Solving global trade challenges across the supply chain
Altana relies on Databricks Mosaic AI to rapidly deploy cutting-edge GenAI products
faster deployment of GenAI models into production
better model performance
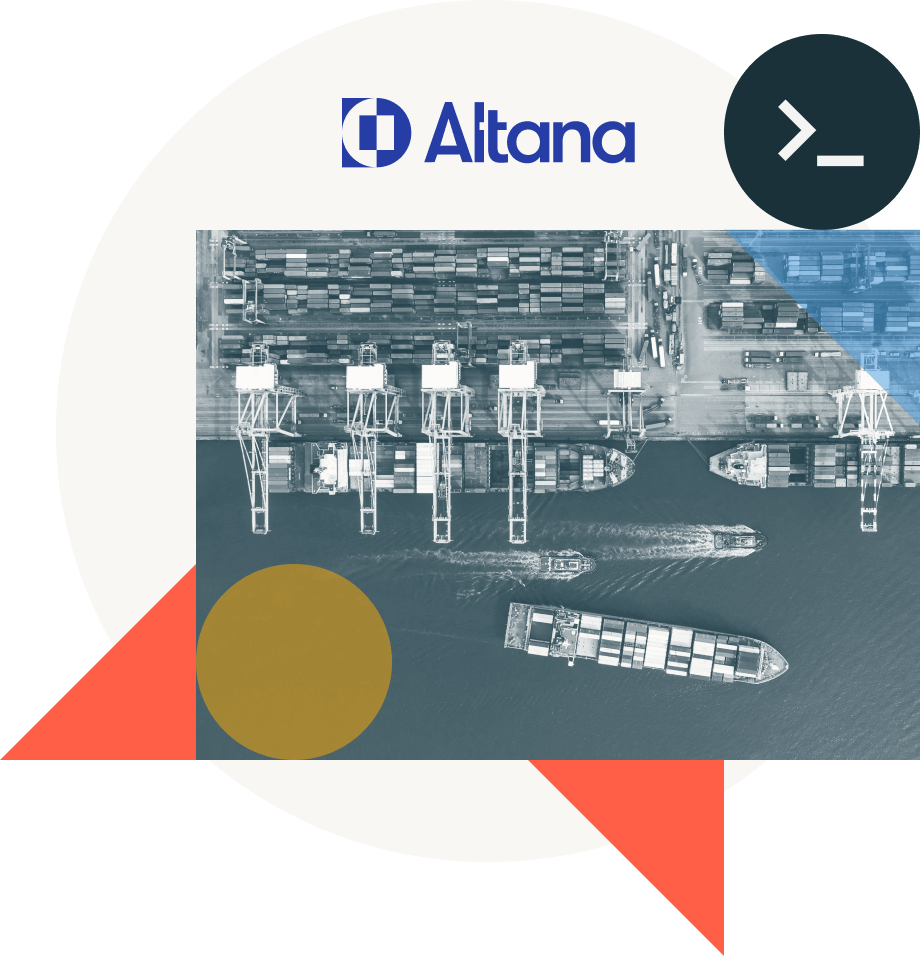
Altana, the world’s first value chain management system, is on a mission to build a more transparent and reliable global supply chain. Altana needs to create many disparate models and manage an enormous amount of data for various customer use cases. Databricks has facilitated managing and optimizing their data and machine learning (ML) workflows, to rapidly iterate and fine-tune models for diverse customer needs. Prior to Databricks, the company had to create boilerplate tools for launching GenAI applications, diverting focus from cutting-edge product functionality. After leveraging Databricks Mosaic AI for their generative AI product development, Altana could streamline their ML lifecycle, enhance collaboration across teams and improve operational efficiency. This transition allowed them to deploy models 20 times faster and achieve up to 50% better performance, enabling customer-centric innovations that were previously unattainable.
Vendor lock-in and workflow inefficiencies hindered Altana’s global trade vision
Altana is a growth-stage product company that is building a global network around supply chains where commerce is transparent and reliable. As Ian Cadieu, CTO of Altana, explained, “We’re focused on building a safer and more prosperous world — where commerce is based on trust and trust is built on clarity; where customers and suppliers, public and private, have a common view of global trade; and where business and government work together to make global commerce more resilient, more secure and more sustainable.” The company operates in multiple countries on three continents, serving over a thousand customers with a team of nearly 200 employees.
