(This post written in collaboration with Zeqiu (Ellen) Wu and Yushi Hu, both PhD students affiliated with the University of Washington, and co-first authors on the paper presented at NeurIPS 2023)
In this blog post, we discuss Fine-Grained RLHF, a framework that enables training and learning from reward functions that are fine-grained in two different ways: density and diversity. Density is achieved by providing a reward after every segment (e.g., a sentence) is generated. Diversity is achieved by incorporating multiple reward models associated with different feedback types (e.g., factual incorrectness, irrelevance, and information incompleteness).
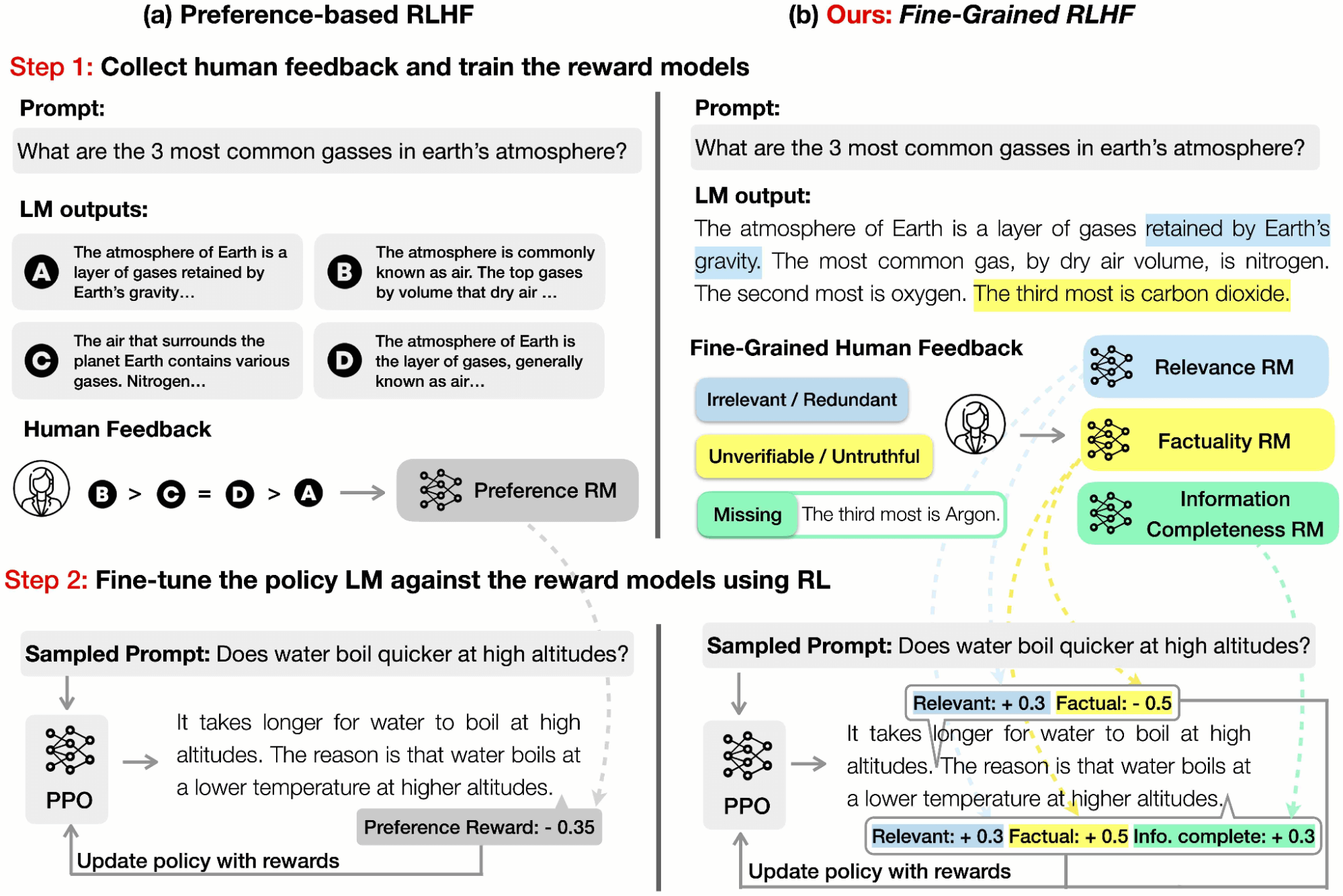
What are Fine-Grained Rewards?
Prior work in RLHF has been focused on collecting human preferences on the overall quality of language model (LM) outputs. However, this type of holistic feedback offers limited information. In a paper we presented at NeurIPS 2023, we introduced the concept of fine-grained human feedback (e.g., which sub-sentence is irrelevant, which sentence is not truthful, which sentence is toxic) as an explicit training signal.
