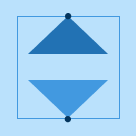
Generative AI is Everything Everywhere, All at Once
Attend Data and AI Summit on "Generation AI" either in-person or virtually to learn more.
In the fast-paced world of finance, firms are constantly seeking ways to remain competitive through increased automation, accelerated product innovation, and improved operational efficiency. Executives believe that Generative AI will play a significant role in helping Financial Services Institutions (FSI) automate, streamline and become more efficient. FSIs have started to invest in AI capabilities to analyze vast amounts of data and provide insights that augment human intelligence. For example, Bloomberg recently announced large-scale language model (LLM) 'Bloomberg-GPT,' a purpose-built 50-billion parameter LLM specifically for the financial industry, while JP Morgan reportedly used a Chat-GPT-based language AI model to analyze 25 years of Fed speeches to uncover potential trading signals.
To achieve these goals, staying at the cutting edge of deep technological advancements is essential. Equally important, however, is the ability to proactively anticipate and mitigate the risks associated with these emerging technologies.
One such technological advance that has garnered significant attention are Large Language Models (LLMs). Generative AI, which includes LLMs, possesses the remarkable ability to generate fresh and distinctive insights by discerning patterns in existing information. LLMs enable an unprecedented understanding and organization of complex data, along with the capacity to generate human-like interaction.
The introduction of LLMs presents a multitude of opportunities. Financial institutions are actively strategizing ways to unlock new possibilities for data analysis, gain deeper insights, and improve decision-making processes. By leveraging the power of LLMs, financial institutions can enhance customer experiences, refine risk management strategies, and optimize operational efficiency.
The future is bright, but are we wearing rose-colored glasses?
However, along with these promising prospects, it is crucial for the industry to remain vigilant in addressing the risks associated with LLMs. Proactively implementing robust governance frameworks, data privacy measures, and security protocols is essential to safeguard sensitive information and protect against potential vulnerabilities. A few questions every C-suite leader is asking their data teams:
- How can we customize LLMs that we own and control with our proprietary data?
- Will a single dominant LLM reign supreme?
- Will FSIs opt to develop their own models with open source tools, as was done for Dolly–an inexpensive-to-build LLM that exhibits the instruction-following capabilities of ChatGPT?
The question of whether to adopt a pre-existing model (like ChatGPT) or develop a custom solution leveraging open-source frameworks (as we did with Dolly), is a crucial strategic consideration.
Every bank, wealth manager and insurer pull from massive volumes of complex unstructured and sensitive data (transactions, market data, trades, IoT sensors, call center logs, customer profiles, customer complaints/claims, market data, risk exposure, ESG). Data generated by connected systems – much of which needs the ability to stream data in real-time and fused with important contextual data sources (which could be highly sensitive data) – needs to respond to important events in a meaningful way.
Consider this: to harness the power of this data, your people don't want more apps, more data and more browser windows, they fundamentally want to do their job better. This is where LLMs have a game-changing impact on the industry as it will radically change how people interact with systems and documents, resulting in several orders of magnitude improvement in employee productivity, customer satisfaction and financial performance.
4 areas Generative AI can drive real impact and ROI today
Below, we will explore four areas where LLMs can lower costs, improve efficiency and drive real-world impact and ROI in Financial Services:
(1) Operational Efficiency
As FSIs embrace AI, it's becoming increasingly clear that operational efficiency is a top use-case. In our recent internal review, we found that roughly 80% of initial LLM use-cases in financial services are focused on streamlining internal processes to save time and resources. One example is in the insurance industry, where insurers are leveraging LLMs to accelerate underwriting decisions by offloading manual research and review processes. Claims management is also benefiting from LLMs, which can quickly identify irregularities and key points in complex claims spanning hundreds of pages of unstructured data.
