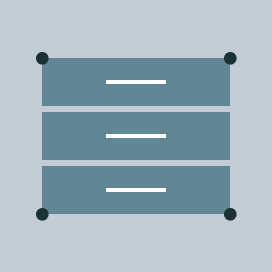
The observation that "software is eating the world" has shaped the modern tech industry. Today, software is ubiquitous in our lives, from the watches we wear, to our houses, cars, factories and farms. At Databricks, we believe that soon, AI will eat all software. That is, the software built over the past decades will be intelligent, leveraging data, making it much smarter. The implications are vast and varied, impacting everything from customer support to healthcare and education.
In this blog, we give our view on how AI will change data platforms. We argue that the impact of AI on data platforms will not be incremental, but fundamental: massively democratizing access to data, automating manual administration, and enabling turnkey creation of custom AI applications. All this will be enabled by a new wave of unified platforms that deeply understand an organization's data. We call this new generation of systems Data Intelligence Platforms.
Data Platforms So Far and Their Challenges
Data warehouses emerged in the 1980s as a solution for organizing structured business data in enterprises. However, by 2010, organizations began accumulating a significant amount of unstructured data to support more varied use cases, such as AI. To address this, data lakes were introduced as an open, scalable system for any type of data. By 2015, it became common for most organizations to operate both data warehouses and data lakes. This dual-platform approach, however, presented significant challenges in governance, security, reliability and management.
Five years ago, Databricks pioneered the concept of the lakehouse to combine and unify the best of both worlds. Lakehouses store and govern all your data in open formats, and natively support workloads ranging from BI to AI. For the first time, lakehouses offered a unified system to (1) query all data sources in an organization together and (2) govern all the workloads that use data (BI, AI, etc.) in a unified way. Lakehouse became its own category of data platform and is now widely adopted by enterprises and incorporated into most vendors' stacks.
Despite the progress, all current data platforms in the market still face several major challenges:
- Technical Skill Barrier: Querying data requires specialized skills in SQL, Python or BI, creating a steep learning curve
- Data Accuracy and Curation: In large organizations, finding the right and accurate data is a challenge, requiring extensive curation and planning
- Management Complexity: Data platforms can skyrocket in costs and experience poor performance if not managed by highly technical personnel
- Governance and Privacy: Governance requirements across the world are rapidly evolving, and with the advent of AI, concerns around lineage, security and privacy are amplified
- Emerging AI Applications: In order to enable generative AI applications that answer domain-specific requests, organizations have to develop and tune LLMs in platforms that are separate from their data, and connect them to their data through manual engineering
Many of these issues arise because data platforms do not fundamentally understand the data in organizations and how it is used. Fortunately, generative AI presents a powerful new tool to address exactly these challenges.
The Core Idea Behind Data Intelligence Platforms
Data Intelligence Platforms revolutionize data management by employing AI models to deeply understand the semantics of enterprise data; we call this data intelligence. They build on the foundation of the lakehouse – a unified system to query and manage all data across the enterprise – but automatically analyze both the data (contents and metadata) and how it is used (queries, reports, lineage, etc.) to add new capabilities. Through this deep understanding of data, Data Intelligence Platforms enable:
- Natural Language Access: Leveraging AI models, DI Platforms enable working with data in natural language, tailored to each organization's jargon and acronyms. The platform observes how data is used in existing workloads to learn the organization's terms and offers a tailored natural language interface to all users – from nonexperts to data engineers.
- Semantic Cataloguing and Discovery: Generative AI can understand each organization's data model, metrics and KPIs to offer unparalleled discovery features or automatically identify discrepancies in how data is being used.
- Automated Management and Optimization: AI models can optimize data layout, partitioning and indexing based on data usage, reducing the need for manual tuning and knob configuration.
- Enhanced Governance and Privacy: DI Platforms can automatically detect, classify and prevent misuse of sensitive data, while simplifying management using natural language.
- First-Class Support for AI Workloads: DI Platforms can enhance any enterprise AI application by allowing it to connect to the relevant business data and leverage the semantics learned by the DI Platform (metrics, KPIs, etc.) to deliver accurate results. AI application developers no longer have to "hack" intelligence together through brittle prompt engineering.
Some might wonder how this is different from the natural language Q&A capabilities BI tools added over the last few years. BI tools only represent one narrow (although important) slice of the overall data workloads, and as a result do not have visibility into the vast majority of the workloads happening, or the data's lineage and uses before it reaches the BI layer. Without visibility into these workloads, they cannot develop the deep semantic understanding necessary. As a result, these natural language Q&A capabilities have yet to see widespread adoption. With data intelligence platforms, BI tools will be able to leverage the underlying AI models for much richer functionality. We, therefore, believe this core functionality will reside in data platforms.
Databricks as a Data Intelligence Platform
At Databricks, we've been building a data intelligence platform on top of the data lakehouse and have grown increasingly excited about the possibilities of AI in data platforms as we have added individual features. We build on the existing unique capabilities of the Databricks lakehouse as the only data platform in the industry with (1) a unified governance layer across data and AI and (2) a single unified query engine that spans ETL, SQL, machine learning and BI. In addition, we've leveraged our acquisition of MosaicML to generate AI models in a Data Intelligence Engine we call DatabricksIQ, which fuels all parts of our platform.
DatabricksIQ already permeates many of the layers of our current stack. It is used to:
- Set the knobs throughout the platform, including automatically indexing columns, laying out partitions and making the foundation of the lakehouse stronger. This will provide lower TCO and better performance for our customers.
- Improve governance in Unity Catalog (UC) by automatically inserting descriptions and tags of all data assets in UC. These are then leveraged to make the whole platform aware of jargon, acronyms, metrics and semantics. This enables better semantic search, better AI assistant quality and improved ability to do governance.
- Improve the generation of Python and SQL in our AI assistant, powering both text-to-SQL and text-to-Python.
- Make those queries much faster by incorporating predictions about the data into query planning in our Photon query engine.
- Inside Delta Live Tables and Serverless Jobs to provide optimal autoscaling and minimize cost based on predictions about the workload.
Last, but perhaps more importantly, we believe that data intelligence platforms will greatly simplify the development of enterprise AI applications. We are integrating DatabricksIQ directly with our AI platform, Mosaic AI, to make it easy for enterprises to create AI applications that understand their data. Mosaic AI now offers multiple capabilities to directly integrate enterprise data into AI systems, including:
- End-to-end RAG (Retrieval Augmented Generation) to build high quality conversational agents on your custom data, leveraging the Databricks Vector Database for "memory."
- Training custom models either from scratch on an organization's data, or by continued pretraining of existing models such as MPT and Llama 2, to further enhance AI applications with deep understanding of a target domain.
- Efficient and secure serverless inference on your enterprise data, and connected into Unity Catalog's governance and quality monitoring functionality.
- End-to-end MLOps based on the popular MLflow open source project, with all produced data automatically actionable, tracked and monitorable in the lakehouse.
Summary
We believe that AI will transform all software, and data platforms are one of the areas most ripe to innovation through AI. Historically, data platforms have been hard for end-users to access and for data teams to manage and govern. Data intelligence platforms are set to transform this landscape by directly tackling both these challenges – making data much easier to query, manage and govern. In addition, their deep understanding of data and its use will be a foundation for enterprise AI applications that operate on that data. As AI reshapes the software world, we believe that the leaders in every industry will be those who leverage data and AI deeply to power their organizations. DI Platforms will be a cornerstone for these organizations, enabling them to create the next generation of data and AI applications with quality, speed and agility.