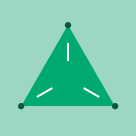
MMM (Marketing or Media Mix Modeling), is a data-driven methodology that enables companies to identify and measure the impact of their marketing campaigns across multiple channels. The purpose of MMM is to help businesses make informed decisions about their advertising and marketing strategies. By analyzing data from various channels such as TV, social media, email marketing, and more, MMM can determine which channels are contributing the most to sales and other business outcomes. By including external events and indicators, decision-makers can better understand the impact of outside factors (such as holidays, economic conditions, or weather) and avoid accidently over-valuing the impact of ad spend alone.
Using MMM, companies can identify which marketing channels are driving the most engagement, sales, or revenue. This information can be used to optimize marketing budgets and allocate resources to the channels that are most effective. For instance, let's say a company has been running marketing campaigns across different channels, such as social media, email marketing, TV ads, etc. However, the marketing team isn't sure which channel is delivering the highest ROI. This is where MMM comes in handy. By analyzing the aggregated data from all these channels, a powerful model can assist the company in identifying the most revenue-generating campaigns, as well as the channels that provide the most efficient return on ad spend, enabling them to optimize their advertising strategies effectively. This allows the business to optimize their marketing efforts and allocate their budget in the right direction.
For years, MMM has been a very powerful tool and been considered as a game-changer, giving savvy businesses the edge they need to stay ahead of the competition. By harnessing the power of data-driven decision-making, MMM empowers companies to make smart investments in their marketing strategies, ensuring that every dollar is spent in the right place, at the right time, and in the right way. This translates into compelling results, from higher customer engagement and increased sales, to a high return on investment.
The Evolution of MMM
MMM has been around for decades and has always been a powerful tool for measuring the effectiveness of marketing campaigns. The model can take into account various factors such as seasonality, competitive activity, and macroeconomic trends to provide a holistic view of the overall impact of marketing activities. However, in recent years, MMM has been somewhat overshadowed by MTA (Multi-Touch Attribution), which offers a more granular approach to attribution by tracking individual users across multiple touchpoints. With MTA, marketers can see which specific touchpoints are driving conversions for individual users and allocate budget accordingly. This level of granularity has made MTA a popular choice for many marketers, especially those in digital marketing.
But with the new privacy regulations, such as the GDPR (General Data Protection Regulation) and CCPA (California Consumer Privacy Act), the cookie-based tracking that MTA relies on is becoming more difficult. This means that MMM, which uses aggregate data rather than user-level data, is now gaining new momentum to shine.
So, you may wonder which tool to choose to measure marketing effectiveness. When choosing between the two, there are several factors to consider when it comes to selecting MMM. One option to consider is to choose MMM when some or all of your data is pre-aggregated. Another factor is whether your marketing efforts involve both online and offline channels. This is increasingly important in today's digital age, where the lines between online and offline marketing are often blurred. Finally, if you have extraneous information that is relevant to your marketing efforts, such as geo-test results, MMM can help you incorporate this data into your model.
Advantages of MMM
First, MMM based analysis can incorporate the impact of offline channels, such as TV, Print, Radio or OOH (Out-Of-Home) advertising, which are not possible to track with cookies. Some more advanced modeling techniques even have the ability to incorporate the funnel effect, which describes how various channels work together, as well as multiplicative effects accounting for the synergistic impact of these channels. Furthermore, it can provide insights into the longer-term impact of marketing activities, which MTA may miss. MMM is considered more scalable and can be used to measure the impact of marketing activities across large geographies or even entire countries.
In addition, MMM can help companies comply with privacy regulations by using aggregated data rather than individual user data. This means that companies can still measure the effectiveness of their marketing activities without compromising user privacy.
In this blog post, we will explore the key features of MMM and how the Databricks Lakehouse Platform can help businesses build a robust and scalable modern MMM solution.
Navigating Common Challenges
MMM is built by a range of companies, from consulting firms, to advertisers, and to software vendors. As businesses continue to seek ways to optimize their marketing spend, MMM has become an increasingly popular method for measuring ROI. However, building a scalable and robust MMM solution can be a challenging task. In this section we discuss some of the common challenges that companies face when building a scalable solution. In this section we discuss some of the common challenges.
One of the most significant challenges in building an MMM solution is the upstream data sources. The Data Science and Machine Learning team needs to stitch data streams from different sources for MMM data, including 3rd party data like econometrics data, which lacks standardized ways of collection. Additionally, the data sources are often scattered across various places such as legacy databases, Hive, sftp flat files, and other sources, making it challenging to obtain the necessary data. Moreover, data needs to be manually refreshed every month, which can be a tedious and time-consuming task.
