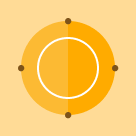
"The bottleneck (for AI) now is in management, implementation, and business imagination, not technology." -Erik Brynjolfsson
Data and AI have emerged as strategic imperatives for most organizations over the last few years. Companies big and small have rallied to hire scores of data and AI experts and have made considerable investments in upgrading and evolving their data platforms.
Thus far, most of the investments have focused on the technology and the technical skills needed to leverage data and AI, and to solve the technological challenges that have emerged with the scale and complexity of an ever evolving data landscape. At Databricks, we are known for facing those challenges head-on and solving them, be it by inventing the lakehouse paradigm, enabling easier sharing and collaboration with Delta Sharing or by providing end-to-end MLOps capabilities with MLFlow.
However, technology alone cannot solve every issue and even the best technology if not used properly is sure to fall short of expectations. This is a trend that we have seen lately in AI. At Databricks we have recognized that having the right operating model, processes and enabling the best ways of working are as essential to the success of companies seeking to make the best use of data and AI as having the right technology, algorithms and skills to develop it.
Part I of this series focuses on a general overview of how the Databricks platform supports a modern operating model for AI, while subsequent parts will delve into the details of what it means for teams and organizations. With that in mind, let's dive into how Databricks supports your operating model for AI:
- Open Collaboration: Being able to establish clear lines of communication within and across teams is fundamental to the success of AI. Currently data engineers, data scientists and analysts work for the most part in separate technology stacks and collaboration between them is minimal or strained at best.
Databricks has endeavored to create a unified environment where these personas can work together to cross-skill, reduce handshakes and converge stages thus greatly reducing technical debt.
At an organizational level, concepts like Data Mesh that have garnered much traction in recent times are almost impossible to implement in the current siloed state that many organizations find themselves in. Open data formats like Delta, and open sharing protocols like Delta Sharing are a game changer for organizations looking to become data forward and empower their teams to develop useful data products. This is because these protocols allow for data to be treated and accessed in a standardized manner and to be securely shared and used both within and outside the organization.
Databricks provides a platform to easily implement the processes needed to bring together Data Providers, Data & AI Professionals and Data Consumers. - Governance & Management: The success of any operating model hinges on accountability, traceability and transparency. Amidst an increasingly complex business and data context, it is essential to put processes in place to safeguard and optimize the use and access to all data artifacts such as tables, files, AI models and dashboards.
The Databricks Lakehouse Platform provides the means to enable a unified governance model through the Unity Catalog. With Unity Catalog, fine grained permissions can be assigned to data artifacts, and users can be grouped and managed in a centralized way. Indeed the ability to easily manage teams and users in terms of what data and what resources they have access to, is instrumental for a sustainable operating model and cost management.
The features Databricks provides to facilitate resource policy, user management, data discovery and access controls makes it possible to define and operate organizational structures that are secure, compliant and that make the best use of the resources available to them, minimizing waste and increasing productivity. Yet another benefit of clear policies and guidelines that Databricks enables is the ability to provide detailed insight and auditability on every action that is taken within the platform and make this available for auditing, quality assurance and regulatory compliance.
Putting together the above means, for instance, following on the Data Mesh example that the role of data owners can be effectively defined and they'll have the tools to manage and trace the creation and usage of their data products so that they may be able to track their impact on the organization.
Databricks enables ownership and governance of data and compute resources and facilitates the management of various teams and organizational structures so that they can work safely and effectively. - Run and Operate: Ultimately the objective of an operating model for AI is to streamline the value generation process from data to business results. Developing AI applications is a highly iterative process and generally strives in an agile environment.
The Databricks platform provides an environment that covers the end-to-end lifecycle of Machine learning operations, from exploration and innovation to experimentation, tracking and deployment. Databricks provides organizations with a MLOps framework and set of tools focused on data centric AI for its teams to follow and to have clear standards for development, testing and serving.Indeed on the topic of tracking and executing a critical part of the success of an operating model for AI is to be able to effectively link the usage of data and AI with measurable business outcomes and Databricks provides the foundation upon which such KPIs can be gathered, governed and maintained. One such KPI that can be used to express the value of data to the business is Return on Data Assets (RODA).
Consider that when running and operating AI projects there are important commercial and budgetary considerations that need to be made. Funding, chargebacks and cost allocation are important for operating a healthy Data and AI pipeline that delivers tangible business results. Databricks gives organizations the ability to track and quantify development cycles from use cases to production and establish standards on how to develop and run workloads that can be enforced, owned, validated and reported upon.
But AI projects do not end when the model is deployed, models need to be monitored, updated and maintained. Here again Databricks provides teams the tools and structure necessary to build clear processes around model management and to effectively link the model outputs to business outcomes closing the virtuous cycle of connecting data with improved business performance.
Databricks streamlines the path to production and enables organizations to make the connection between data and AI development and business results.
Conclusion
Organizations operate in a wide variety of contexts and come in many different shapes and sizes, hence it makes sense that there is no universal operating model for AI that fits everywhere. Regulatory requirements, data and resource availability and many other factors will play a role in determining the right operating model.
It is important then, to have a platform that is able to scale with the organization as more use cases and users enter the pipeline, as more models are deployed and as more of the business is enhanced by data and AI. The services that underpin the operating model need to be flexible while at the same time ensuring that security and explainability permeate every step of the process.
Databricks helps companies overcome not only their technical challenges by providing state-of-the-art data processing and AI capabilities but it provides the structure and platform on top of which organizations can:
- Increase speed to value and realize and track tangible business benefits.
- Enable Governance and collaboration.
- Establish Ownership and operate end-to-end.
Visit Databricks.com to learn more about how Databricks helps its clients solve their toughest challenges with Data and AI!