In banking, generative AI could radically reduce the programming costs and improve developers' productivity. We agree with Michael Abbott, Global Banking Lead at Accenture, when he makes the case in a recent article that the holy grail in banking could be using generative AI to reduce the cost of programming (roughly 10% of a bank's cost base today to maintain legacy core and code) while dramatically improving the speed of development, testing and documenting code. He continues to explore the possibilities of Generative AI and says, imagine if you could read the COBOL code inside of an old mainframe and quickly analyze, optimize and recompile it for a next-gen core. Use cases like this not only reduces the bank's expenses but also increases productivity of its developers to focus on higher value work.
With these advancements, the adoption of LLMs enables the financial industry to deliver improved and expedited services to customers while enhancing operational efficiency.
Ready to implement: Every model in banking or insurance is thoroughly reviewed by an independent team of experts. LLM can reverse engineer a modelling strategy and extract all necessary context used for model validation. Check out the Model Risk Management solution accelerator created jointly with global consultancy EY blog.
(2) Call Center Analytics and Personalization
In today's hyper-personalized world, conversational finance has taken center stage, allowing for more tailored services and increased efficiency. At a retail bank, the current generation of chatbots can perform simple tasks such as checking account balances. As generative AI gets embedded in the next generation of chatbots, customers will be able to get far more value-added insights to questions such as "I want to save $1000 over the next 3 months, how can I achieve my goal?" The AI will create a tailored plan for you that includes searching for a cheaper place to buy your daily coffee, suggesting subscriptions to cancel, recommending a high interest rate savings product for your savings account and automatically finding and applying discount coupons to brands where you use your debit card.
Additionally, call center staff will be able to increase customer NPS scores by learning from all previous support tickets and move into higher value work by driving revenues through higher conversion of cross-sell and up-sell opportunities.
Ready to implement: Customers are experimenting with LLMs to suggest changes in product / services across different channels based on customer complaints. Specifically, LLMs are used to summarize, categorize, and draft responses and consolidated Net Promoter Scores (NPS) to draw insights from survey data. Consider the ready to download Detect Customer Intent from IVR or Virtual Agents notebook or read the How Lakehouse powers LLM for Customer Service Analytics in Insurance blog.
(3) Risk Management and Fraud Detection
AI also helps drive more efficient risk management through faster document analysis and natural language processing. AI-powered platforms offer proactive solutions for market risk by identifying restructuring opportunities, such as notifying landlords about bankruptcies affecting their tenanted properties and alerting traders to vulnerable positions during market fluctuations.
To enhance compliance, AI enables intelligent monitoring to prevent financial crimes and fraudulent activities, minimizing false positives and negatives. To illustrate this, a recent article from Nasdaq's chair and chief executive, Adena Friedman, explains how Nasdaq uses AI for predictive market maintenance — preventing disruptions before they occur — and how AI's helps detect, deter and stop financial crime.
AI streamlines ongoing KYC (Know Your Customer) processes by finding missing data points and advising on necessary actions, leading to improved customer experience and time savings. Additionally, AI enhances fraud and AML (Anti-Money Laundering) monitoring by identifying patterns indicating fraudulent activities and monitoring real-time payment flows.
At many banks today, human-in-the-loop frameworks remain and AI augments and automates data gathering and reporting to regulators, while also identifying and recommending solutions to issues or discrepancies. With more training and security improvement, the use of LLMs may transform the compliance function, making labor-intensive functions of data collection and compliance regulations a lot more efficient.
In POC: We have a handful of European banks that are considering using Dolly to generate models to respond to the less complicated/sensitive queries from regulators' requests they receive on a daily basis. Another POC we have is with an insurer that uses LLM for "insurance contract analysis" to help summarize the hundreds of pages of insurance contracts and triaging to a specialist.
(4) Reporting and Forecasting
Finally, reporting and forecasting will be revolutionized, with AI pulling in data across more sources and automating the process of highlighting trends and generating forecasts and reporting. For example, generative AI can write formulas and queries in Excel, SQL, and BI tools to automate analysis, surface patterns, and suggest inputs for forecasts from a broader set of data with more complex scenarios, such as macroeconomics. AI can also automate reporting and the creation of text, charts, and graphs for reports, adapting them based on different examples.