Another obstacle in achieving accurate MMM results is the absence of data. For instance, it is common for econ data ingestion and traditional/offline media data to be missing, which can substantially impede the accuracy of the outcomes. This challenge can be particularly pronounced for businesses that have a presence in multiple countries, where regulations may impose restrictions on data collection and sharing. In the modern AI era, the MMM solution, being a form of machine learning model, is not exempt from the challenges and risks associated with the emerging field of AI. Tracking the lineage of data sources to the models from ingestion all the way to the insight dashboard can be a significant hurdle for traditional approaches, making it difficult to maintain transparency and accountability.
Moreover, the existence of team silos can hinder the creation of a scalable MMM solution. Model building often happens in segregated environments, leading to barriers between teams both horizontally, spanning technical and business functional domains, and vertically, across organizational levels, brands, categories, and business units. The absence of version control methods for models, code, and data can result in inconsistencies and inaccuracies within the MMM solution. Furthermore, unwieldy legacy code may make the solution challenging to maintain and update, necessitating extensive refactoring efforts. In addition, manual processes often occur, and analysis is typically performed as a one-time activity every few months, which individuals or small teams must repeat instead of being part of a more automated, repeatable, and reliable DSML pipeline.
Finally, data publishing and insight sharing are often delayed and require separate integration. This can make it challenging for stakeholders to make informed decisions quickly, leading to further delay in decision making and lost opportunities and poor agility to react in an increasingly fast-paced and dynamic economic environment - especially when competitors are moving at the speed of light. Many existing MMM solutions also lack the flexibility and privacy protection needed to collaborate effectively with customers and partners.
As a result, businesses must take a comprehensive and meticulous approach to implementing MMM in the age of AI, accounting for the unique challenges and risks associated with DSML technology.
Build your scalable and flexible MMM with the Databricks Lakehouse
Reference diagram
The Databricks Lakehouse is designed to provide a unified platform for companies to build modernized MMM solutions that are both scalable and flexible.
One of the most significant advantages of the Databricks Lakehouse is its ability to unify various upstream data sources. This means that the platform can bring together different data sources that are essential for MMM, such as the offline econometrics data, media campaign data and CRM data, and unify them into a single source of truth. This is particularly useful in today's data-driven world, where companies have to deal with large volumes of data from different sources.
Another key advantage of the Databricks Lakehouse can greatly benefit MMM is the ability to streamline the data pipelines. Post data ingestion, MMM process requires the transformation of various marketing channels and analyzing their impact on KPIs, which involves handling a wide range array of data from various sources. The Lakehouse can automate data ingestion, processing, and transformation, reducing the time and effort required to manage data pipelines manually. This ensures that data is delivered incrementally, with comprehensive data quality monitoring.
What's more, the Lakehouse provides a lineage tracking system that ensures to establish the lineage for all your data assets - not just data itself but also include the code, model artifacts and job iterations - is a significant benefit of the Databricks Lakehouse. It provides full transparency and traceability of data usage, enabling businesses to confidently make data-driven decisions. This is especially important in the context of MMM, where the accuracy and reliability of data are critical for understanding the impact of marketing channels on sales and shifting the marketing dollar allocation.
Moving to the modeling phase, one of the key differentiators of the Databricks Lakehouse is its powerful DSML capabilities, which are particularly evident in its first-class ML Runtime and MLOps tools. One crucial task for MMM modeling is the extensive prior setting and variables transformation, which require a large number of iterations. MLflow enables marketers to track the derivation and transformation of their independent variables (features) and their usage in models. Additionally, the Databricks Feature Store encourages best practices in feature engineering, providing the DSML team with the tools and infrastructure necessary to create, discover, and reuse features. This streamlines the modeling process and enhances the accuracy of predictions for business outcomes. These capabilities enable marketers to seamlessly leverage the full potential of their data, driving more informed and effective marketing decisions.