A reward function in RLHF is a model that takes in a piece of text and outputs a score indicating how “good” that piece of text is. As seen in the figure above, traditionally, holistic preference-based RLHF would provide a single reward for the entire piece of text with the definition of “good” having no particular nuance or diversity.
In contrast, our rewards are fine-grained in two aspects:
(a) Density: We provided a reward after each segment (e.g., a sentence) is generated, similar to OpenAI's "step-by-step process reward". We found that this approach is more informative than holistic feedback and, thus, more effective for reinforcement learning (RL).
(b) Diversity: We employed multiple reward models to capture different types of feedback (e.g., factual inaccuracy, irrelevance, and information incompleteness). Multiple reward models are associated with different feedback types; interestingly, we observed that these reward models both complement and compete with each other. By adjusting the weights of the reward models, we could control the balance between the different types of feedback and tailor the LM for different tasks according to specific needs. For instance, some users may prefer short and concise outputs, while others may seek longer and more detailed responses.
Human feedback obtained anecdotally from human annotators was that labeling data in fine-grained form was easier than using holistic preferences. The likely reason for this is that judgments are localized instead of spread out over large generations. This reduces the cognitive load on the human annotator and results in preference data that is cleaner, with higher inter-annotator agreement. In other words, you're likely to get more high quality data per unit cost with fine-grained feedback than holistic preferences.
We conducted two major case studies of tasks to test the effectiveness of our method.
Task 1: Detoxification
The task of detoxification aims to reduce the toxicity in the model generation. We used Perspective API to measure toxicity. It returns a toxicity value between 0 (not toxic) and 1 (toxic).
We compared two kinds of rewards:
(a) Holistic rewards for (non-)toxicity: We use 1-Perspective(y) as the reward.
(b) Sentence-level (fine-grained) rewards for (non-)toxicity: We query the API after the model generates each sentence instead of generating the full sequence. For each generated sentence, we use -Δ(Perspective(y)) as the reward for the sentence (i.e. how much toxicity is changed from generating the current sentence).
Table 1 shows that our Fine-Grained RLHF with sentence-level fine-grained reward attains the lowest toxicity and perplexity among all methods while maintaining a similar level of diversity. Figure 2 shows that learning from denser fine-grained rewards is more sample-efficient than holistic rewards. One explanation is that fine-grained rewards are located where the toxic content is, which is a stronger training signal compared with a scalar reward for the whole text.
Task 2: Long-Form Question Answering
We collected QA-Feedback, a dataset of long-form question answering, with human preferences and fine-grained feedback. QA-Feedback is based on ASQA, a dataset that focuses on answering ambiguous factoid questions.
There are three types of fine-grained human feedback, and we trained a fine-grained reward model for each of them:
1: Irrelevance, repetition, and incoherence (rel.); The reward model has the density level of sub-sentences; i.e., returns a score for each sub-sentence. If the sub-sentence is irrelevant, repetitive, or incoherent, the reward is -1; otherwise, the reward is +1.
2: Incorrect or unverifiable facts (fact.); The reward model has the density level of sentences; i.e., returns a score for each sentence. If the sentence has any factual error, the reward is -1; otherwise, the reward is +1.
3: Incomplete information (comp.); The reward model checks if the response is complete and covers all the information in the reference passages that are related to the question. This reward model gives one reward for the whole response.
Fine-Grained Human Evaluation
We compared our Fine-Grained RLHF against the following baselines:
SFT: The supervised finetuning model (trained on 1K training examples) that is used as the initial policy for our RLHF experiments.
Pref. RLHF: The baseline RLHF model that uses holistic reward.
SFT-Full: We finetuned LM with human-written responses (provided by ASQA) of all training examples and denoted this model as SFT-Full. Notice that each gold response takes 15 min to annotate (according to ASQA), which takes much longer time than our feedback annotation (6 min).
Human evaluation showed that our Fine-Grained RLHF outperformed SFT and Preference RLHF on all error types and that RLHF (both preference-based and fine-grained) was particularly effective in reducing factual errors.
Customizing LM behaviors
By changing the weight of the Relevance reward model and keeping the weight of the other two reward models fixed, we were able to customize how detailed and lengthy the LM responses would be. In Figure X, we compared the outputs of three LMs that were each trained with different reward model combinations.
Fine-Grained reward models both complement and compete with each other
We found that there is a trade-off between the reward models: relevance RM prefers shorter and more concise responses, while Info Completeness RM prefers longer and more informative responses. Thus, these two rewards compete against each other during training and eventually reach a balance. Meanwhile, Factuality RM continuously improves the factual correctness of the response. Finally, removing any one of the reward models will degrade the performance.
We hope our demonstration of the effectiveness of fine-grained rewards will encourage other researchers to move away from basic holistic preferences as the basis for RLHF and spend more time exploring the human feedback component of RLHF. If you would like to cite our publication, see below; you can also find more information here.