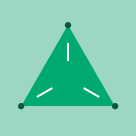
Databricks Is Named a Visionary in the 2020 Gartner Magic Quadrant for Cloud Database Management Systems (DBMS)
Last week, Gartner published the Magic Quadrant (MQ) for Cloud Database Management Systems, where Databricks was recognized as a Visionary in the market.1 This was the first time Databricks was included in a database-related Gartner Magic Quadrant. We believe this is due in large part to our investment in Delta Lake and its ability to enable data warehousing workloads on data lakes. Combined with our position as a Leader in the 2020 Magic Quadrant for Data Science and Machine Learning Platforms2 announced earlier this year, Databricks is one of only a few vendors to be included in both MQ reports and the only one to achieve it through a unified platform due to our focus on lakehouse architecture.
Gartner evaluated 16 vendors for their completeness of vision and ability to execute.
We are confident the following attributes contributed to our company’s success:
- Our simple platform combines the best attributes of data lakes and data warehouses to enable lakehouse architecture
- Our unique ability to unify all of your data types and data workloads across all industries
- Our dedication to innovation, data portability, and customer success that’s rooted in technology
One Simple Platform for All Your Data
Databricks’ continued growth has been rooted in the pursuit of lakehouse architecture, which is enabled by a new system design that implements similar data structures and data management features to those in a data warehouse directly on the flexible, low-cost storage used for cloud data lakes. The architecture is what you would get if you had to redesign data warehouses in the modern world, now that cheap and highly reliable storage (in the form of object stores) is available.
By marrying the advantages of both legacy architectures, and leaving behind many of the drawbacks, customers can run both traditional analytics and data science / ML workloads on the same platform. This approach substantially reduces the complex data operations necessary to constantly move data between the data lake and downstream data warehouses. It also has the added benefit of eliminating the inherent data silos that get created so that data teams can operate off of one source of truth. All in all, organizations can increase their velocity and lower their costs by moving towards lakehouse architecture.
Unification of all data types and data workloads
Because of the architectural benefits of a lakehouse, structured, semi-structured, and unstructured data can now coexist as first-class citizens. This is important because the individual roles within data teams are becoming increasingly intertwined.
The biggest advantage of Databricks’ Unified Data Analytics Platform is its ability to run data processing and machine learning workloads at scale and all in one place. Most recently, we significantly extended our data management and analytics capabilities with the announcement of SQL Analytics at the Data+AI Summit Europe 2020. SQL Analytics provides Databricks customers with a first-class experience for performing BI and SQL workloads directly on the data lake. The service provides a dedicated SQL-native workspace, built-in connectors to let analysts query data lakes with the BI tools they already use, innovations in query performance that deliver fast results on larger and fresher data sets than analysts traditionally have access to, and new governance and administration capabilities. Altogether, we can deliver up to 9x better price/performance for analytics workloads than traditional cloud data warehouses.
Additionally with Databricks, data teams can build reliable data pipelines with Delta Lake, which adds reliability and performance to existing data lakes. Data scientists can explore data and build models in one place with collaborative notebooks, track and manage experiments and models across the lifecycle with MLflow, and benefit from built-in and optimized ML environments (including the most common ML frameworks).
Rooted in Open Source
Databricks is the founder of many successful projects, starting with the creation of Apache Spark, a unified analytics engine for large-scale data processing.
Since then, we’ve innovated with Delta Lake as the foundation for the vision of the lakehouse. Delta Lake has brought reliability, performance, governance, and quality to data lakes, which is necessary to enable analytics on the data lake. Thousands of organizations have since adopted Delta Lake to provide an open standard for how they store their data, eliminating the long-term challenges that come with proprietary data formats.
We also created MLflow, an open source machine learning platform to let teams reliably build and productionize ML applications. Since then, we have been humbled and excited by the adoption of the data science community. With more than 2.5 million monthly downloads, 200 contributors from 100 organizations, and 4x year-on-year growth, MLflow has become the most widely used open source ML platform, demonstrating the benefits of an open platform to manage ML development that works across diverse ML libraries, languages, and cloud and on-premise environments. Today, it forms the foundation of our machine learning workflow capabilities to help ensure that customers have access to the most open and flexible set of tools possible.
Overall, with Databricks, customers can make better, faster use of data to drive innovation with one simple, open platform for analytics, data science, and ML that brings together teams, processes and technologies.
Gartner does not endorse any vendor, product or service depicted in its research publications, and does not advise technology users to select only those vendors with the highest ratings or other designation. Gartner research publications consist of the opinions of Gartner’s research organization and should not be construed as statements of fact. Gartner disclaims all warranties, expressed or implied, with respect to this research, including any warranties of merchantability or fitness for a particular purpose.
1Gartner, “Magic Quadrant for Cloud Database Management Systems,” written by Donald Feinberg, Merv Adrian, Rick Greenwald, Adam Ronthal, Henry Cook, November 23, 2020.
2Gartner “Magic Quadrant for Data Science and Machine Learning,” written by Peter Krensky, Pieter den Hamer, Erick Brethenoux, Jim Hare, Carlie Idoine, Alexander Linden, Svetlana Sicular, Farhan Choudhary, February 11, 2020.