Introduction to Data Lakes
Data lakes provide a complete and authoritative data store that can power data analytics, business intelligence and machine learning
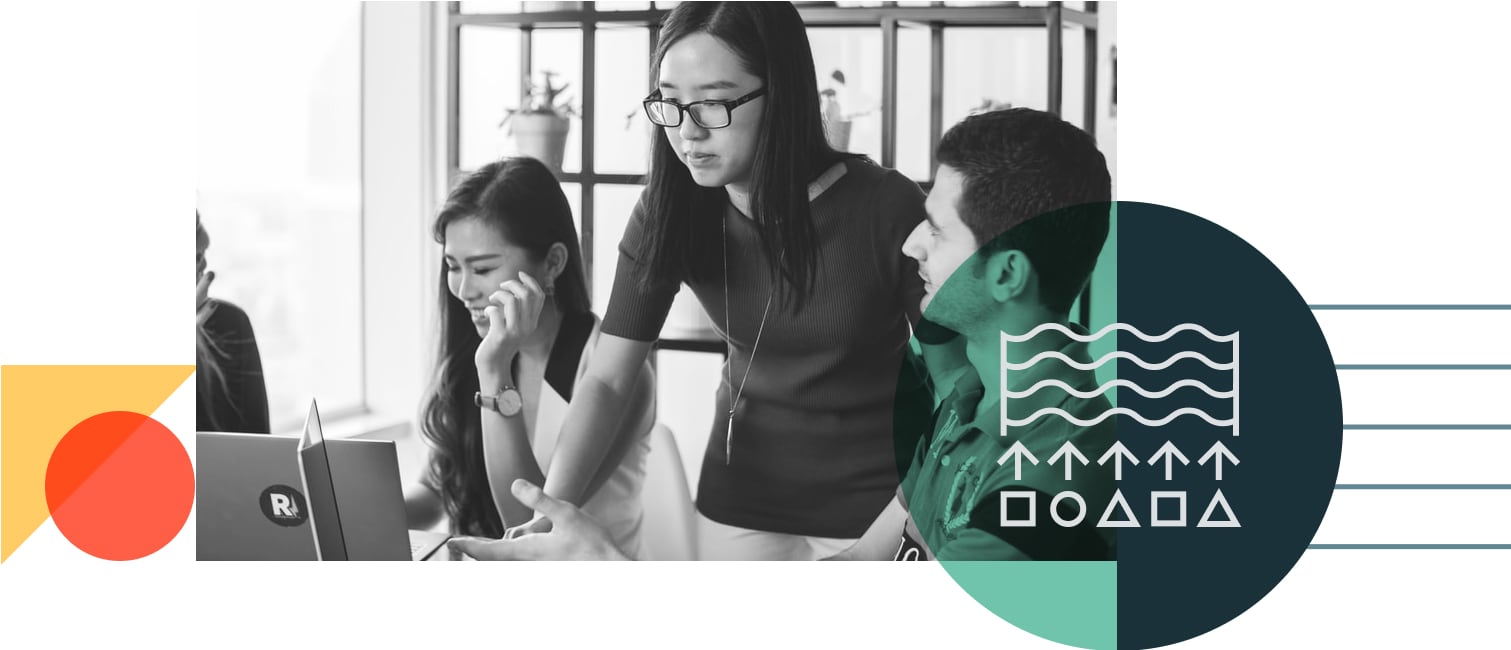
Introduction to data lakes
What is a data lake?
A data lake is a central location that holds a large amount of data in its native, raw format. Compared to a hierarchical data warehouse, which stores data in files or folders, a data lake uses a flat architecture and object storage to store the data. Object storage stores data with metadata tags and a unique identifier, which makes it easier to locate and retrieve data across regions, and improves performance. By leveraging inexpensive object storage and open formats, data lakes enable many applications to take advantage of the data.
