Personalizing fantasy sports for millions of users
Faster time-to-market for new ML-powered solutions
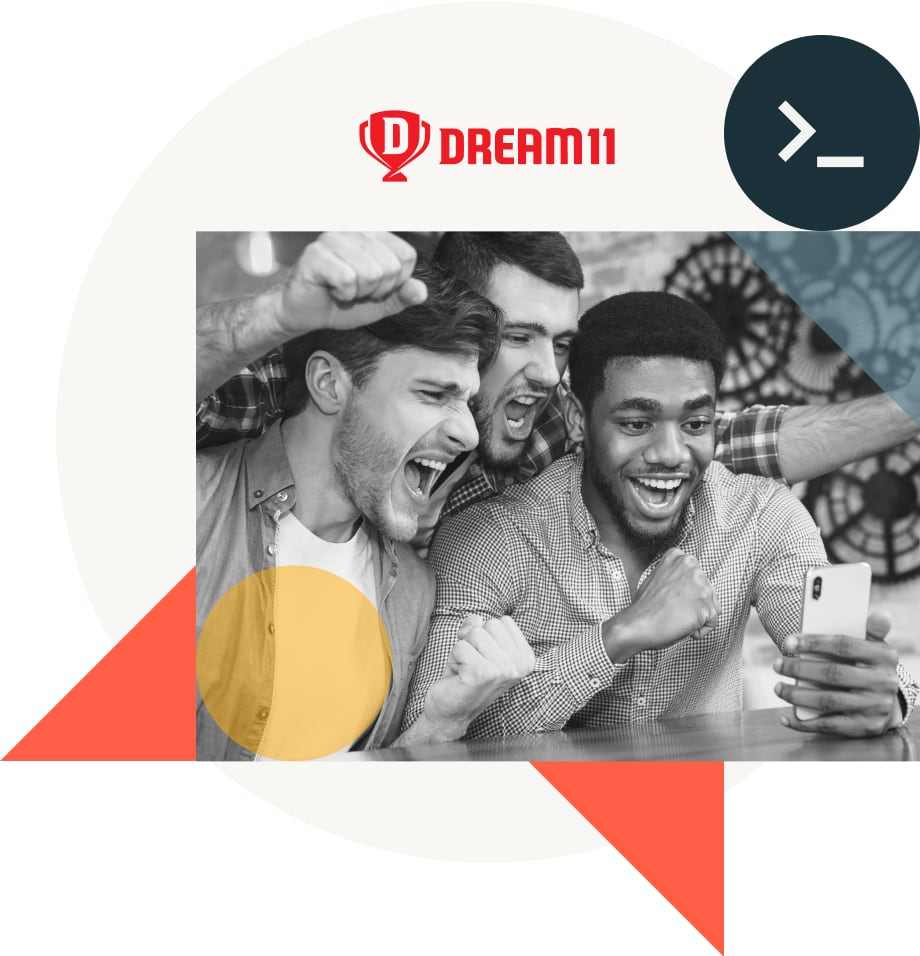
Dream11 is the world’s largest fantasy sports platform, with over 120 million users playing fantasy cricket, football, kabaddi, basketball, hockey, volleyball, handball, rugby, futsal, American football and baseball. The platform manages about 100 million requests per minute, with more than 16 terabytes of information and billions of data points per day. With phenomenal volumes of user activity and engagement on its platform, Dream11 has been on a mission to raise the bar for driving adoption as well as personalizing its fantasy sports experience for users at scale. But without scalable machine learning capabilities, they were unable to tap into the promise of their data to create next-level fantasy sporting experiences. By leveraging Databricks, Dream11 aims to democratize data across teams, foster seamless collaboration to improve productivity and innovation, and deliver on customer-centric solutions that help them stay ahead of the curve in critical areas ranging from fraud detection to data security and personalization.
Data complexity and the complications of scaling across applications
During the 2020 Indian Premier League, Dream11 saw a surge in participation, with over 100 million requests per minute. Managing the experience of users and ensuring the efficient operation of their fantasy sports platform — with up to 5.5 million concurrent users — was no easy feat.
Legacy systems created unmanageable complexities like sharing code and scaling compute infrastructure, which drained DevOps resources and compounded management overhead. The limited machine learning capabilities hindered Dream11’s ability to develop recommendation engines to personalize each user journey, much less enable participants to access customized contests and content efficiently. Furthermore, the plausibility of fraud detection and the safety of its users, particularly should Dream11’s terms of use be breached, was a reality that their data team had difficulties in scaling ML pipelines to over 120 million users.
“In order to build the ML systems necessary for personalized fantasy sports recommendations, real-time fraud detection and operational efficiency, Dream11 needed a centralized, highly reliable and data scientist friendly machine learning platform that could scale a massive and sudden surge of data seamlessly across all our entities at speed,” said Nilesh Patil, Lead Applied Scientist at Dream11.
Bringing an open and collaborative data experience to Dream11
By unleashing the possibilities of working with Databricks on AWS, Dream11 was able to take full advantage of working seamlessly across different enterprise groups while adhering to good data governance processes by varying access and involvement according to the needs of its team members.
In doing so, the Dream11 teams improved their ability to securely and smoothly partner with one another, harness and build on similar designs from other members in the team, and launch their products within a shorter period of time. Where code sharing used to be a messy and complex process, Dream11 would now be able to share analysis, notebooks and production jobs more seamlessly using Databricks. This allowed Dream11 to work in a more collaborative way across teams to get a machine learning system to go live under the vigilant eye of its DevOps team. By revving up its speed to market, Dream11 was also able to deliver a critical machine learning use case involving personalization, social recommendations and real-time predictions for the Indian Premier League within just one week.
Databricks had also enabled the Dream11 team to embrace a greater sense of agility in their work. This has resulted in its propensity to scale at a much faster pace, process voluminous data quickly and launch their products without the conventional bottlenecks of having to set up infrastructure from scratch each time.
“Databricks has enabled us to consolidate massive amounts of data under a unified ML platform while taking personalization and fraud detection to the next level. Our internal teams have also been able to collaborate more efficiently, allowing us to improve the experience of playing fantasy sports and grow our user base,” said Pradip Thoke, Vice President, Data Engineering at Dream11.
Growing users and maintaining customer retention by focusing on personalization
Today, Dream11’s once-siloed data teams are now working across the enterprise and collaborating seamlessly with one another — and with machines — for a marked improvement in data processing and machine learning. Case in point, Dream11’s Databricks adoption doubled in September 2020 within 3 months, increasing productivity and speeding up processes for Dream11. Supported by higher data processing capabilities, Dream11 has shortened its time-to-market of new ML solutions — such as scaled-out recommendation systems and real-time fraud and anomaly detection programs — from 5 months to 4 weeks.
“Our collaboration with Databricks has enabled us to take control of our data and manage it more efficiently. Not only were we able to scale the data, but thanks to the integrated siloed teams, we are better able to launch our product, deliver personalization and enhance our fraud detection systems,” said Abhishek Ravi, CIO at Dream11.
With user concurrency expanding from 3 million in 2020 to 5.5 million in 2021 and with “requests per minute” jumping from 40 million RPM in 2020 to 100 million RPM in 2021, Dream11 is today in pole position to exceed its goal to further scale its user base. How’s that for an impeccable track record of scaling with data?