Asset managers are also utilizing LLMs to diversify portfolios and extract valuable ESG insights from corporate disclosures. The International Finance Corporation (IFC), a development finance institution committed to tackling poverty in emerging markets, is harnessing data and AI to create machine learning solutions. Through their Machine Learning ESG Analyst (MALENA) platform, IFC employs NLP and entity recognition algorithms to facilitate the review of ESG issues at scale. This not only supports the work of IFC experts but also assists other investors operating in emerging markets. By capitalizing on historical datasets and open source AI solutions, IFC is democratizing access to ESG capacity, empowering users to efficiently analyze and classify text.
While AI helps asset managers make more informed investment decisions, ensuring a sustainable and responsible approach to finance, it can also help streamline procurement and payables processes by auto-generating and adapting contracts, purchase orders, invoices, and reminders. Overall, the use of AI in financial reporting and forecasting can help financial institutions more efficiently and effectively manage potential risks and stay compliant with changing regulations.
In POC: A financial institution in Asia is enabling self-service dashboards for 2,000+ analysts and users sitting in different business units across the bank to create reports using "human prompts" in order to speed up changes and creation of reports, essentially moving from text to sql.
How to get started
These examples are just some of many possible areas where LLMs can unlock value in the financial services industry. By training a model that is customized to their specific data and business requirements, organizations can achieve more tailored and impactful results.
A financial institutions approach to LLM will depend on 3 choices companies will have to make:
- Open Sourced or Closed: companies will have to decide whether to heavily leverage a proprietary third-party provider of LLMs such as OpenAI or to build their own LLM using open source tools. This decision will likely be driven by security concerns.
- Productivity or Revenue: companies will either focus on non-customer facing use cases or customer-facing ones, and that decision will be influenced by reputational and/or regulatory concerns.
- Internal or External: companies must choose to focus on LLMs that unlock the power of their proprietary datasets or rely on externally available datasets from the internet, data providers, etc. They'll likely make this decision based on compliance concerns.
Here are 6 steps to consider to take advantage of this new technology:
- Source project ideas from your entire organization. Use the chart provided in the previous section for guidance on how to classify and organize these projects. Select a few projects in each quadrant to prioritize according to the business value (for example, consider revenue versus productivity).
- Ingest data using OCR or NLP to hydrate your LLM. Combine the latest technologies in Optical Character Recognition and Natural Language Processing in order to transform any financial document, in any format (email, html, pdf, word, etc) into valuable data assets that can be used to feed your proprietary LLM. To start testing how to extract text from any document, you can sample our Digitizing Documents notebook.
- Invest in data infrastructure with built-in governance and security to support future use of generative AI. Build a data lakehouse that can collect, store, and manage all types of data (structured, semi-structured, unstructured) and supports the BI and AI use cases this technology will leverage. You might also consider ease of implementation.
- Experiment with off-the-shelf models before trying to fine-tune a model to mitigate the risk of failure. The cost of not taking any action can be high, so don't be afraid to experiment.
- Create mission-oriented teams to increase speed and focus on R&D. Embed tech implementers within the business to achieve success. Establish data ownership from Day 1.
- Bootstrap your LLM. Invest in capturing, annotating, and classifying data to make LLMs and Generative AI models powerful and relevant to your company. At the same time, document the risk implications including compliance, regulatory, reputational, and customer satisfaction.
