Maintaining regulatory compliance with ease
Hawaiian Electric leverages GenAI tools to answer regulatory questions faster
Time spent individually querying regulatory documents — down from five minutes
Implementation time to go from zero to RAG
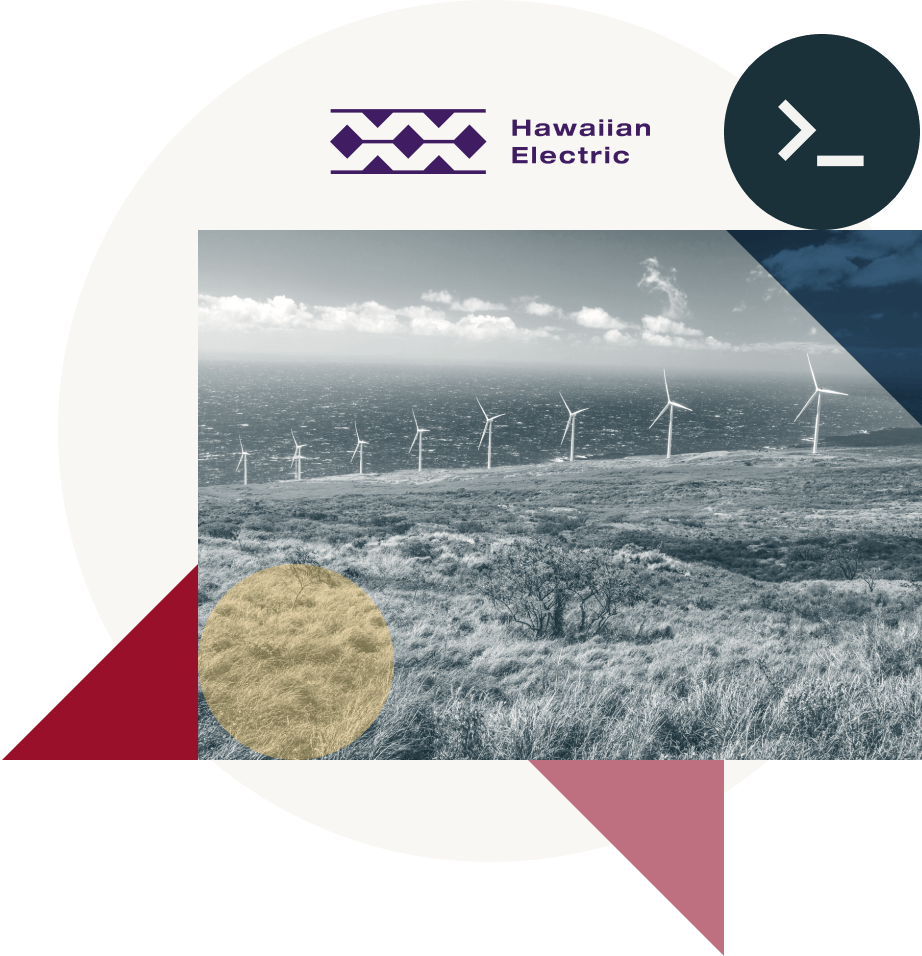
Hawaiian Electric Company (HECO) seeks to create a green Hawaii, providing communities with reliable and renewable energy. The utility company faced operational inefficiencies due to the time-consuming manual referencing of extensive regulatory documentation. HECO leveraged the Databricks Data Intelligence Platform to develop a retrieval augmented generation (RAG) model proof of concept (POC), streamlining question-answering by integrating relevant data into the generation process. This allowed the regulatory team to quickly and accurately cross-reference documentation and provide answers to information requests about regulatory requirements and how HECO is staying compliant. The conversational RAG chatbot significantly reduced the time to answer queries from minutes to seconds, improving both efficiency and accuracy.
Operational bottlenecks for the regulatory team
Hawaiian Electric (HECO), responsible for delivering power to 95% of Hawaii’s residents, grappled with a significant challenge: managing and efficiently accessing a vast trove of regulatory documentation. These documents are critical for referencing and operational decision-making. Unfortunately, the traditional method of manually sifting through these documents was repetitive and time-consuming. This process required HECO’s regulatory team to not only search for the relevant documentation but also to read through the entire text to get answers, hindering their ability to respond to information requests quickly and at scale.
Joel Wasson, Manager of Enterprise Data and Analytics at HECO, described the scenario in more detail. “As a utility, we have massive amounts of regulatory legal documents and we have to cross-reference a significant amount. There’s a deep wealth of information in there as well as legal opinions. We get significant operational demands in the form of information requests — we have to keep pulling up and referencing what we’re doing as a company, what the regulators are telling us, and, more broadly, what the utility industry is doing.”