Altana’s business is deeply rooted in data and ML, which are core to their product offerings. They cater to a diverse array of customers with varied cloud demands, requiring a comprehensive platform optimized for rapid development and deployment. Their advanced workflows integrate custom deep learning models and fine-tuned agent workflows to provide robust solutions for global trade challenges.
Altana uses generative AI in several innovative ways. One use case involves streamlining cross-border trading needs by generating accurate tax and tariff classifications for goods, as well as producing comprehensive legal write-ups. “These models generate what is the right tax and tariff classification of this good and then auto-generates an entire legal write-up that a customer can use for justification purposes,” Cadieu described. Another use case focuses on enhancing their global supply chain knowledge graph by integrating customer data in a privacy-preserving manner, thereby enriching the understanding of supply chain dependencies and enabling strategic decision-making.
Before partnering with Databricks, Altana faced significant challenges in managing and optimizing their data and ML workflows. “We deploy a large number of different models that combine into a few different workflows for customers. We needed to rapidly iterate on approaches, fine-tune the data and models for customers and measure R&D as well as live performance across several cloud deployments,” Cadieu explained. The company’s existing infrastructure forced them to spend valuable resources building boilerplate infrastructure and evaluation tools instead of focusing on product functionality.
Cadieu also highlighted the difficulties of balancing product functionality, total cost of ownership, vendor lock-in and information security priorities. “We were facing tension between all these dimensions, but with Databricks’ open architecture, it allowed us to strike the right balance and be in control when we needed to swap in specific models, regional deployments, or optimize specific components as needed.” This balance was crucial for Altana to streamline their operations and enhance their product development velocity.
Rapidly train, test and deploy ML workflows with Databricks Mosaic AI
Altana leveraged the Databricks Data Intelligence Platform to optimize their ML and data workflows and deliver GenAI products more quickly. Their most advanced workflows use a combination of custom deep learning models for the early phases, followed by fine-tuned agent workflows with retrieval augmented generation (RAG) for later refinements. Then, reinforcement learning from human feedback (RLHF) from customers is used for further model refinement. As Cadieu detailed, “We’re using Databricks to keep track of all the user input/output decisions so that we can identify which steps and models in the workflow need fine-tuning for cost, performance or latency reasons.”
Cadieu’s team took a compound AI systems approach, combining existing large language models (LLMs) like DBRX, Llama 3 and Phi-3 with their own internal proprietary datasets for their RAG workflows. These open source models were also used as the backbone for some of their deep learning solutions. Databricks Mosaic AI tools like Managed MLflow and Model Serving were pivotal in managing their extensive ML lifecycle. “The ‘batteries included’ nature of Databricks allows us to do it all in one place and collaborate across our data ingest, data engineering, ML and software teams,” Cadieu added. “A key improvement for us was the model and LLM evaluation tools in MLflow, combined with Model Serving. These have allowed our ML teams to rapidly train, test and deploy their own models for customers.”
One of the standout features for Cadieu was Delta Lake, which offered real-time data ingestion and robust data management capabilities. “We migrated everything to the Delta format about a year ago. The time travel feature has been very helpful in complex debugging of data workflows,” Cadieu explained. Additionally, Delta Lake’s shallow copies for experimentation and AI-driven performance optimizations were crucial in managing the total cost of ownership as the company scaled.
Databricks Unity Catalog provided the team with the necessary tools for data governance, ensuring customer data privacy and security. “We have an extensive focus on data management and governance with our federated architecture and privacy-preserving approach. All customer data is kept separate and private,” Cadieu said. “Unity Catalog has given us exactly what we need to govern all this information properly while maintaining client confidentiality.”