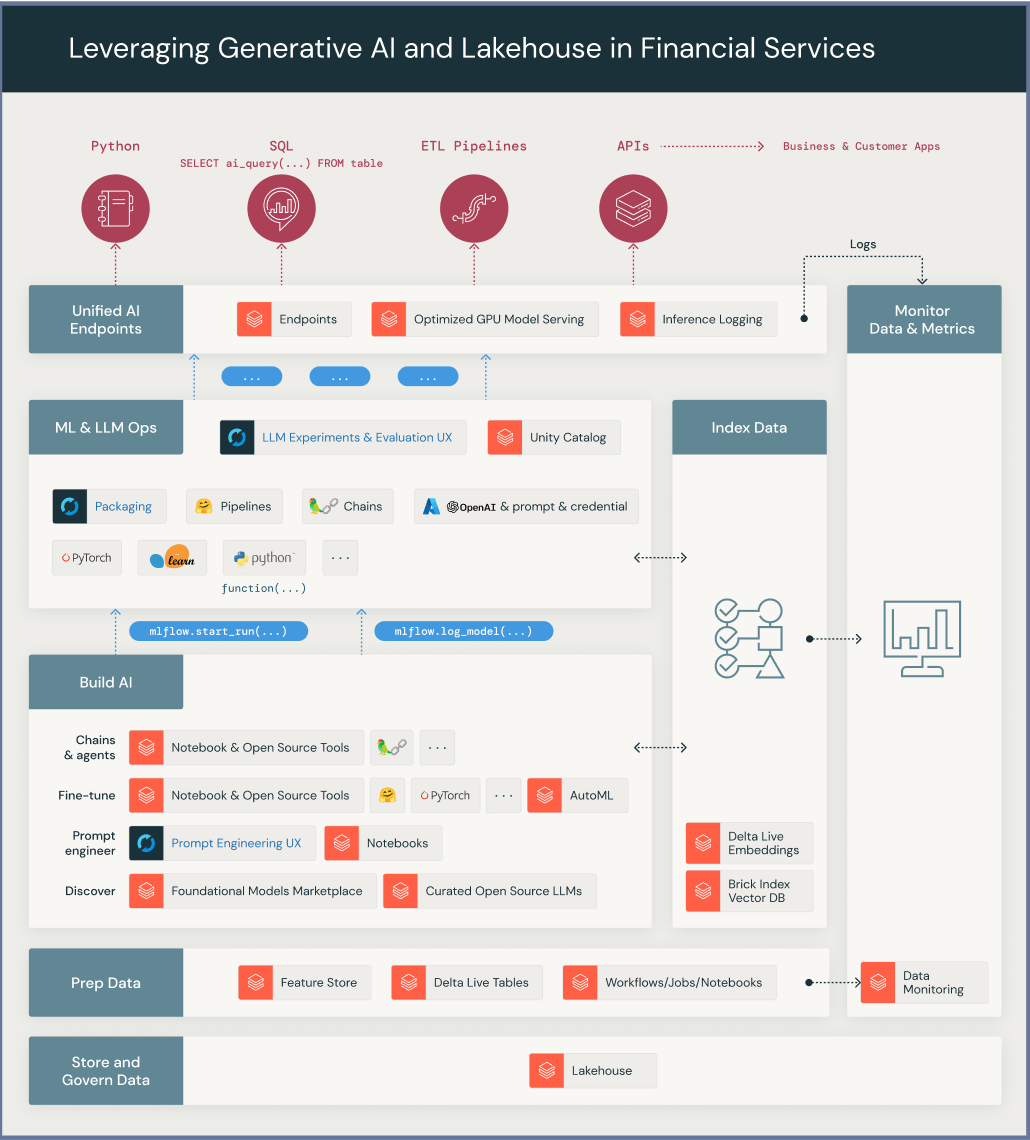
We're in the earliest days of the democratization of AI in financial services, and much work remains to be done, but we believe the Generative AI represents an exciting new opportunity for financial institutions that want to build their own instruction-following models and bring LLM projects to life for their business.
What to learn more? Attend Data and AI Summit on "Generation AI" either in-person or virtually. Visit our site to learn about the Lakehouse for Financial Services or learn how you can harness LLMs yourself in our webinar: Build Your Own Large Language Model Like Dolly.