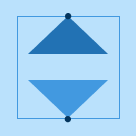
Introduction
On January 4th, a new era in digital marketing began as Google initiated the gradual removal of third-party cookies, marking a seismic shift in the digital landscape. Initially, this development only affects 1% of Chrome users, but it’s a clear signal of things to come. The demise of third-party cookies heralds a new era in digital marketing. As the digital ecosystem continues to evolve, marketers must rethink their approach to engagement and growth, a moment to reassess their strategies and embrace new methodologies that prioritize user privacy while still delivering personalized and effective marketing.
During these moments, the question "What are we looking for?" within marketing analytics resonates more than ever. Cookies were just a means to an end after all. They allowed us to measure what we believed was the marketing effect. Like many marketers, we’ll just aim to demystify the age-old question: “Which part of my advertising budget is really making a difference?”
Demystifying cookies
If we are trying to understand marketing performance, it’s fair to question what cookies were actually delivering anyway. While cookies aimed to track attribution and impact, their story resembles a puzzle of visible and hidden influences. Consider a billboard that appears to drive 100 conversions. Attribution simply counts these apparent successes. However, incrementality probes deeper, asking, "How many of these conversions would have occurred even without the billboard?" It seeks to unearth the genuine, added value of each marketing channel.
Picture your marketing campaign as hosting an elaborate gala. You send out lavish invitations (your marketing efforts) to potential guests (leads). Attribution is akin to the doorman, tallying attendees as they enter. Yet, incrementality is the discerning host, distinguishing between guests who were enticed by the allure of your invitation and those who would have attended anyway, perhaps due to proximity or habitual attendance. This nuanced understanding is crucial; it's not just about counting heads, but recognizing the motives behind their presence.
So you may now be asking, “Ok, so how do actually evaluate incrementality?” The answer is simple: we’ll use statistics! Statistics provides the framework for collecting, analyzing, and interpreting data in a way that controls external variables, ensuring that any observed effects can be attributed to the marketing action in question rather than to chance or external influences. For this reason, in recent years Google and Facebook have moved their chips to bring experimentation to the table. For example, their liftoff or uplift testing tools are A/B test experiments managed by them.
The rebirth of reliable statistics
Within this same environment, regression models have had a renaissance wherein different ways they have been adjusted to consider the particular effects of marketing. However, in many cases challenges arise because there are very real nonlinear effects to contend with when applying these models in practice, such as carry-over and saturation effects.
Fortunately, in the dynamic world of marketing analytics, significant advancements are continuously being made. Major companies have taken the lead in developing advanced proprietary models. In parallel with these developments, open-source communities have been equally active, exemplifying a more flexible and inclusive approach to technology creation. A testament to this trend is the expansion of the PyMC ecosystem. Recognizing the diverse needs in data analysis and marketing, PyMC Labs has introduced PyMC-Marketing, thereby enriching its portfolio of solutions and reinforcing the importance and impact of open-source contributions in the technological landscape.
PyMC-Marketing uses a regression model to interpret the contribution of media channels on key business KPI’s. The model captures the human response to advertising through transformation functions that account for lingering effects from past advertisements (adstock or carry-over effects) and decreasing returns at high spending levels (saturation effects). By doing so, PyMC-Marketing gives us a more accurate and comprehensive understanding of the influence of different media channels.
What is media mix modeling (MMM)?
Media mix modeling, MMM for short, is like a compass for businesses, helping them understand the influence of their marketing investments across multiple channels. It sorts through a wealth of data from these media channels, pinpointing the role each one plays in achieving their specific goals, such as sales or conversions. This knowledge empowers businesses to streamline their marketing strategies and, in turn, optimize their ROI through efficient resource allocation.
Within the world of statistics, MMM has two major variants, frequentist methods, and Bayesian methods. On one hand, the frequentist approach to MMM relies on classical statistical methods, primarily multiple linear regression. It attempts to establish relationships between marketing activities and sales by observing frequencies of outcomes in data. On the other hand, the Bayesian approach incorporates prior knowledge or beliefs, along with the observed data, to estimate the model parameters. It uses probability distributions rather than point estimates to capture the uncertainty.
What are the advantages of each?
Probabilistic regression (i.e., Bayesian regression):
- Transparency: Bayesian models require a clear construction in their structure, how the variables relate to each other, the shape they should have and the values they can adopt are usually defined in the model creation process. This allows assumptions to be clear and your data generation process to be explicit, avoiding hidden assumptions.
- Prior knowledge: Probabilistic regressions allow for the integration of prior knowledge or beliefs, which can be particularly useful when there's existing domain expertise or historical data. Bayesian methods are better suited for analyzing small data sets as the priors can help stabilize estimates where data is limited.
- Interpretation: Offers a complete probabilistic interpretation of the model parameters through posterior distributions, providing a nuanced understanding of uncertainty. Bayesian credible intervals provide a direct probability statement about the parameters, offering a clearer quantification of uncertainty. Additionally, given the fact the model follows your hypothesis around the data generation process, it is easier to connect with your causal analyses.
- Robustness to overfitting: Generally more robust to overfitting, especially in the context of small datasets, due to the regularization effect of the priors.