The introduction of retrieval augmented generation (RAG) presented a compelling solution to this challenge. Unlike off-the-shelf large language models (LLMs) that rely solely on preexisting knowledge and might not be up-to-date or specific to HECO’s unique data, RAG enhances the LLM’s specificity and relevance by integrating real-time data retrieval into the generation process. This means that RAG can pull specific, relevant information from HECO’s regulatory documents in real time, ensuring accurate and contextually appropriate answers.
“Trying to sort and sift and make sense of all this massive amount of content is a challenge. It takes an entire department to do this — which we have — and that’s unique to the utilities industry,” Wasson explained, highlighting the necessity for such a solution. “We thought, ‘Hey, this is a good application for leveraging a generative AI model that could potentially make sense of all this content.’ We wanted to provide it in a more usable format to make it more efficient to reference, cross-reference and answer questions and briefs.”
Building a conversational RAG chatbot with Databricks GenAI
To address these challenges, HECO partnered with Databricks Professional Services to develop a RAG model proof of concept (POC) that significantly streamlined the process of finding relevant information. The Databricks team helped set up a robust endpoint for querying regulatory documents via a RAG model-serving endpoint. The POC also involved creating a user-friendly Databricks Notebook interface that allowed the regulatory team to iteratively refine their queries and a comprehensive LLMOps process to ensure a production-quality system.
To build and deploy their generative AI application, HECO leveraged Databricks Vector Search, a highly performant serverless database with governance built in. In just two weeks, Databricks took HECO from square one to a RAG POC.
“The actual implementation went pretty quickly, largely due to the excellence of Databricks Professional Services, which fast-tracked us quite a bit,” said Wasson. “We had an expert working on this, so there wasn’t a lot of downtime on the learning curve for us. Now that we’re ready to scale out into production, I think it will be a short time frame because of the excellent solution architecture and account management that we received from Databricks. We know what we’re working with, so we’re able to rapidly scale and develop our solution.”
Wasson highlighted the critical role Unity Catalog played in monitoring. “We felt more confident working within the Databricks Data Intelligence Platform knowing that we have security features and control, especially when working with documents that weren’t public. Among the first questions we get asked by lawyers is if the data is secure and where is it going. The ability to say that we’re developing GenAI in our secure environment and without data leaving our control is incredibly significant.”
Another useful platform capability was Vector Search, which automatically generated Delta Live Tables (DLT) pipelines to speed the ingestion of preprocessed data into the vector database for more efficient data processing and transformation. This ensured that data was consistently prepared and available for analysis and supported the RAG model’s Q&A capabilities. The POC ultimately reduced time to insight, allowing the regulatory team to quickly — and accurately — answer questions without manually reading through entire texts. The solution was implemented as a conversational RAG chatbot, enabling users to ask follow-up questions and interact with the model in an intuitive and accessible manner.
Reducing time to answer from minutes to seconds
By automating the process of querying regulatory documents, the utility company significantly reduced the time spent on manual searches. Wasson elaborated, “When asked how long a single answer would take to cross-reference with a piece of documentation manually, one analyst said it was about five minutes. That’s not a lot of time in a singular instance, but it is when multiplied by many times a day. The time savings are pretty significant now that querying takes five seconds as opposed to five minutes.”
The RAG model not only improved efficiency but also enhanced the accuracy and reliability of the information retrieved. This is particularly valuable for the regulatory team, as it ensures that they can provide well-referenced and trustworthy information. With the ability to point out which page of which document is being referenced, the conversational RAG chatbot acts as a source of truth for lawyers.
Looking forward, HECO plans to scale GenAI capabilities to other departments within the company. Because every single manager has to answer questions, Wasson believes that this chatbot should open up beyond his regulatory colleagues. “Whether it’s a customer service support model or using it to query contracts or design documents, there are so many opportunities. I’m excited to open this RAG model up to the rest of the company because it will save a lot of people a lot of time and get people interacting with the data in a better way.” Fortunately, Databricks Model Serving makes model choice a modular component, paving the way for the HECO team to experiment and choose the best external model for future deployments.
Wasson concluded, “To support the mission of our data being used as a strategic asset, we need to democratize the use of our data so that people are making decisions based on the information that’s actually available to them. It’s exciting to tell people that they don’t need to be a Python coder or SQL coder or even look at a dashboard. They can start interacting with the data in a way that’s meaningful to them. And breaking down these barriers hasn’t always been possible — until you have a solution like the Databricks Platform leveraging the Azure infrastructure.”