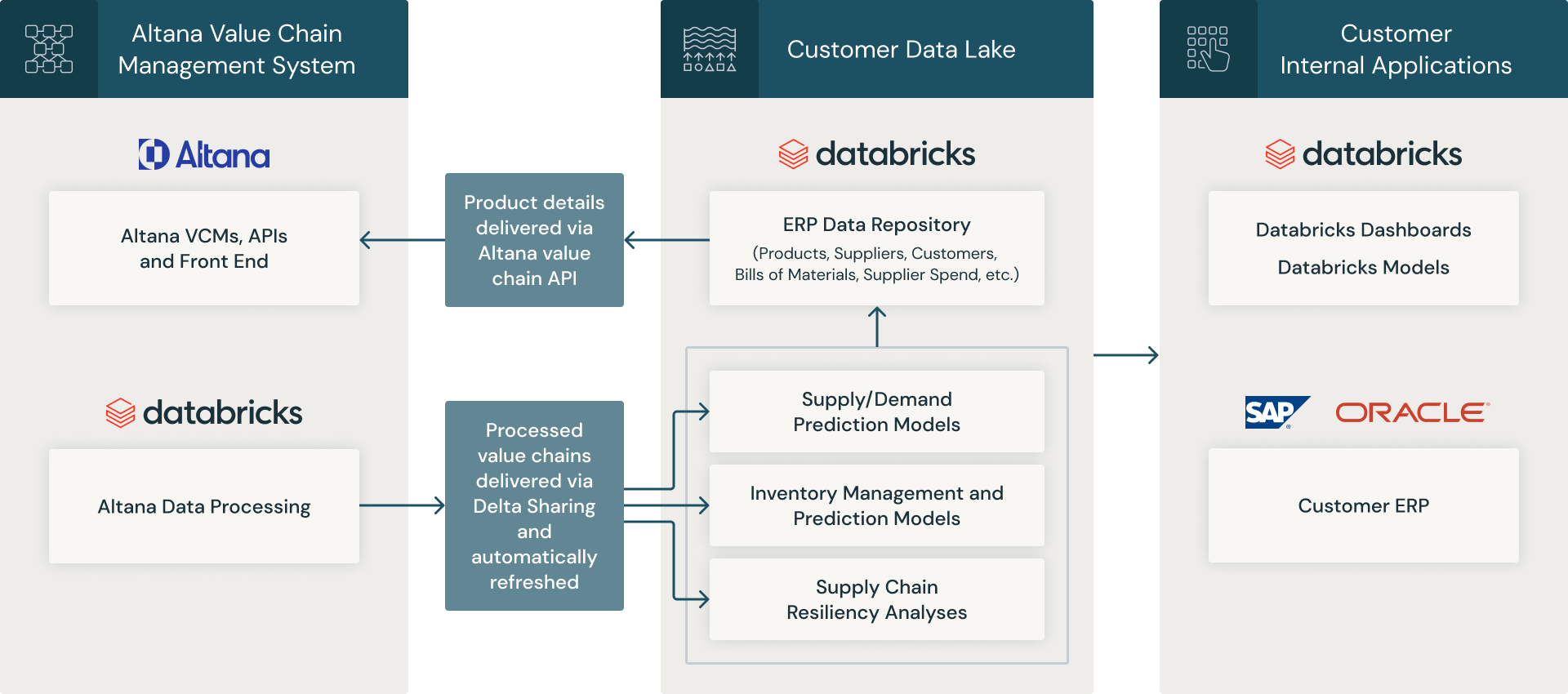
Deploying GenAI models 20x faster with up to 50% improved performance
By integrating and normalizing diverse data sources and serving ML models on a unified platform, Altana achieved a significant boost in operational efficiency and product development speed. As Cadieu summarized, “The end-to-end solution of data models, hosting and performance management — all supported by top-notch tooling — has allowed us to focus on building products for our customers as opposed to the ritual infrastructure.”
“The platform has helped us rapidly accelerate product delivery and time to value for our customers,” he continued. “We have a data-intensive business that requires integrating, enriching and normalizing data from a variety of sources and unifying with customer data before serving ML models and products. Databricks’ integrated approach has been a huge unlock for us.” Since integrating end-to-end solutions that combine systems of models, deep learning and RAG/fine-tuning workflows, Cadieu indicated that their model performance has improved by 20–50% — ”even for our most demanding workloads.” This allows Altana to ship a truly AI-driven workflow for their users. “Since migrating our GenAI development workflows to Databricks, we’ve expanded our model catalog. We’re training and deploying models more than 20 times faster to production,” Cadieu emphasized.
And this is just the beginning. Cadieu and the rest of the Altana team firmly believe that AI and ML workflows should be integrated more deeply across their product surface area. “We’re already integrating agentic workflows in our ETL process for enrichment, validation and normalization. We’ll be expanding the usage further,” Cadieu said. “Databricks is a core part of that as we can build across our org from a common operating environment.”
To learn more about how Altana helps customers understand supplier networks and value chains through AI-powered insights, visit: altana.ai.