By now, it should be evident that Databricks brings tremendous efficiency gains to the MMM team! With Databricks, even single-node data scientists can distribute tuning and training, run multiple scenarios and configurations simultaneously across the cluster, and build independent models across brands, categories, and geographies in parallel, see demonstration below:
The ML Runtime is a fully managed, secured, and collaborative ML environment that directly leverages the DS team's productivity without requiring them to bootstrap, build, or maintain their own DS environment. Moreover, it fosters a collaborative workstream by facilitating easy sharing of work, which prevents inconsistent approaches adopted by different teams. One solution is to develop mechanisms for populating missing data, such as sourcing from a marketplace or storing data procured from sources like Dun & Bradstreet, S&P, Edgar, weather data, and market research in a well-curated location in the Lakehouse. This approach can prevent teams from reinventing the wheel when it comes to data and code, ultimately saving time and resources. However, it is vital to acknowledge that this lack of reuse and reinventing the wheel also applies to code and the rest of the pipeline, making it necessary to move MMM to team silos to increase efficiency and minimize discrepancies.
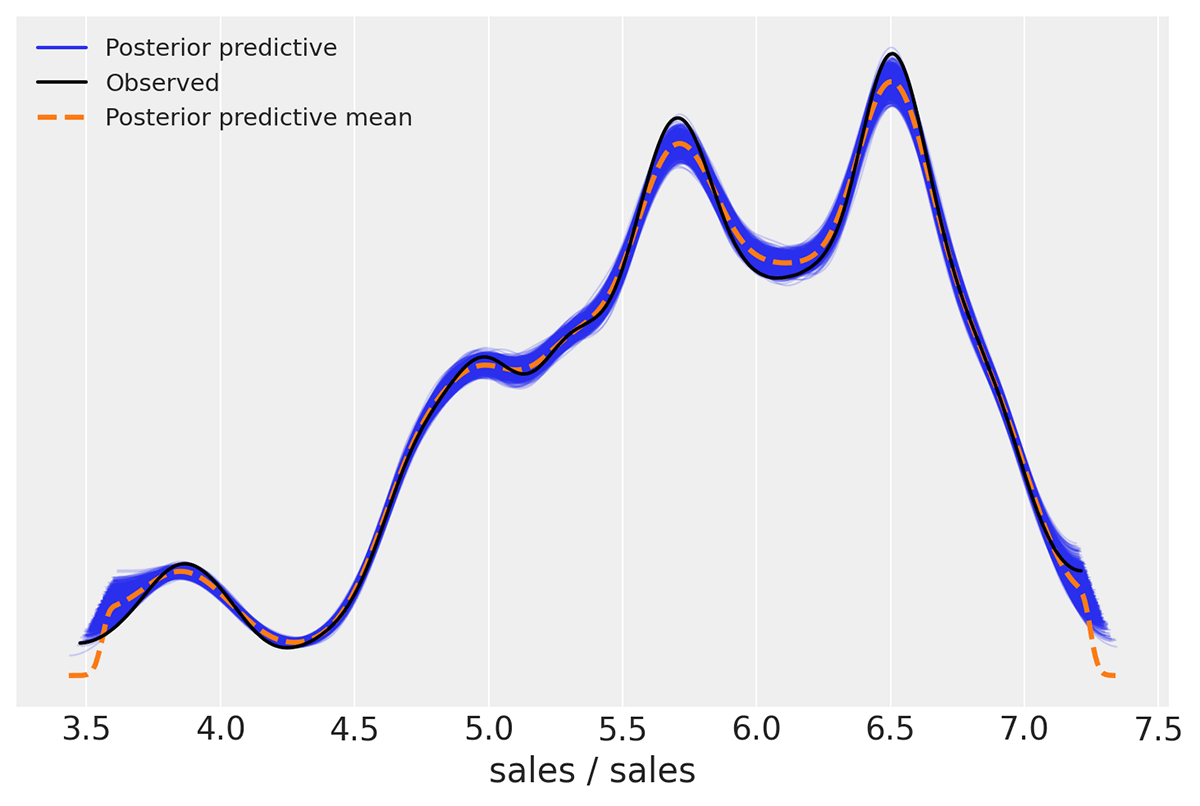
The open-source nature of Lakehouse provides the ideal environment for running all popular open-source libraries for MMM, such as PyMC in Python and Robyn in R. This feature empowers users to build solutions that cater to their specific needs and avoid vendor lock-in.
Last but not the least, DBSQL with BI integration and Databricks marketplace allows the MMM team to publish the model insights with ease, shortening the time to bring new modeling projects from data collection to MMM Executive actionable insights. By consolidating and standardizing all data and AI activity, the Lakehouse lends itself the best place to build not only an MMM solution but also any other data and AI solution the team will work on today and tomorrow.
Learn how Databricks for Lakehouse can help you optimize marketing campaigns across multiple channels with MMM. Access the solution accelerator.