What is Managed MLflow?
Managed MLflow extends the functionality of MLflow, an open source platform developed by Databricks for building better models and generative AI apps, focusing on enterprise reliability, security and scalability. The latest update to MLflow introduces innovative GenAI and LLMOps features that enhance its capability to manage and deploy large language models (LLMs). This expanded LLM support is achieved through new integrations with industry-standard LLM tools OpenAI and Hugging Face Transformers — as well as the MLflow Deployments Server. Additionally, MLflow’s integration with LLM frameworks (e.g., LangChain) enables simplified model development for creating generative AI applications for a variety of use cases, including chatbots, document summarization, text classification, sentiment analysis and beyond.
Benefits
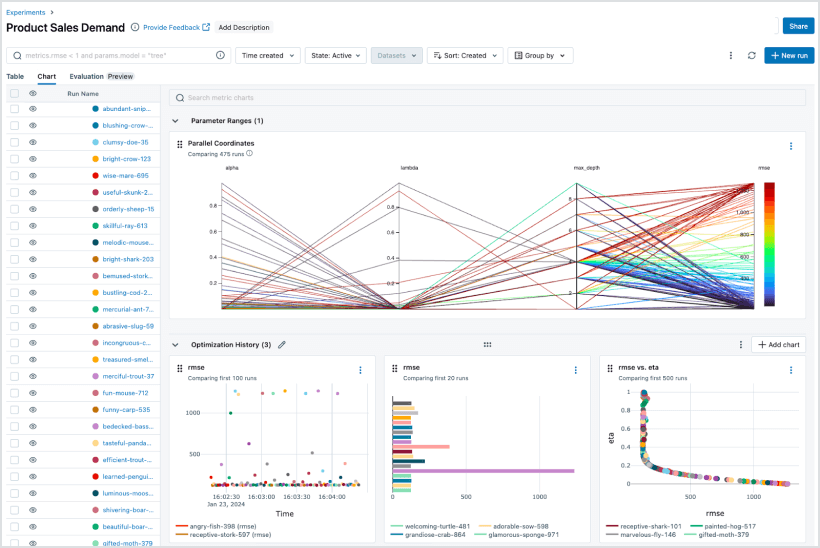
Model development
Enhance and expedite machine learning lifecycle management with a standardized framework for production-ready models. Managed MLflow Recipes enable seamless ML project bootstrapping, rapid iteration and large-scale model deployment. Craft applications like chatbots, document summarization, sentiment analysis and classification effortlessly. Easily develop generative AI apps (e.g., chatbots, doc summarization) with MLflow’s LLM offerings, which seamlessly integrate with LangChain, Hugging Face and OpenAI.
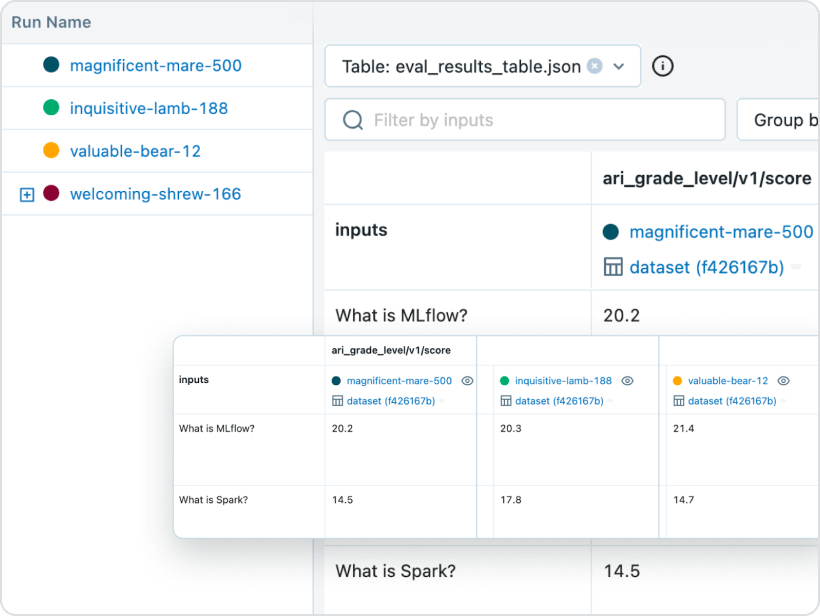
Experiment tracking
Run experiments with any ML library, framework or language, and automatically keep track of parameters, metrics, code and models from each experiment. By using MLflow on Databricks, you can securely share, manage and compare experiment results along with corresponding artifacts and code versions — thanks to built-in integrations with the Databricks workspace and notebooks. You will also be able to evaluate the results of GenAI experiments and improve quality with MLflow evaluation functionality.

Model management
Use one central place to discover and share ML models, collaborate on moving them from experimentation to online testing and production, integrate with approval and governance workflows and CI/CD pipelines, and monitor ML deployments and their performance. The MLflow Model Registry facilitates sharing of expertise and knowledge, and helps you stay in control.
