Bolstering defense intelligence for a safer world
to deploy a fully trained sentiment analysis model
model accuracy, compared with 64% using GPT-4
reduction in model latency
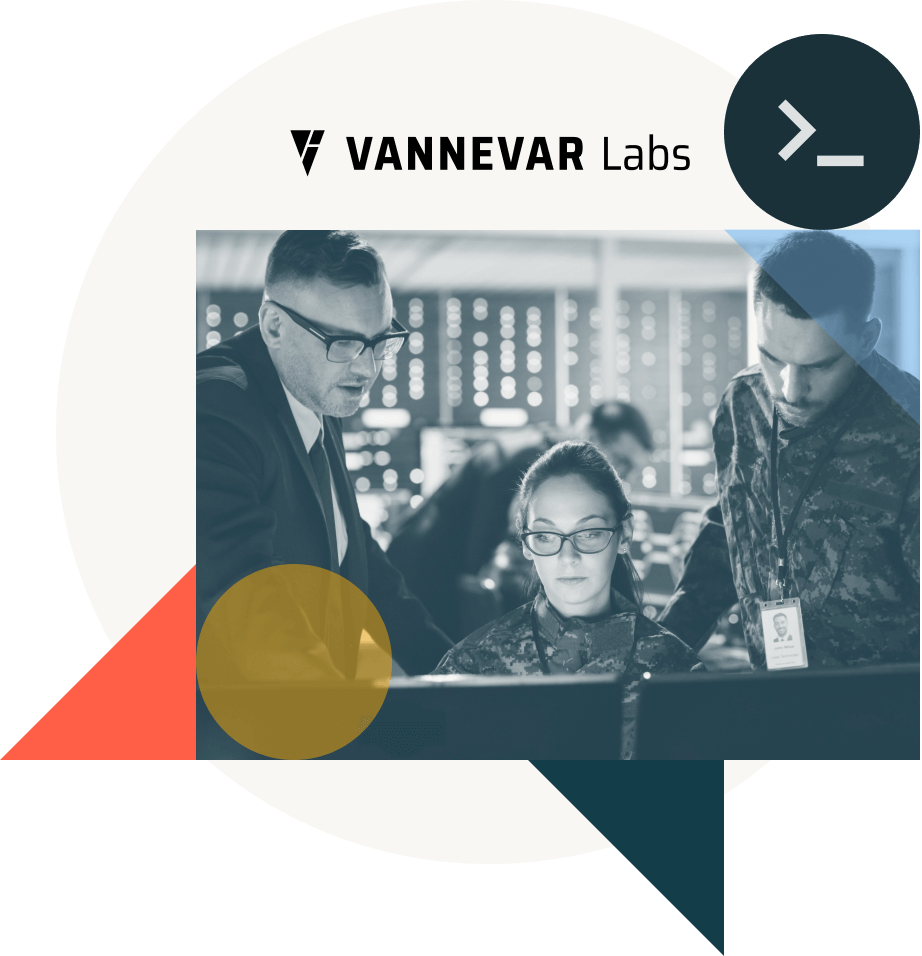
Defense-tech startup Vannevar Labs plays a critical role in supporting America’s strategic efforts to deter and de-escalate global conflicts. Operating at the forefront of defense technology, the company leverages advanced software and hardware to support hundreds of mission-focused users across various branches of the U.S. Department of Defense (DoD). Before partnering with Databricks, Vannevar Labs struggled with the limitations of commercial models, such as GPT-4, which delivered suboptimal accuracy and was not cost-effective, especially given the multilingual complexities of their data. However, after using Databricks Mosaic AI to integrate a compound AI system, the team successfully fine-tuned and deployed a highly accurate sentiment analysis model within just two weeks, reducing latency by 75% and achieving significant cost savings. This transformation has empowered Vannevar Labs to scale their AI-driven insights more efficiently and continue enhancing their mission-critical applications, opening new possibilities for continuous improvement and innovation in defense technology.
Trustworthy sentiment analysis is hindered by inaccurate training models
Vannevar Labs is a defense-tech startup that supports those on America’s front lines tasked with deterring and de-escalating conflict, particularly with Russia and China. The company is dedicated to building advanced software and hardware that inform and support this mission across a variety of contexts, including maintaining maritime vigilance, disrupting misinformation and collecting nontraditional intelligence. As Cane Punma, Senior Machine Learning Engineer at Vannevar Labs, explained, “In strategic competition, the intelligence and actions that shape conflict occur well before the conflict itself. We build tools to win this fight now.”