Regular regression (i.e., frequentist regression)
- Simplicity: Regular regression models are generally simpler to deploy and implement, making them accessible to a broader range of users.
- Efficiency: These models are computationally efficient, especially for large datasets, and can be easily applied using standard statistical software.
- Interpretability: The results from regular regression are straightforward to interpret, with coefficients indicating the average effect of predictors on the response variable.
The field of marketing is characterized by a great amount of uncertainty that must be carefully considered. Since we can never have all the real variables that affect our data generation process, we should be cautious when interpreting the results of a model with a limited view of reality. It's important to acknowledge that different scenarios can exist, but some are more likely than others. This is what the posterior distribution ultimately represents. Additionally, if we don't have a clear understanding of the assumptions made by our model, we may end up with incorrect views of reality. Therefore, it's crucial to have transparency in this regard.
Boosting PyMC-Marketing with Databricks
Having an approach to modeling and a framework to help build models is great. While users can get started with PyMC-Marketing on their laptops, in technology companies like Bolt or Shell, these models need to be made available quickly and accessible to technical and non-technical stakeholders across the organization, and brings several additional challenges. For instance, how do you acquire and process all the source data you need to feed the models? How do you keep track of which models you ran, the parameters and code versions you used, and the results produced for each version? How do you scale to handle larger data sizes and sophisticated slicing approaches? How do you keep all of this in sync? How do you govern access and keep it secure, yet also shareable and discoverable by team members that need it? Let’s explore a few of these common pain points we hear from customers and how Databricks helps.
First, let’s talk about data. Where does all this data come from to power these media mix models? Most companies ingest vast amounts of data from a variety of upstream sources such as campaign data, CRM data, sales data and countless other sources. They also need to process all that data to cleanse it and prepare it for modeling. The Databricks Lakehouse is an ideal platform for managing all those upstream sources and ETL, allowing you to efficiently automate all the hard work of keeping the data as fresh as possible in a reliable and scalable way. With a variety of partner ingestion tools and a huge selection of connectors, Databricks can ingest from virtually any source and handle all the associated ETL and data warehousing patterns in a cost effective manner. It enables you to both produce the data for the models, and process and make use of the data output by the models in dashboards and for analysts queries. Databricks enables all of these pipelines to be implemented in a streaming fashion with robust quality assurance and monitoring features throughout with Delta Live Tables, and can identify trends and shifts in data distributions via Lakehouse Monitoring.
Next, let’s talk about model tracking and lifecycle management. Another key feature of the Databricks platform for anyone working in data science and machine learning is MLflow. Every Databricks environment comes with managed MLflow built-in, which makes it easy for marketing data teams to log their experiments and keep track of which parameters produced which metrics, right alongside any other artifacts such as the entire output of the PyMC-Marketing Bayesian inference run (e.g., the traces of the posterior distribution, the posterior predictive checks, the various plots that help users to understand them). It also keeps track of the versions of the code used to produce each experiment run, integrating with your version control solution via Databricks Repos.
To scale with your data size and modeling approaches, Databricks also offers a variety of different compute options, so you can scale the size of the cluster to the size of the workload at hand, from a single node personal compute environment for initial exploration, to clusters of hundreds or thousands of nodes to scale out processing individual models for each of the various slices of your data, such as each different market. Large technology companies like Bolt need to run MMM models for different markets. However, the structure of each model is the same. Using Python UDF’s you can scale out models sharing the same structure over each slice of your data, logging all of the results back to MLflow for further analysis. You can also choose GPU powered instances to enable the use of GPU-powered samplers.
To keep all these pipelines in sync, once you have your code ready to deploy along with all the configuration parameters, you can orchestrate it’s execution using Databricks Workflows. Databricks Workflows enables you to have your entire data pipeline and model fitting jobs along with downstream reporting tasks all work together according to your desired frequency to keep your data as fresh as needed. It makes it easy to define multi-task jobs and monitor execution of those jobs over time.
Finally, to keep both your model and data secure and governed, but still accessible to the team members that need it, Databricks offers Unity Catalog. Once the model is ready to be consumed by downstream processes it can be logged to the model registry built in to Unity Catalog. Unity Catalog gives you unified governance and security across all of your data and AI assets, allowing you to securely share the right data with the right teams so you’re media mix models can be put into use safely. It also allows you to track lineage from ingest all the way through to the final output tables, including the media mix models produced.
Conclusion
The end of third-party cookies isn't just a technical shift; it's an opportuntiy for a strategic inflection point. It's a moment for marketers to reflect, embrace change, and prepare for a new era of digital marketing — one that balances the art of engagement with the science of data, all while upholding the paramount value of consumer privacy. PyMC-Marketing, supported by PyMC Labs, provides a modern framework to apply advanced mathematical models to measure and optimize data-driven marketing decisions. Databricks helps you build and deploy the associated data and modeling pipelines and apply them at scale across organizations of any size. To learn more about how to apply MMM models with PyMC-Marketing on Databricks, please check out our solution accelerator, and find out how easy it is to take the next step marketing analytics journey.
Check out the updated solution accelerator, now using PyMC-Marketing today!