AI deployment. Your AI strategy should outline how various elements will come together.
Key components to consider include:
Data: Data systems need to be flexible and efficient, with the infrastructure to optimize data value while ensuring safety and security. Many enterprises are moving toward consolidating data functions into one platform to optimize support for AI initiatives.
Algorithms: It’s important to plan how algorithms will integrate with existing systems, including data systems, ERP, CRM or other enterprise software.
Infrastructure: IT infrastructure must provide the computing power, scalability and flexibility needed for AI while supporting seamless integration of data and AI operations. Integrated governance structures for data and AI models are especially important for minimizing AI risk.
Making sure AI and other components work together is key for realizing maximum value from AI investments.
Monitoring and evaluation
Learning and growth are integral to AI. An effective AI strategy must address how the organization will keep track of AI performance and adjust as needed over time. Here are the steps to take:
- Monitor: Measure AI performance, using well-defined metrics.
- Evaluate: Assess whether AI performance is meeting objectives.
- Optimize: Refine AI models and processes to improve effectiveness and reach your goals.
Constant evaluation and evolution ensures that AI performance stays in step with your business objectives and continues to drive value. Keep in mind that metrics and goals may need to change along the way as technologies and the business develop.
Create a roadmap
Once you’ve explored the AI landscape and decide on the major elements of your AI strategy, it’s time to create a roadmap. The roadmap outlines the people, processes, resources and timelines essential to turning your AI strategy into reality. A clear, actionable roadmap ensures that stakeholders are aligned with the greater strategic vision, AI projects are implemented according to consistent protocols, and AI is comprehensively integrated into the organization. This plan paves the way for successful AI implementation and long-term business success.
How to effectively use AI and data with Databricks
Databricks Mosaic AI enables enterprises to integrate data and AI with streamlined management. Mosaic AI is on the Databricks Data Intelligence Platform, a lakehouse-based unified platform for data and governance that provides a backbone for tailored AI-powered applications.
With Mosaic AI, organizations can build and deploy AI models, from predictive models to advanced generative AI and LLMs. Mosaic AI allows enterprises to have complete control over their data and models while maintaining AI quality and lowering costs. They can also govern and track data and model lineage across the full AI lifecycle.
Mosaic AI offers enterprises the ability to customize AI solutions using their unique data, then monitor and govern data, features and AI models—all in one place. This advanced solution empowers enterprises to fully harness the power of AI to transform the future.