Vannevar Labs operates at the cutting edge of defense technology, providing solutions that cater to hundreds of mission-focused users across various branches of the DoD. Their business use cases are diverse, ranging from maritime sensing systems to sentiment analysis for characterizing and tracking the impact of misinformation. Specifically, Vannevar sought to improve the accuracy of classifying the sentiment of news articles, blogs and social media related to specific narratives — a critical capability in their efforts to understand the strategic communication of nation-states.
Despite their innovative approach, Vannevar Labs faced significant challenges that led them to seek out Databricks to build this sentiment analysis model. Initially, Punma’s team struggled with the limitations of using GPT-4 and prompt engineering, which did not yield satisfactory results for their specific needs. “The best results we could get were around 65% accuracy, and it was overall too expensive for us,” Punma added. “There was also a multilingual problem. We have data in Tagalog, Spanish, Russian and Mandarin, and GPT-4 struggled with lower-resourced languages like Tagalog.”
This led them to consider fine-tuning a model using their domain-specific data. However, they encountered several hurdles, particularly in spinning up the necessary GPU resources to fine-tune these models, as GPUs were in short supply at the time. Gathering a sufficient number of instruction labels to fine-tune these models was a companywide challenge.
Challenges in orchestration and infrastructure management further complicated his team’s efforts. “Figuring out the most efficient way to train our models so that we can improve how many cycles we do is important, and infrastructure management is a big part of that equation,” Punma noted. “We work with publicly available data, but we still have to find it and aggregate it from multiple places.”
Fine-tuning and training multilingual data with Databricks Mosaic AI
To overcome the hurdles Vannevar Labs faced in their sentiment analysis use case, the company turned to Databricks. Using Databricks Mosaic AI, Vannevar built an end-to-end compound AI system supporting data ingestion, model fine-tuning and deployment, enabling them to achieve the accuracy and efficiency required for their critical defense missions.
Punma’s team leveraged Mosaic AI Model Training to fine-tune their models. Specifically, they fine-tuned Mistral’s 7B parameter model using domain-specific data. This model was chosen for its open source nature and its ability to efficiently operate on a single NVIDIA A10 Tensor Core GPU. “To meet the real-time demands of our applications, we needed a smaller model to fit on a single A10 and have very low real-time latency,” Punma explained.
Mosaic AI’s Command Line Interface (MCLI) and Python SDK tools made it easy for Vannevar to orchestrate, scale and monitor the GPU nodes and container images used in model training and deployment. MCLI’s robust capabilities for data ingestion allowed seamless, secure connection to Vannevar’s datasets, and played a crucial role in the model training lifecycle.
Additional platform features enabled Vannevar Labs to convert their trained models to a standard Hugging Face format and export them to their Amazon S3 or Hugging Face Model Repository for production use. According to Punma, “We had a kickoff call with the Mosaic engineering team, and they pointed me to this Hugging Face repo that was really helpful. It has a lot of good examples of the full workflow of fine-tuning any large language model (LLM) from scratch. The repo primarily uses the MPT-7B model and outlines the key steps for MDS conversion, domain adaptation, instruction fine-tuning, and then converting the model for deployment. It’s a really comprehensive resource, and I was able to adapt it perfectly for our use case.”
Databricks also facilitated efficient training across multiple GPUs by managing the configurations through YAML files, which significantly simplified the orchestration and infrastructure management. “I’ve had experience fine-tuning models, but I think Databricks Mosaic AI offers an incredibly efficient infrastructure for fine-tuning a whole LLM network,” Punma added. “The MCLI managed configurations allowed us to easily adapt training parameters and efficiently train across multiple GPUs, hooking into third-party monitoring tools like Weights & Biases.”
Deploying an improved sentiment analysis model in 2 weeks
Databricks Mosaic AI not only provided the technical resources necessary to fine-tune and deploy models but also streamlined the entire machine learning process, from data ingestion to model deployment — making it possible for Vannevar Labs to enhance their sentiment analysis capabilities effectively. Punma said, “Within just 2 weeks, we were able to go from a tutorial to deploying a fully functional, fine-tuned sentiment analysis model.” This rapid deployment was a critical success factor, enabling the company to enhance their collection efforts across multiple defense missions quickly.
The fine-tuned model achieved an overall F1 score of 76%, an improvement over the 65% accuracy previously achieved with GPT-4, and delivered results faster and more cost-effectively. Latency time was reduced by 75% compared with previous implementations. According to Punma, “On all three fronts — accuracy, cost and speed — the fine-tuning solution built and trained with Databricks came out ahead. The latency is way faster — a quarter of the time — so we’re able to run large backfill jobs and process significantly more data more efficiently.”
To learn more about how Vannevar Labs uses advanced technology to help the U.S. and its allies deter and de-escalate conflict around the world, visit vannevarlabs.com.