Data lakes were developed in response to the limitations of data warehouses. While data warehouses provide businesses with highly performant and scalable analytics, they are expensive and proprietary and can't handle the modern use cases most companies are looking to address. Data lakes are often used to consolidate all of an organization’s data in a single, central location, where it can be saved “as is,” without the need to impose a schema (i.e., a formal structure for how the data is organized) up front like a data warehouse does. Data in all stages of the refinement process can be stored in a data lake: raw data can be ingested and stored right alongside an organization’s structured, tabular data sources (like database tables), as well as intermediate data tables generated in the process of refining raw data. Unlike most databases and data warehouses, data lakes can process all data types — including unstructured and semi-structured data like images, video, audio and documents — which are critical for today’s machine learning and advanced analytics use cases.
Why would you use a data lake?
First and foremost, data lakes are open format, so users avoid lock-in to a proprietary system like a data warehouse, which has become increasingly important in modern data architectures. Data lakes are also highly durable and low cost, because of their ability to scale and leverage object storage. Additionally, advanced analytics and machine learning on unstructured data are some of the most strategic priorities for enterprises today. The unique ability to ingest raw data in a variety of formats (structured, unstructured, semi-structured), along with the other benefits mentioned, makes a data lake the clear choice for data storage.
When properly architected, data lakes enable the ability to:
Data lake challenges
Despite their pros, many of the promises of data lakes have not been realized due to the lack of some critical features: no support for transactions, no enforcement of data quality or governance, and poor performance optimizations. As a result, most of the data lakes in the enterprise have become data swamps.
For these reasons, a traditional data lake on its own is not sufficient to meet the needs of businesses looking to innovate, which is why businesses often operate in complex architectures, with data siloed away in different storage systems: data warehouses, databases and other storage systems across the enterprise. Simplifying that architecture by unifying all your data in a data lake is the first step for companies that aspire to harness the power of machine learning and data analytics to win in the next decade.
How a lakehouse solves those challenges
The answer to the challenges of data lakes is the lakehouse, which adds a transactional storage layer on top. A lakehouse that uses similar data structures and data management features as those in a data warehouse but instead runs them directly on cloud data lakes. Ultimately, a lakehouse allows traditional analytics, data science and machine learning to coexist in the same system, all in an open format.
A lakehouse enables a wide range of new use cases for cross-functional enterprise-scale analytics, BI and machine learning projects that can unlock massive business value. Data analysts can harvest rich insights by querying the data lake using SQL, data scientists can join and enrich data sets to generate ML models with ever greater accuracy, data engineers can build automated ETL pipelines, and business intelligence analysts can create visual dashboards and reporting tools faster and easier than before. These use cases can all be performed on the data lake simultaneously, without lifting and shifting the data, even while new data is streaming in.
Building a lakehouse with Delta Lake
To build a successful lakehouse, organizations have turned to Delta Lake, an open format data management and governance layer that combines the best of both data lakes and data warehouses. Across industries, enterprises are leveraging Delta Lake to power collaboration by providing a reliable, single source of truth. By delivering quality, reliability, security and performance on your data lake — for both streaming and batch operations — Delta Lake eliminates data silos and makes analytics accessible across the enterprise. With Delta Lake, customers can build a cost-efficient, highly scalable lakehouse that eliminates data silos and provides self-serving analytics to end users.
Data lakes vs. data lakehouses vs. data warehouses
Data lake | Data lakehouse | Data warehouse | |
---|---|---|---|
Types of data | All types: Structured data, semi-structured data, unstructured (raw) data | All types: Structured data, semi-structured data, unstructured (raw) data | Structured data only |
Cost | $ | $ | $$$ |
Format | Open format | Open format | Closed, proprietary format |
Scalability | Scales to hold any amount of data at low cost, regardless of type | Scales to hold any amount of data at low cost, regardless of type | Scaling up becomes exponentially more expensive due to vendor costs |
Intended users | Limited: Data scientists | Unified: Data analysts, data scientists, machine learning engineers | Limited: Data analysts |
Reliability | Low quality, data swamp | High quality, reliable data | High quality, reliable data |
Ease of use | Difficult: Exploring large amounts of raw data can be difficult without tools to organize and catalog the data | Simple: Provides simplicity and structure of a data warehouse with the broader use cases of a data lake | Simple: Structure of a data warehouse enables users to quickly and easily access data for reporting and analytics |
Performance | Poor | High | High |
Learn more about common data lake challenges