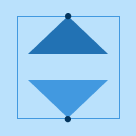
In the era of rapid data growth and increasing pressure on financial institutions to utilize data for AI or genAI models, data governance is becoming increasingly critical. Regulators are paying more attention to AI applications, with the European Union (EU) Parliament passing comprehensive AI regulations and the U.S. federal government taking steps to regulate AI use. This highlights the growing importance of AI regulation. [To learn more, this was summarized in a Databricks blog, "AI regulation is rolling out"]
Data governance is foundational and must precede the use of generative AI. Without it, financial institutions cannot meet regulatory demands, explain AI results, or control for algorithmic and data-centric bias. As AI models become more complex, it's crucial to consider how they are governed and how they interact with both internal and external data assets.
Data Governance is foundational and comes before GenAI
As data and technology leaders introduce more complexity with generative AI, they need to think not just about the data types covered, but also how they cover AI models. They must also consider both data assets within the organization and outside of it. This extends to how they can leverage generative AI to automate manual processes and reduce the time spent on data tagging.
Gartner predicts that by 2026, 20% of large enterprises will use a single data and analytics governance platform to unify and automate discrete governance programs. This simplified approach reduces the need to maintain policies and controls across every data asset in a silo.
Getting started with governance: People, Process, and Platform
To start with governance, three essential elements are needed: people, process, and platform.
People: For people, the focus should be on technical enablement, ensuring it translates to business users. Empower users for transformation. Successful data and AI strategies depend on employees' adoption and use of insights, leading to behavioral changes. Empower, reskill, and encourage data and AI usage across all levels.
Key questions: How to tailor data access and insights for different roles? Are you sharing / providing data (internally or externally) or trying to? How do you foster a culture of continuous learning for new technologies? Think about how you can make it accessible for business users – they didn't grow up in Python, they didn't grow up in SQL, so what are the things you're going to do to bring them along on the generative AI journey that's not just founded or grounded solely in the technology?
Process: The process should start with the end goal, aligning the data and AI strategy with business goals, prioritizing the IT stack, and establishing a structured, enterprise-wide journey. Take an agile approach to people too – often, people violate policy because they didn't understand it. Make sure you integrate an agile approach into your AI policies.
Key questions: How do you transition from ad hoc to structured adoption of AI? How can you quickly identify what's working and why? How can you address non-working aspects and make adjustments? Does the organization lack a data strategy grounded in a data democratization?
Platform: The platform should have the right enforcement in place, balancing the need for risk minimization and innovation. It should adopt open interfaces and data formats to navigate future disruptions. Many CISOs want to minimize risk. And often, this results in stopping or slowing down innovation to ensure controls are in place. On the other hand, you have the Chief Product Officers who are all about innovating as fast as possible. So make sure that you're balancing those processes.
Key questions: Does your firm have (multiple) disparate / incompatible platforms? Where do you need to set up boundaries and barriers and controls? How do you balance proprietary solutions with open source for flexibility and efficiency? How can you prepare for the pace of innovation? Is the organization trying to implement a data mesh architecture?
Unity Catalog supports data governance, unifying data and AI
Financial services technology leaders face pressure to cut costs, manage risks, and ensure compliance, while also monetizing data and fostering innovation. Databricks Unity Catalog is a unified governance solution for data and AI on the Data Intelligence Platform. It simplifies enterprise governance, sharing, and collaboration by offering a unified model for data, analytics, and AI. It also enables secure access and collaboration on trusted data, using AI to boost productivity and fully utilize the lakehouse architecture's capabilities.
Unity Catalog is transforming data governance by providing a unified layer for managing structured and unstructured data, machine learning (ML) models, and other digital assets across any cloud or platform. It enables secure access and collaboration on trusted data, using AI to boost productivity and fully utilize the lakehouse architecture's capabilities.
The benefits of unified governance for data and AI are as follows:
- Unified visibility: Central cataloging of all data, analytics, and AI assets across clouds, regions, and platforms allows teams to discover, access, and analyze information easily. This can accelerate innovation and reduce costs.
- Unified access management: Providing a single tool for access management simplifies policy management and offers enhanced security for data and AI.
- End-to-end monitoring and reporting: The ability to monitor and audit data entitlements and access patterns of sensitive data and AI assets from one place facilitates proactive monitoring and robust access controls, minimizing vulnerabilities and mitigating the risk of non-compliance and security breaches.
- Platform-independent sharing and collaboration: A standardized approach to facilitate cross-platform, cross-cloud, and cross-region secure sharing of data and AI assets, including ML models, dashboards, and notebooks. This reduces duplication costs while also enabling collaboration with a vast ecosystem of data providers, partners, and customers to unlock new revenue streams and drive business value.
Data governance in action in Financial Services
National Australia Bank (NAB): NAB, Australia's largest business bank, relies on Databricks to securely deliver data at speed and scale. Databricks provides access to reliable data in one platform, and Unity Catalog enables governance across the company – ensuring access to data whenever business users need it. NAB is unlocking use cases that were previously out of reach. Now, they can explore generative AI for customer service, marketing campaigns and financial crime detection, reporting and monitoring. Watch the video
Coastal Community Bank: Coastal Community Bank is headquartered in Everett, Washington, far from the world's largest financial centers. The bank's CCBX division offers banking as a service (BaaS) to financial technology companies and broker-dealers. To provide personalized financial products, better risk oversight, reporting and compliance, Coastal turned to the Databricks Data Intelligence Platform, including Unity Catalog.
Applying software engineering principles to data can often be neglected or ignored by engineering teams, but Coastal knew that it was critical to managing the scale and complexity of the internal and external environment in which they were working. This included having segregated environments for development, testing and production, having technical leaders approve the promotion of code between environments, and include data privacy and security governance. Read more
Block: At Block, data transfers and siloed governance policies exacerbated challenges in auditing and policy enforcement with IAM roles. With over 12PB of governed data, Block turned to Databricks Unity Catalog which unifies Block's data estate, simplifying access management and cost attribution. Secure access to sensitive data maintained through fine-grained access policies ensures compliance and ultimately reduces data egress cost by 20%. Read more
Simplifying governance at a time when genAI testing and experimentations is at an all time high is critical. That's why we're excited to announce DBRX, a new standard in efficient and quality-assured LLM development. DBRX offers built-in governance and monitoring, ensuring data integrity from the initial stage to the final model. It empowers users to create custom models securely and cost-effectively using enterprise data. With DBRX, you can ensure the highest production quality for your models, surpassing open source standards on all benchmarks.