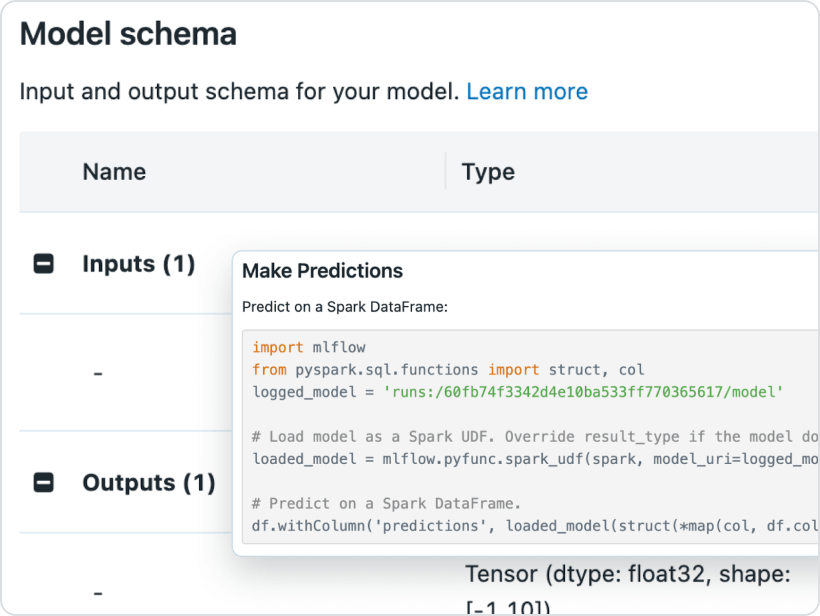
Model deployment
Quickly deploy production models for batch inference on Apache Spark™ or as REST APIs using built-in integration with Docker containers, Azure ML or Amazon SageMaker. With Managed MLflow on Databricks, you can operationalize and monitor production models using Databricks jobs scheduler and auto-managed clusters to scale based on the business needs.
The latest upgrades to MLflow seamlessly package GenAI applications for deployment. You can now deploy your chatbots and other GenAI applications such as document summarization, sentiment analysis and classification at scale, using Databricks Model Serving.
Features
See our Product News from Azure Databricks and AWS to learn more about our latest features.
Comparing MLflow offerings
Open Source MLflow | Managed MLflow on Databricks | |
---|---|---|
Experiment Tracking | ||
MLflow tracking API | ||
MLflow tracking server | Self-hosted | Fully managed |
Notebooks integration | ||
Workflows integration | ||
Reproducible Projects | ||
MLflow Projects | ||
Git and Conda integration | ||
Scalable cloud/clusters for project runs | ||
Model Management | ||
MLflow Model Registry | ||
Model versioning | ||
ACL-based stage transition | ||
CI/CD workflow integrations | ||
Flexible Deployment | ||
Built-in batch inference | ||
MLflow Models | ||
Built-in streaming analytics | ||
Security and Management | ||
High availability | ||
Automated updates | ||
Role-based access control |
How it works
MLflow is a lightweight set of APIs and user interfaces that can be used with any ML framework throughout the Machine Learning workflow. It includes four components: MLflow Tracking, MLflow Projects, MLflow Models and MLflow Model Registry
Managed MLflow on Databricks
Managed MLflow on Databricks is a fully managed version of MLflow providing practitioners with reproducibility and experiment management across Databricks Notebooks, jobs and data stores, with the reliability, security and scalability of the Databricks Data Intelligence Platform.
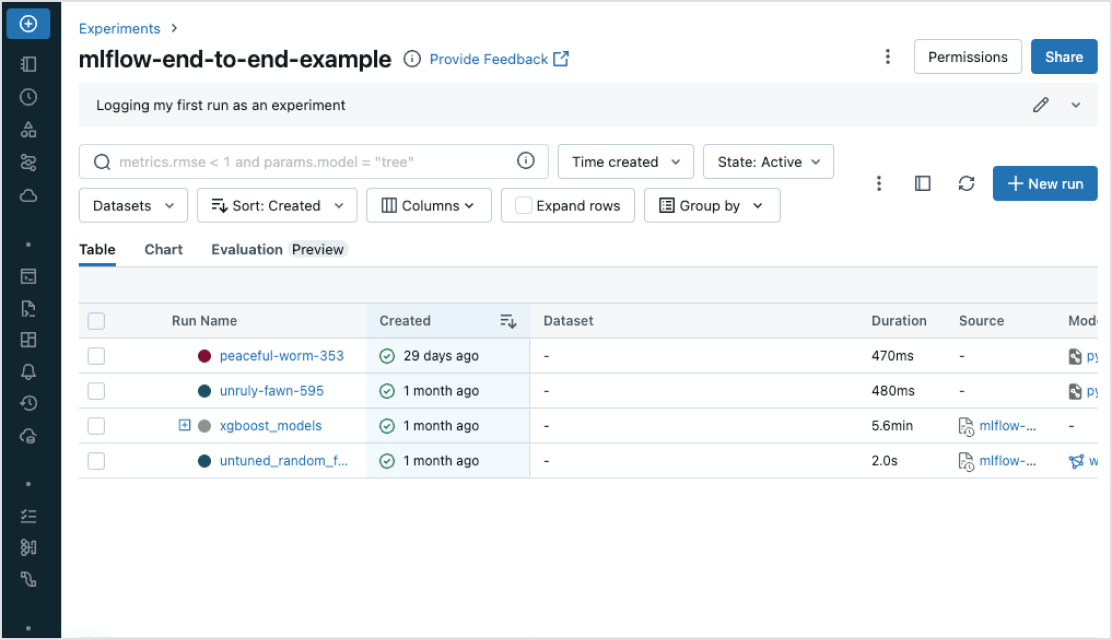
Resources
Blogs
Videos
Tutorials
Webinars
eBooks
Notebooks
Documentation
Ready to get started?